Avail Your Offer Now
Celebrate the festive season with an exclusive holiday treat! Enjoy 15% off on all orders at www.statisticsassignmenthelp.com this Christmas and New Year. Unlock expert guidance to boost your academic success at a discounted price. Use the code SAHHOLIDAY15 to claim your offer and start the New Year on the right note. Don’t wait—this special offer is available for a limited time only!
We Accept
- The Basics of ANOVA
- Exploring Welch's ANOVA
- T-Test vs. ANOVA
- Manual Calculation of One-Way ANOVA
- Omnibus Tests and Their Role
- Performing One-Way ANOVA in R and Python
- Calculating the Grand Mean
- Conclusion
When it comes to analyzing data, understanding the different statistical methods available can make a significant difference in the quality and accuracy of your results. ANOVA (Analysis of Variance) is a crucial technique for comparing means across multiple groups and determining whether any observed differences are statistically significant. This method is particularly useful when you need to evaluate more than two groups simultaneously. Among the various forms of ANOVA, Welch’s ANOVA stands out as a robust alternative for situations where the assumption of equal variances is not met.
In this blog, we will explore the fundamentals of ANOVA, focusing on Welch’s Test and its application in real-world scenarios. We will also examine how T-tests compare with ANOVA and discuss their respective uses. Understanding these concepts is essential not only for academic success but also for practical data analysis. For students seeking help with ANOVA assignments, this blog will provide valuable insights into how ANOVA techniques, including Welch’s ANOVA, can be applied effectively. By delving into these statistical methods, we aim to equip you with the knowledge needed to tackle your analyses confidently. Whether you are working on an ANOVA assignment or dealing with complex datasets, a clear grasp of ANOVA and its variants will enhance your ability to draw accurate conclusions and present meaningful results. Join us as we break down these essential statistical tools and uncover their practical applications in both academic and professional contexts
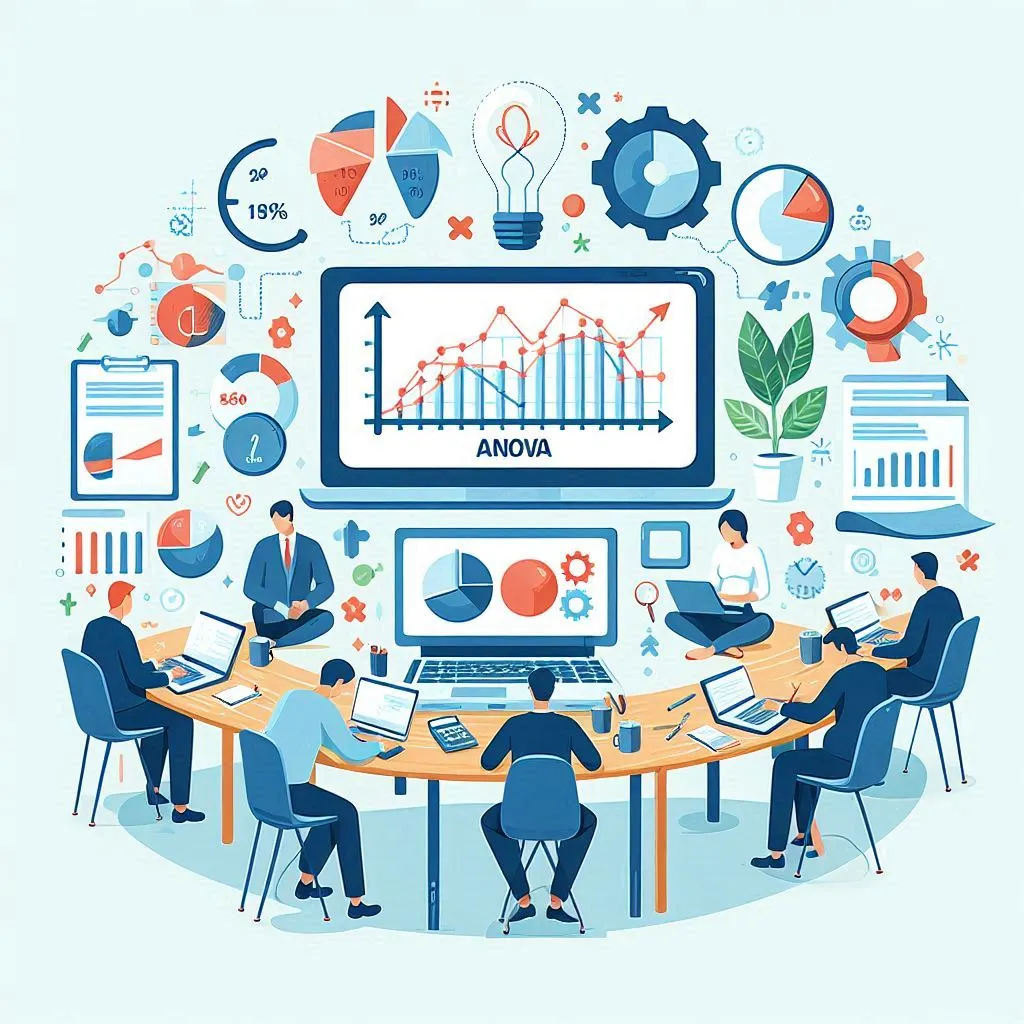
The Basics of ANOVA
ANOVA is a powerful tool used when comparing the means of three or more groups. Unlike a T-test, which compares the means between two groups, ANOVA assesses whether there are any statistically significant differences among multiple groups simultaneously. The core idea behind ANOVA is to partition the total variance observed in the data into components attributable to different sources—typically the variation between groups and the variation within groups.
