- Problem Description
- Chapter 1: Introduction
- 1.0 Introduction
- 1.1 Background
- 1.2 Statement of the Problem
- 1.3 Objectives of the Study
- 1.4 Research Questions
- 1.5 Research Hypothesis
- 1.6 Theoretical Perspective of Contract Farming
- 1.7 Significance of the Study
- 1.8 Scope of the Study
- 1.9 Operational Definition of Terms as Used in the Study
- Table 1: The Study Variables
- 1.11 Relevance to the Body of Research
- Chapter 2: Literature Review
- 2.1 Introduction
- 2.2 Contract Farming
- 2.3 Contract Farming in Uganda
- 2.4 Factors Responsible for CF Participation
- 2.5 Impact of CF Participation on Household Income of Smallholder Farmers
- 2.6 CF Implementation Frameworks and Smallholder Farmers
- Chapter 3: Research Methodology
- 3.1 Introduction
- 3.2 Research Design
- Study Area
- 3.4 Population and Time Frame
- 3.5 Sampling and Sample Size Determination
- 3.6 Inclusion and Exclusion Criteria
- 3.7 Data Collection Instruments
- 3.8 Data Collection and Analysis
- 3.9 Impact Evaluation Method
- 3.11 Limitations of the Study and Alternative Strategies
- 3.12 Conclusion
- CHAPTER 4: DATA ANALYSIS AND INTERPRETATION OF RESULTS
- 4.0 Introduction
- 4.1 Descriptive Statistics
- 4.2 Characteristics of the Study Population
- Table 2: Continuous Variables
- Table 3: Categorical Variables
- CHAPTER FOUR ESTIMATION, PRESENTATION, AND INTERPRETATION OF RESULTS
- Table 3.1: Descriptive Statistics of Continuous Variables (Overall Sample)
- 4.3 Pre-Estimation Tests
- 4.4 Post-Estimation Test Results
- 4.5 Econometric Results
- Conclusion
- Policy Implications and Recommendations
- Limitations of this Study and Areas where Further Research is necessary
Smallholder participation in tobacco contract farming is a critical aspect of the agricultural landscape, impacting both farmers' livelihoods and the tobacco industry. This in-depth research study delves into the complex factors driving farmers' decisions to engage in contract farming, particularly in the context of a Data Analysis assignment. Through meticulous data analysis and econometric modeling, the study unveils key drivers like age, education, and farming experience, shedding light on the impact of these factors on smallholder participation. These findings are crucial for stakeholders in the tobacco sector and policymakers aiming to enhance the sustainability and productivity of smallholder tobacco farming.
Problem Description
Agriculture in Uganda relies heavily on smallholder farmers, who contribute 80% of the country's agricultural output. However, these farmers face challenges like limited access to credit and unreliable produce delivery. In response, agribusinesses have turned to contract farming (CF) to work with these farmers, formalizing agreements to ensure a consistent supply of agricultural products. While CF promises to benefit both farmers and agribusinesses, there is ongoing debate about its true impact on household income among smallholder farmers in Uganda.
Chapter 1: Introduction
1.0 Introduction
In Uganda, the government has adopted agro-industrialization as a strategy to foster integration between farmers and agro-industries. This approach aims to boost private sector investments in agriculture, provide support to smallholder farmers, and ultimately reduce poverty and income inequality.
1.1 Background
Smallholder farmers play a significant role in Uganda's agricultural sector. However, their risky nature makes it challenging for them to access credit or gain the trust of other value chain actors. Agribusinesses have turned to contract farming to overcome these challenges by formalizing agreements with smallholder farmers.
The cotton sector in Uganda is a prime example where contract farming has been implemented, helping ginners and merchants provide support and technology to farmers. Despite its potential benefits, CF has faced criticism for exploiting farmers and limiting their access to competitive resources.
1.2 Statement of the Problem
While CF has gained considerable attention in Uganda and other developing countries for its potential to enhance farmers' income, the extent of its impact in Uganda remains unclear. Some studies suggest a positive impact on income, while others argue the opposite. This study aims to clarify the impact of CF on household income among smallholder farmers in Uganda.
1.3 Objectives of the Study
General Objective
This study seeks to examine the impact of CF on the household income of rural smallholder farmers in Uganda. It particularly focuses on assessing the impact of smallholder farmers' participation in the agri-commodity value chain through CF on their income and livelihoods.
Specific Objectives
The specific objectives of this study include:
- Identifying the main factors influencing CF participation among farmers.
- Assessing the impact of these factors on smallholder farmers' decisions to participate in CF.
- Evaluating the impact of CF on household income among smallholder farmers.
1.4 Research Questions
To achieve the objectives, this study will answer the following research questions:
- What are the main factors influencing farmers' participation in CF?
- To what extent do these factors influence CF participation among smallholder farmers?
- Does CF participation have a significant impact on income levels among smallholder farmers?
1.5 Research Hypothesis
The research literature shows controversy and gaps in understanding the impact of CF on household income among farmers. This study aims to answer the main research question: Does CF participation impact the household income of smallholder farmers?
