- Problem Description
- Data Attributes and Instances
- Pre-processing Tasks
- Data Mining Modeling Techniques
- Results
- Interpretation of Results
- Concluding Remarks
Adolescent Eating Disorders Data Mining Report presents a comprehensive analysis of adolescent eating disorders through advanced data mining techniques. The study delves into a dataset of 220 observations over four years, focusing on 55 patients with eating disorders and their symptoms. Rigorous pre-processing tasks ensured data accuracy, covering cleaning, normalization, transformation, and handling missing values. The application of decision trees, random forest, and k-means clustering techniques provided actionable insights for the diagnosis and treatment of patients, with significant symptoms identified and recommendations outlined.
Problem Description
This report delves into the exploration of adolescent eating disorders, particularly emphasizing anorectic and bulimic behaviors. The dataset encompasses 220 observations spanning four years, with a comprehensive evaluation of 55 patients suffering from eating disorders who were assessed for 16 symptoms during each observation. The primary objective here is to unveil patterns and connections within the data, aiming to provide valuable assistance for your Data Mining assignment concerning the diagnosis and treatment of patients grappling with eating disorders.
Data Attributes and Instances
The dataset consists of 20 attributes, including patient information and symptom scores, with 220 instances collected at four-time points. The study involves 55 adolescents with known eating disorders, each observed four times over four years. The pre-processing tasks ensured the data's readiness for analysis, resulting in 217 valid observations after handling missing values.
Pre-processing Tasks
The data underwent various pre-processing tasks to ensure accuracy in analysis:
- Data Cleaning:
- Checked for inconsistencies and errors.
- Removed duplicates (none found).
- Examined structural issues through frequency distribution (none found).
- Normalizing:
- Ensured variables were on the same scale.
- Transforming:
- Altered data format for compatibility with SPSS.
- Handling Missing Values:
- Imputed missing values using statistical methods.
Data Mining Modeling Techniques
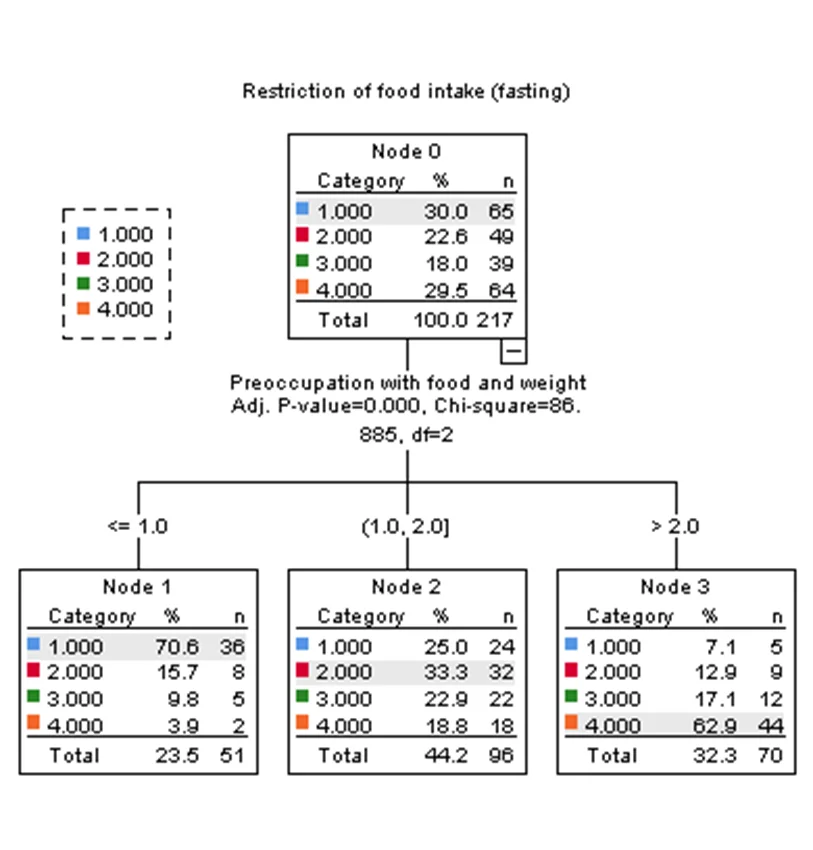
Three data mining techniques were applied to identify patterns and relationships:
- Decision Trees:
- Built a decision tree for classification.