To answer this, the following hypotheses were formulated:
- Socio-economic and demographic factors significantly influence CF participation among smallholder farmers.
- There is a positive correlation between CF participation and household income levels among farmers.
1.6 Theoretical Perspective of Contract Farming
Existing research on contract farming primarily centers on its typology based on transaction costs, asset specificity, and risk transfer. Studies addressing the impact of contract farming emphasize its role in mitigating market and production risks. They argue that CF reduces transaction costs, aids small producers in overcoming market challenges, and offers firms a stable supply, optimizing their operations while reducing investment costs and risks (Eaton & Shepherd, 2001; Knoeber & Thurman, 1995).
The theoretical foundation of this study is grounded in two main theories: the life cycle theory and the transaction cost theory. The life cycle theory, derived from Adam Smith's Theory of labor limitation, suggests that contracting initially consolidates vertically in early-stage industries but diminishes as the industry matures due to product differentiation and traceability (Rehber, 2007). Conversely, the transaction cost theory posits that firms exist to reduce transaction costs in exchanges, especially when it is more cost-effective to produce an input instead of purchasing it. This theory emphasizes the role of smallholder farmers' socio-economic factors in shaping their perceptions of costs and risks when deciding to engage in CF (Mugwagwa et al., 2020; Hobbs & Young, 1999; Key & Runsten, 1999).
1.7 Significance of the Study
This study aims to provide clarity on the conflicting research findings regarding the impact of contract farming on household income among farmers in Uganda. It has both policy and practical relevance. The study's results can guide policymakers in expanding contract farming, offering benefits to smallholder farmers, and combating poverty and income inequality. Additionally, the findings will serve as a valuable reference for future researchers exploring this topic in different contexts.
1.8 Scope of the Study
This study focuses on assessing the impact of contract farming on household income among smallholder farmers in rural areas of Uganda. The study will be conducted in the cotton-growing District of Pakwach in the West Nile sub-region of Northern Uganda. Data will be collected from 384 smallholder cotton farmers, both contracted and independent, from December 2021 to February 2022 to evaluate the impact of CF on household income.
1.9 Operational Definition of Terms as Used in the Study
This section provides operational definitions of terms used in the study to clarify and standardize research data. The study explores the impact of contract farming (CF) on household income among smallholder farmers. Key definitions include:
Contract Farming (CF)
CF is considered the independent variable in this study, denoting farmers' participation in CF. It is measured using a dummy variable where 0 indicates nonparticipation, and 1 indicates participation in CF. While CF is an independent variable, it also serves as an explanatory variable that predicts or explains differences in the response variable. Explanatory factors for CF participation include demographic characteristics and economic factors.
Household Income
Household income is defined as the total income of all members of a household over a certain age. For this study, household income is the annual income of smallholder farmers in the year 2021 and is measured in Ugandan shillings (UGX).
Smallholder Farmers
Smallholder farmers, as defined by the Uganda Bureau of Statistics, are those cultivating less than an acre of land, engaging in labor-intensive agriculture, primarily using family labor and rudimentary technology. They produce mainly for family consumption with limited surplus for sale (UBOS, 2020). The Uganda Cotton Development Organization defines smallholder cotton farmers as those with an average farm size of 0.5 hectares (Aanyu et al., 2020).
Study Variables
In this study, the primary independent variable is the participation of smallholder farmers in contract farming (CF), indicated as 1 if adapted and 0 otherwise. Additionally, several independent or explanatory variables encompass demographic and socio-economic factors characterizing smallholder farming households. These variables include:
- Age of household head
- Gender of household head
- Marital status
- Education level
- Household size
- Cotton farming experience
- Farm size
- Risk aversion
- Crop yield
- Market price
- Access to credit
- Access to extension services
- Membership in a farmers' group or association
The dependent variable is the household income of smallholder farmers. Definitions, measurements, and units for these variables are detailed in Table 1.10.1.
Table 1: The Study Variables
Variable | Definition | Value and Unit of Measurement |
---|---|---|
Dependent Variables | ||
Household Income | Average annual Household income of smallholder farmers (2020) | Continuous variable (UGX) |
Independent Variables | ||
Contract farming (CF) | Participation in CF | Dummy, 0 = nonparticipation in CF, 1 = participation in CF |
Socio-Economic Characteristics of Farmers | ||
Age | Age of the Household Head | Continuous variable (years) |
Gender | Gender of the household head | Dummy variable, 0 = Female, 1 = Male |
Household size | Number of family members | Continuous variable in numbers |
Marital status | Marital status of the household head | Dummy variable, 1 = married, 0 = not married |
Level of Education | Education level of the household head | Years of schooling |
Experience in Farming | Number of years of farming by household | Continuous variable (years) |
Farm size | Size of farmland cultivated by the household | Continuous variable (in acres) |
Risk aversion | The level of risk aversion to production and market risks | Dummy variable, 1 = High, 0 = Otherwise |
Crop yield | Cotton crop yield per acre | Continuous variable (UGX) |
Market price | Seed cotton price per kilogram | Continuous variable (UGX) |
Credit Facilities | Access to credit services | Dummy variable, 1 = access to credit, 0 = Otherwise |
Market Access | Time taken to find a market for produce without CF | Continuous variable (minutes) |
Extension services | Access to agriculture extension services | Dummy variable, 1 = access to extension services, 0 = Otherwise |
Membership to farmer group | Belongs to a farmer’s group | Dummy variable, 0 = No, 1 = Yes |
Household Income | Income from cotton farming (Y) | Continuous variable (UGX) |
(UGX is Ugandan Shillings, with an exchange rate of $1 = 3,600 UGX)
1.11 Relevance to the Body of Research
The impact of contract farming on household income among farmers in developing countries has remained a contentious subject in scholarly and rural development discourse since the 1970s. Existing research evidence from the developing world has produced varied outcomes regarding the effect of contract farming on farmers' income. Some studies suggest a positive impact, while others find a negative effect. Furthermore, certain studies conclude that there is no evidence of smallholder farmers' exclusion from CF affecting their income.
Therefore, this study seeks to contribute to the existing body of knowledge by elucidating the conflicting findings surrounding the impact of CF on household income among farmers in developing countries. It aims to do so through extensive and innovative research conducted among smallholder farmers in the rural areas of Northern Uganda. This research intends to provide a clearer perspective on the implications of contract farming and its role in shaping the income of smallholder farmers.
Chapter 2: Literature Review
2.1 Introduction
This chapter provides an in-depth review of previous research studies related to contract farming (CF) and its influence on the household income of farmers. The literature is reviewed to gain a comprehensive understanding of this research area. Database searches were conducted using Google Scholar with specific keywords and Boolean logic to select relevant, recent, and peer-reviewed articles. Scopus was also employed to validate sources and ensure that non-indexed sources were excluded.
2.2 Contract Farming
Contract farming, defined as an agreement between farmers and other actors in the supply chain, has become a powerful tool in transforming subsistence farming into commercial agriculture in developing countries (Meemken & Bellemare, 2020). There are various models of CF that vary based on the nature of the contractor, product, vertical coordination intensity, and stakeholders involved. These models include centralized CF, nucleus estate model, multi-stakeholder model, and informal agreements. CF is considered a popular avenue for agricultural value chain integration and vertical coordination (Rehber, 2007).
The life cycle theory, rooted in Adam Smith's Theory of labor limitation by market extent, suggests that contracting is a means of consolidation in early industry stages, and it diminishes in mature stages due to product differentiation and traceability (Rehber, 2007). As firms grow, specialization becomes a basis for profitability, reducing the need for integration. In the context of CF, integration is common in emerging markets or developing economies, where these conditions are met, as seen in the cotton sector in Uganda.
Vertical coordination in CF, referred to as vertical integration, provides a win-win mechanism for collaboration between firms and producers (Rehber, 2004). However, the economic viability of contracts depends on factors such as product quality, perishability, technical difficulty in production, high-quality products, conducive environments, and market willingness to pay premiums. In developing countries, high transaction costs contribute to market imperfections and increase the risk of market failures (Pingali et al., 2005). CF becomes a practical alternative due to transaction costs like supervision, land acquisition, training, and crop risks. Thus, CF is essential in reducing transaction costs, which must be lower than alternative arrangements for CF to be feasible (Eaton & Shepherd, 2001).
2.3 Contract Farming in Uganda
In Uganda, CF is practiced across various subsectors and plays a significant role in agricultural value chains. This practice has expanded to include crops such as seed oil crops, cotton, sorghum, barley, maize, and apiculture, largely driven by farmer groups and cooperatives seeking to mitigate market risks and production constraints (Akumu et al., 2020).
Smallholder farmers in Uganda are considered risky for agribusiness firms, making them reliant on backward linkages. They account for over 80% of Uganda's agricultural production. However, these farmers have been known to break agreements by selling produce on the open market, leading to loss for supporting agribusiness firms (Nalukenge, 2005). CF has the potential to provide a viable alternative mechanism for enhancing productivity, market access, quality control, and safety among farmers in Uganda (Aanyu et al., 2020).
2.4 Factors Responsible for CF Participation
The factors driving CF adoption in developing countries are varied and include advocacy and demonstration effects from development agencies and NGOs. These organizations aim to improve farmers' gains from agriculture to enhance rural livelihoods and reduce poverty and income inequality (Minot & Sawyer, 2016). Empirical evidence on the factors leading to the increasing popularity of CF in Uganda is limited. However, local institutions, socioeconomic factors, and environmental variables impact the adaption strategies employed by farmers.
2.5 Impact of CF Participation on Household Income of Smallholder Farmers
There is a lack of specific studies examining the impact of CF on farmers' incomes in Uganda. Some studies conducted in Uganda have provided conflicting findings regarding the effect of CF on farmers. While some studies indicate positive outcomes in terms of market deepening, productivity, and income, others have found that CF negatively affects farmers' income, welfare, and the environment. The impact of CF on household income among smallholder farmers in Uganda remains unclear.
The impact of CF on farmers' income varies across different developing countries. Some studies have reported positive outcomes, while others found negative effects or no impact on income. This variation indicates the need for further research to clarify the impact of CF on smallholder farmers' incomes, particularly in the context of individual countries (Alemu et al., 2016; Briones, 2015; Mwambi et al., 2016).