- Identified significant symptoms: Binge Eating, Body Weight, Preoccupation with Food and Weight.
- Accuracy: 51.6%.
- Created a model using multiple decision trees.
- Identified significant symptoms: Body Weight, Binge Eating, Preoccupation with Food and Weight, Purging.
- Accuracy: 85%.
- Revealed three patient clusters based on symptom scores.
Results
Decision Tree Model
- Accuracy: 51.6%
- Significant Symptoms: Binge Eating, Body Weight, Preoccupation with Food and Weight.
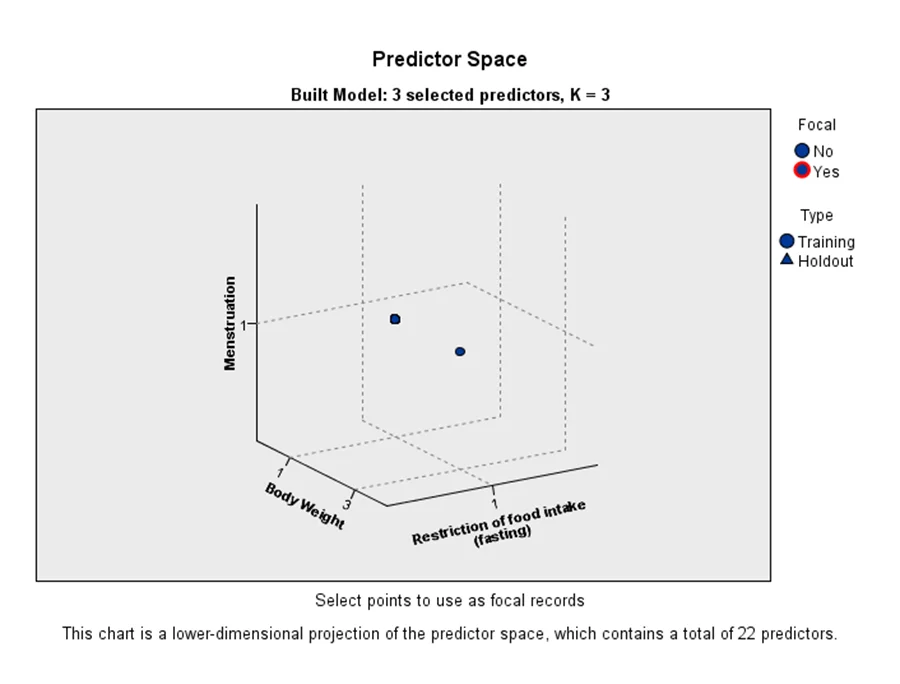
Random Forest Model
- Accuracy: 85%
- Significant Symptoms: Body Weight, Binge Eating, Preoccupation with Food and Weight, Purging.
K-means Clustering
- Cluster 1: Purging, Vomiting, Menstruation
- Cluster 2: Body Weight, Preoccupation with Food and Weight, Mental State (Mood)
- Cluster 3: Binge Eating, Family Relations, Friends
Interpretation of Results
The models identified Body Weight, Binge Eating, Preoccupation with Food and Weight, and Purging as significant symptoms. Random Forest outperformed Decision Trees in accuracy. Recommendations include focusing on weight management, monitoring eating habits, and counselling patients with preoccupation with food and weight.
Concluding Remarks
The data mining techniques provided valuable insights for diagnosing and treating adolescent eating disorders. While the models showed promising results, limitations include ordinal data and oversimplified patient diagnosis. Future studies should consider additional factors like genetics for a more comprehensive analysis.
You Might Also Like
Explore our diverse sample collection for insightful assignments covering fundamental concepts to advanced methodologies. Dive into a wealth of topics and solutions tailored to your needs. Unlock valuable knowledge and inspiration through our curated samples.
Data Mining