2.6 CF Implementation Frameworks and Smallholder Farmers
In developing countries, smallholder farmers often face production and market challenges, which CF is seen as a solution for mitigating. However, the governance structure of CF typically favors agribusiness firms, resulting in exploitative arrangements for smallholder farmers. While CF empowers smallholder farming communities and enhances the commercial viability of agriculture, the institutional arrangements tend to be unequal, benefiting agribusiness firms more (Kumar et al., 2018; Martiniello, 2021).
Despite the challenges, CF has been shown to empower youth and women, increase business acumen among smallholder farmers, improve market access, and enhance household income. The researcher intends to develop a CF framework and recommend institutional arrangements to address these challenges and empower smallholder farmers.
Chapter 3: Research Methodology
3.1 Introduction
Research methodology is the blueprint guiding the identification, selection, processing, and analysis of information concerning a research subject (Goddard & Melville, 2004). This chapter provides insight into the approach, procedures, and methods employed to investigate the impact of contract farming (CF) participation on household incomes among smallholder cotton farmers in Northern Uganda, focusing on the case of smallholder cotton farmers in Pakwach District. It describes the data sources, collection methods, and analysis procedures used, alongside justifications and other essential components of the study design.
3.2 Research Design
Research design, as articulated by Bell et al. (2018), pertains to the strategy that guides the selection of research methods. In this study, a causal approach was employed with a mixed qualitative and quantitative research design. The decision to use a mixed design was based on the need to address research questions effectively and the inadequacy of existing knowledge regarding the study phenomenon in the Ugandan context. The mixed design offered methodological flexibility and allowed participants' perspectives to be incorporated, thus proving the most practical approach for comprehensive data collection and analysis (Creswell & Plano Clark, 2018). The methodological design of this study is delineated into various components, which are discussed in subsequent sections.
Research Approach
A research approach encompasses the procedures relied upon in conducting a study, including data collection and analysis. For this study, a deductive approach was adopted, as it aimed to test assumptions, theories, and hypotheses concerning the impact of contract farming on household income among farmers. It aligns with the interpretive framework and research design used in the study.
Research Paradigm
The choice of research paradigm, influenced by philosophical perspectives, was pragmatism. Pragmatism enabled the combination of qualitative and quantitative methods to evaluate a real-life case, accommodating both positivist and interpretivist stances. This approach was selected to address the research questions effectively, given the need for a mixed qualitative and quantitative approach.
Study Area
The research was conducted among smallholder cotton farmers in Pakwach District, part of the West Nile sub-region in Northern Uganda. The district is characterized by a diverse demographic and geographical landscape, with agriculture as the primary economic activity. This region is recognized for its traditional cotton cultivation and predominantly rural settings. Moreover, the study area falls within one of Uganda's poorest regions, as reported by the 2019/2020 Uganda National Household Survey (UBOS, 2021).
3.4 Population and Time Frame
The population, comprising smallholder cotton farmers, was estimated to be around 250,000 households involved in cotton farming (CDO, 2020). Primary data was collected from 384 smallholder farmers, of which 164 were contracted and 220 were non-contracted cotton farmers. Data collection took place from December 2021 to January 2022.
3.5 Sampling and Sample Size Determination
A multi-level random sampling technique was employed to select the study respondents. The sample size was determined using a formula, resulting in 384 households selected to participate in the study. This included 164 contracted and 220 non-contracted cotton farmers. The larger sample of non-contracted farmers was chosen to serve as a control group for data analysis.
3.6 Inclusion and Exclusion Criteria
Participants in this study included smallholder farmers engaged in cotton cultivation for at least three years, aged 18 and above to provide informed consent for sharing information related to household income.
3.7 Data Collection Instruments
Primary data collection involved structured questionnaires with both open-ended and closed-ended questions, allowing for qualitative and quantitative data collection. Additionally, Focused Group Discussions (FGD) and Key Informants Interviews (KII) were conducted to supplement data from questionnaires. The information collected from these instruments was further validated using secondary data from official sources.
Validity and Reliability of Research Instruments
Pilot testing of questionnaires was conducted with 30 participants, ensuring the reliability and validity of the instruments. Ongoing integrity checks were performed during the interview process to maintain data quality.
3.8 Data Collection and Analysis
Data Collection
Data collection was carried out through structured questionnaires administered by trained enumerators using the KoBoCollect software. Out of 384 questionnaires, data from 361 respondents were considered valid for further analysis, after accounting for various challenges in data collection.
Data Analysis Strategy
The data processing procedure involved editing, coding, classification, and tabulation using Excel. The data was transformed into a quantitative format for analysis using statistical software.
3.9 Impact Evaluation Method
Impact evaluation methods were considered to analyze the effects of CF participation on household income. Propensity Score Matching (PSM) was selected as the most suitable method, given its capability to handle selection bias and the absence of observational data on the control group.
Factors Responsible for CF Participation Among Farmers
The study investigated the main factors influencing CF participation using a range of demographic and socio-economic factors. The relationship was analyzed as follows:
CF participation = ƒ(demographic and socio-economic factors)
Evaluating the Impact of CF Participation
The study aimed to assess the impact of CF participation on household income. The research employed Propensity Score Matching (PSM) as the chosen method for estimating this impact. This approach was considered most suitable for addressing selection bias and the absence of observational data on the control group, offering robust results.
Empirical Model Specification
To address the research question concerning the main factors that influence smallholder farmers' participation in Contract Farming (CF), a regression analysis was conducted. This analysis aimed to predict the variation in the response variable, which was the participation in CF, based on a set of explanatory variables that included demographic and socio-economic factors identified in this study. This approach helped mitigate endogeneity issues that could arise from the inclusion of a dummy variable for CF participation.
The first step in the model was to estimate the probability of a household's participation in CF by obtaining propensity scores. This was accomplished using a logistic regression model. Logistic regression was chosen because it is appropriate for modeling binary dependent variables (in this case, 1 for participation in CF and 0 for non-participation) and avoids issues of bias and ensuring the probabilities fall within the permissible range of 0 to 1.
The basic regression model :
𝑌𝑖 = 𝛼0 + 𝛼1 '𝑋𝑖+ 𝜀𝑖
Where:
- 𝑌 𝑖 represents participation in CF (1 for participation, 0 for non-participation).
- 𝑋 𝑖 includes all the explanatory variables.
- 𝛼 0 is the intercept.
- 𝛼 1 represents slope coefficients.
The probability of a farmer's participation in CF was estimated using a logistic regression model and took the form of a cumulative logistic distribution function:
Pri = 𝐸 (𝑌 𝑖= 1| X𝑖) = α0 + α1 𝑋𝑖
The Sigmoid function, defined as S(z) = 1 / (1 + e^(-zi)), was employed to map the expected predictions to probabilities of success, where 'zi' was defined as α0 + α1 X𝑖. The Sigmoid function is valuable in cases where probability is predicted as an output because it ensures values are within the range of 0 and 1.
To handle non-linearity, a probability odds ratio (OR) was introduced. The probability odds ratio measures the association between specific explanatory variables and CF participation. It was expressed as:
L𝑖 = ln( 𝑝 r𝑖/ (1 − 𝑝r𝑖)) = 𝑧 𝑖= α0 + α1 ' X𝑖
The interpretation of the estimated slope coefficients in the logistic regression model could be viewed as the change of odds or the probabilistic changes in favor of success. Marginal effects were estimated to interpret these coefficients:
𝜕𝑃 r𝑖 / 𝜕𝑥𝑖= 𝛼 𝜕𝐹(𝑥𝑖α) / 𝜕𝑥𝑖 = 𝛼 exp(−𝑥𝑖α) / (1 + exp(−𝑥𝑖α))^2
This model helped assess the impact of various explanatory variables on the likelihood of CF participation.
The study's literature review provided a theoretical foundation for the explanatory variables related to CF participation.
In the second stage, CF participants were considered the treated group, while non-contracted farmers formed the control group. Propensity scores matching (PSM) was employed to estimate the average treatment effects, comparing these two groups. This technique aimed to account for the selection of participants based on observable characteristics and attribute differences in outcomes to the treatment effect.
PSM required matching each CF participant with one or more non-participants who shared similar observable characteristics. Propensity scores represented the degree of similarity between CF participants and non-participants.
In terms of the ethical considerations, the study ensured the informed consent of participants, protected their privacy, and maintained confidentiality. Measures were put in place to respect the dignity and autonomy of the individuals involved.
3.11 Limitations of the Study and Alternative Strategies
The study acknowledged several limitations, such as time constraints, financial resources, travel restrictions due to COVID-19, and scope limitations. To address these challenges, alternative strategies were adopted. Ethical clearance was obtained promptly, and COVID-19 safety measures were followed. Additionally, if the working hypotheses were invalidated, alternative hypotheses were prepared as the next likely explanation.
Furthermore, as it was not practical to study the entire population of smallholder farmers in Uganda, a sampling strategy was used as a delimitation.
3.12 Conclusion
This chapter outlined the methodology used in the study, explaining the study design, selection of the study area, sampling procedure, data collection, and the empirical model specification. The next chapter will focus on analyzing and interpreting the results to address the research objectives and questions.
CHAPTER 4: DATA ANALYSIS AND INTERPRETATION OF RESULTS
4.0 Introduction
This chapter presents the empirical findings based on farm-level data from Hurungwe district, focusing on the factors influencing smallholder farmers' decisions to participate in tobacco contract farming and the impact of participation on land productivity. The chapter is structured as follows: it begins with descriptive statistics, followed by pre-estimation tests, post-estimation tests, and interpretation of econometric results.
4.1 Descriptive Statistics
In this section, we provide an overview of the characteristics of the study population based on data collected during fieldwork. The dataset includes responses from 361 smallholder farmers, of which 150 were tobacco contract farming participants, representing 42% of the study population, and 211 were non-contracted farmers, representing 58% of the study population.
4.2 Characteristics of the Study Population
The characteristics of the surveyed population are described and summarized below for both continuous and categorical variables.
Table 2: Continuous Variables
Variable | Population N=361 | Contracted N=150 | Non-Contract N=211 | T-Test | |||
---|---|---|---|---|---|---|---|
Mean | Std. Div | Mean | Std.Div | Mean | Std.Div | ||
FAge | 42.29 | 13.15 | 46.67 | 12.1 | 39.17 | 13.02 | |
HH Size | 7.39 | 4.31 | 8.39 | 4.57 | 6.67 | 3.98 | |
Educ | 6.46 | 3.44 | 7.53 | 3.30 | 5.70 | 3.35 | |
Experience | 18.11 | 10.83 | 19.65 | 9.43 | 17.01 | 11.62 | |
Farm Size | 2.65 | 1.67 | 3.58 | 1.98 | 1.99 | 0.98 | |
Crop Yield | 537.25 | 755.54 | 822.66 | 1,003.81 | 334.36 | 405.11 | |
Time to Market | 14.33 | 11.77 | 18.14 | 10.86 | 1.99 | 0.98 | |
Market Price | 2,967.2 | 246.27 | 2,959.0 | 300.1 | 2973.0 | 200.0 |
Table 3: Categorical Variables
Variable | Type | Population N=361 | Contracted N=150 | Non-Contract N=211 | T-Test | |||
---|---|---|---|---|---|---|---|---|
Freq | %ge | Freq. | %ge | Freq. | %ge | |||
Gender | Male | 312 | 86.4 | |||||
Female | 49 | 13.6 | ||||||
Total | 361 | 100.0 | ||||||
Marital Status | Married | 335 | 92.8 | |||||
Not Married | 23 | 7.20 | ||||||
Total | 361 | 100.0 | ||||||
Labour Source | Family | 310 | 85.9 | |||||
Hired | 51 | 14.1 | ||||||
Total | 361 | 100.0 | ||||||
Land Tenure | Customary | 327 | 90.6 | |||||
Leasehold | 33 | 9.4 | ||||||
Freehold | 0.00 | 0.00 | ||||||
Total | 361 | 100.0 | ||||||
Risk Aversion | Yes | 138 | 61.8 | |||||
No | 223 | 38.2 | ||||||
Total | 361 | 100.0 | ||||||
Access to Cr. | Yes | 49 | 13.6 | |||||
No | 312 | 86.4 | ||||||
Total | 361 | 100.0 | ||||||
Acces to Ext | Yes | 269 | 74.5 | |||||
No | 92 | 25.5 | ||||||
Total | 361 | 100.0 | ||||||
Group Member | Yes | 70 | 19.4 | |||||
No | 291 | 80.6 | ||||||
Total | 361 | 100.0 |
Source:Survey data analysis (2022)
CHAPTER FOUR ESTIMATION, PRESENTATION, AND INTERPRETATION OF RESULTS
4.1 Introduction
This chapter presents the empirical findings on the factors influencing smallholder farmers' decisions to participate in tobacco contract farming and the impact of participation on household income, using farm-level data. The chapter begins with descriptive statistics followed by pre-estimation tests, post-estimation tests, and the interpretation of econometric results.
4.2 Descriptive Statistics
In this section, we summarize the data collected from fieldwork. The explanatory variables are categorized as categorical and continuous.
Contracting Firms and Proportion of Participants in Tobacco Contract Farming
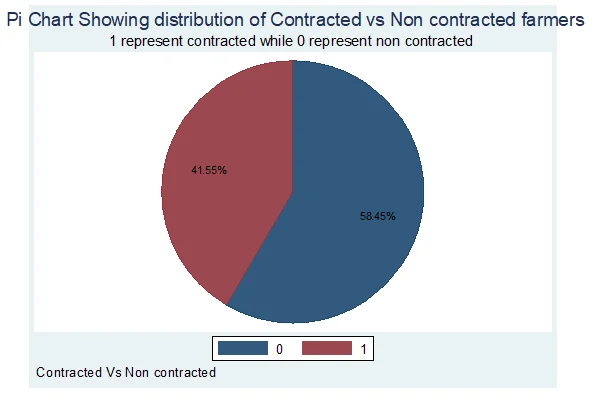
Figure 3 provides insights into the percentage of participants and non-participants in tobacco contract farming.
Figure 3: Number of Contracting Firms that farmers are contracted with and Percentage of Contracted and Non-Contracted Farmers.
The collected data indicates that out of the 361 farmers surveyed, 41.55% (equivalent to 150 farmers) participated in tobacco contract farming, while 58.45% did not.
Descriptive Statistics for Continuous Variables
Table 2 below summarizes the descriptive statistics for continuous variables among both participants and non-participants.
Table 3.1: Descriptive Statistics of Continuous Variables (Overall Sample)
Variable | Obs | Mean | Std.Dev |
---|---|---|---|
FAge | 361 | 42.28809 | 13.14851 |
Hhsize | 361 | 7.387812 | 4.313965 |
FEduc | 361 | 6.459834 | 3.443862 |
FExper | 361 | 18.10803 | 10.83215 |
FSize | 361 | 2.652355 | 1.674665 |
Yield | 361 | 537.2548 | 755.5426 |
Time_mkt | 361 | 14.32964 | 11.77377 |
Mkt_px | 361 | 2967.175 | 246.2695 |
Y_Hh_ | 361 | 2382988 | 5323960 |
4.3 Pre-Estimation Tests
In this section, two important pre-estimation tests were conducted to ensure that the models meet the necessary assumptions and can be relied upon. These tests help validate the statistical methods used.
Multicollinearity Test
Multicollinearity is an issue that can affect the reliability of regression models. The presence of multicollinearity makes it difficult to separate the effects of individual independent variables on the dependent variable. To check for multicollinearity, a pairwise correlation matrix was used to examine the inter-correlation among continuous explanatory variables. The results indicate that there are no serious issues of multicollinearity, which suggests that logistic regression is a valid approach for this analysis.
The Overlapping Condition Check
The overlapping condition check is a pre-estimation test conducted before running a treatment evaluation model. This condition ensures that farmers in the participant group have matching partners with close propensity scores in the non-participant group. The matching of these two groups is essential to eliminate self-selection bias and ensure the validity of the treatment evaluation model. The overlapping condition was checked and found to be satisfied, which is a positive sign for the model's validity.
4.4 Post-Estimation Test Results
Diagnostic tests are performed to check whether the model was correctly specified, which is essential to rely on the results for policy recommendations.
Goodness of Fit of the Logit Model
The p-value obtained from the Hosmer-Lemeshow was less than 0.001, indicating that the logistic model was correctly specified. This suggests that the model's results can be relied upon for policy recommendations.
The Balancing Condition Property Check
The Balancing Condition test was performed using a Balance Box plot. The results showed that the property is satisfied, implying that the distribution of confounding factors was similar among the participant and non-participant matched samples. This indicates that self-selection bias was eliminated, allowing for the computation of the average treatment effect on the treated (ATET).
4.5 Econometric Results
This section presents the results obtained from both the Logistic regression and the treatment evaluation model.
Factors Influencing Smallholder Participation in Tobacco Contract Farming
It's important to note that the coefficients in logistic regression indicate the direction of change in probabilities rather than the magnitude of the change. Therefore, interpreting the results using marginal effects is recommended.
Treat | Coef. | St.Err. | t- value | p- value | [95%Conf | Interval] |
---|---|---|---|---|---|---|
FStatus | 1.063 | 0.912 | 1.17 | 0.244 | -0.724 | 2.850 |
FAge | 0.046 | 0.031 | 1.47 | 0.140 | -0.015 | 0.108 |
Hhsize | -0.195 | 0.061 | -3.18 | 0.001 | -0.315 | -0.075 |
FEduc | 0.140 | 0.051 | 2.74 | 0.006 | 0.040 | 0.240 |
FExper | 0.058 | 0.034 | 1.69 | 0.091 | -0.010 | 0.126 |
FLabour | 1.680 | 0.894 | 1.88 | 0.060 | -0.073 | 3.432 |
FSize | 0.769 | 0.230 | 3.34 | 0.001 | 0.317 | 1.220 |
Land_ten | -0.374 | 0.766 | -0.49 | 0.625 | -1.876 | 1.127 |
Yield | 0.001 | 0.000 | 1.36 | 0.175 | -0.000 | 0.001 |
Pdn_Risk | 1.466 | 0.868 | 1.69 | 0.091 | -0.235 | 3.167 |
Access_cr | 0.804 | 0.795 | 1.01 | 0.312 | -0.755 | 2.362 |
Time_mkt | -0.052 | 0.030 | -1.73 | 0.083 | -0.111 | 0.007 |
Mkt_px | 0.000 | 0.001 | 0.12 | 0.908 | -0.001 | 0.001 |
Px_risk | -2.179 | 0.926 | -2.35 | 0.019 | -3.993 | 0.365 |
Access_ext | 5.422 | 1.269 | 4.27 | 0.000 | 2.934 | 7.909 |
Fgroup | 1.549 | 0.547 | 2.83 | 0.005 | 0.476 | 2.621 |
Constant | -10.00 | 2.846 | -3.52 | 0.000 | -15.58 | -4.426 |
Table 4: Logistic Regression Output (Propensity Scores)
This table presents the coefficients for various explanatory variables in the logistic regression model, along with their standard errors, t-values, and p-values.
Here is a brief interpretation of some of the results:
- Gender (FStatus):The coefficient is positive, indicating that being male increases the probability of participating in tobacco contract farming by 11.0%. This aligns with the expectation that men are more likely to engage in strenuous farming activities like tobacco farming.
- Age (FAge):The coefficient is negative, suggesting that being older reduces the probability of participating. Specifically, being older by one year reduces the probability by 2.6%.
- Education (FEduc):More years of education increase the probability of participation. An increase of one year in education leads to a 7.0% higher probability of participation.
- Farming Experience (FExper): Having more experience in tobacco farming increases the chance of participation. An additional year of experience leads to a 2.5% higher probability of participation.
- Family Labor (FLabour): The coefficient is positive, indicating that having more family labor increases the probability of participation.
- Farm Size (FSize):A larger farm size increases the probability of participation. A one-unit increase in farm size leads to an 8.0% higher probability of participation.
- Access to Extension Services (Access_ext):Access to extension services significantly increases the probability of participation. Farmers with access have a 56.2% higher probability of participating.
These interpretations provide insights into how each variable affects the likelihood of smallholder farmers participating in tobacco contract farming.
Interpretation of Logit Marginal Effects Results
Table 6 presents the average marginal effects, which provide information about the marginal change in the probability of success given a change in the explanatory variables. Here are some key interpretations:
- Gender (FStatus): The marginal effect is 0.110, which means that being male increases the probability of participation by 11.0%.
- Age (FAge): The marginal effect is 0.005, suggesting that being one year older reduces the probability of participation by 0.5%.
- Education (FEduc): An additional year of education increases the probability of participation by 1.4%.
- Farming Experience (FExper):Each additional year of experience in tobacco farming increases the probability of participation by 0.6%.
- Farm Size (FSize): An increase of one unit in farm size leads to an 8.0% higher probability of participation.
- Access to Extension Services (Access_ext):Having access to extension services increases the probability of participation by 56.2%.
These interpretations offer a deeper understanding of how changes in these variables impact the probability of smallholder farmers participating in tobacco contract farming.
Conclusion
In conclusion, this study delved into the factors influencing smallholder farmers' decisions to participate in tobacco contract farming and assessed the impact of participation on land productivity in the Hurungwe district of Mashonaland West province, Zimbabwe. Through rigorous empirical analysis, the following key findings have emerged:
- Factors Influencing Participation: Gender, farmer's age, education level, farming experience, access to the internet, proximity to the main road, and the reputation of contracting firms significantly influence smallholder farmers' participation in tobacco contract farming. Specifically, being male, younger, better educated, more experienced in farming, having access to the internet, and considering the firm reputable increase the likelihood of participation.
- Positive Impact of Contract Farming:The study revealed that participation in tobacco contract farming has a positive impact on land productivity, specifically, the yield per hectare. Farmers engaged in contract farming realized a higher yield per hectare on average compared to non-participating farmers. The estimated average treatment effect on the treated (ATET) suggests that, on average, contract farmers achieved 1,089,518 kilograms more yield per hectare than non-participants.
Policy Implications and Recommendations
Based on the empirical findings, this study offers several policy implications and recommendations:
- Promoting Contract Farming:Policymakers and agricultural authorities should encourage the expansion of contract farming arrangements. Such initiatives can contribute to improving land productivity and overall agricultural output. These policies should be designed to facilitate and incentivize both farmers and contracting firms to participate in contract farming.
- Firm Reputation:It is essential for contracting firms to maintain a good reputation by fulfilling their promises to farmers and honoring the conditions of the contracts. Reliable and reputable contracting firms are more likely to attract smallholder farmers, leading to increased participation.
- Education and Training:Encourage farmers to invest in education and training related to tobacco farming. This knowledge equips them with the skills and expertise required to enhance yield per hectare. Extension services should be made available to smallholder farmers, providing them with the necessary training and information.
- Access to Capital: Make capital more accessible to farmers through partnerships with contracting firms, banks, and other financial institutions. Access to capital can enable farmers to invest in better farming practices and inputs, ultimately increasing land productivity.
- Infrastructure Development:Local authorities and the government should invest in road construction and maintenance. Good road infrastructure makes it easier for farmers to access markets, obtain necessary inputs, and engage with contracting firms. The study highlighted that farmers near main roads were more likely to participate in contract farming.
- Internet Access:Efforts should be made to provide affordable internet access to rural areas. This can empower farmers with information, market access, and tools for better decision-making. Network providers can play a vital role in expanding internet coverage in farming regions.
Limitations of this Study and Areas where Further Research is necessary
This study, while providing valuable insights, is not without limitations.
- Sample Size:The study's sample size was relatively small (160 smallholder tobacco farmers). Future research with a larger sample size can provide more comprehensive and generalizable results.
- Data Analysis:The use of advanced treatment evaluation tools like Difference in Differences (DID) should be explored for more robust results. DID analysis can help compare yield changes between participants and non-participants before and after participating in contract farming programs.
- Long-Term Impact: Investigating the long-term impact of contract farming on land productivity and farmers' livelihoods is an area that warrants further research. Understanding how these effects evolve over time is crucial for designing sustainable agricultural policies.
In conclusion, this study contributes to the understanding of smallholder farmers' decisions to participate in tobacco contract farming and the positive impact of participation on land productivity. The policy recommendations aim to support and enhance the adoption of contract farming, ultimately benefiting both farmers and the agricultural sector in Zimbabwe. Further research can build upon these findings to deepen our understanding of the dynamics between contract farming and land productivity.
Similar Samples
Explore a diverse array of exemplary assignments showcased in our sample section. Gain insight into the depth and caliber of our statistical solutions. Each sample meticulously reflects our proficiency and clarity in statistical analysis. Experience firsthand the excellence that awaits you in the realm of statistical assistance.
Statistics Research