The main goal of ANOVA is to determine whether the differences in means are significant enough to reject the null hypothesis, which posits that all group means are equal. If the test reveals significant differences, it indicates that at least one group mean is different from the others.
Exploring Welch's ANOVA
Welch's ANOVA is a variation of the traditional ANOVA designed to handle situations where the assumption of equal variances across groups is not met. This test is particularly useful when dealing with heterogeneous variances and unequal sample sizes, which are common in real-world data.
The procedure for Welch’s ANOVA involves comparing the means of groups with potentially unequal variances and sample sizes. It adjusts the degrees of freedom used in the test to account for these discrepancies, making it a robust choice for many practical applications. Welch’s ANOVA does not assume homogeneity of variances, unlike the traditional ANOVA, which makes it more flexible in handling real-world data where variances might differ across groups.
T-Test vs. ANOVA
To understand the differences between a T-test and ANOVA, it’s essential to grasp their purposes and applications.
- T-Test: A T-test is used when comparing the means of two groups. For example, it might be used to compare the average test scores of two different teaching methods. The T-test evaluates whether the observed difference between the means of these two groups is statistically significant or if it could have occurred by chance.
- ANOVA: In contrast, ANOVA is employed when comparing three or more groups. Suppose you are evaluating the effectiveness of three different teaching methods. ANOVA helps determine whether there are any significant differences among the means of these three groups. If the ANOVA results are significant, it suggests that at least one group’s mean is different from the others. However, ANOVA does not tell you which specific groups differ; further post-hoc tests are required for this.
In summary, while the T-test is suitable for comparing two means, ANOVA is designed for more complex scenarios involving multiple groups.
Manual Calculation of One-Way ANOVA
Performing a One-Way ANOVA manually involves several steps to understand the underlying mechanics of the test. Here’s a simplified outline of the process:
- Calculate Group Means: For each group in your data, compute the mean value.
- Calculate the Grand Mean: This is the average of all observations combined, regardless of group.
- Determine Variability: Compute the between-group variability (how much group means differ from the grand mean) and within-group variability (how individual observations vary within each group).
- Compute the F-Statistic: This is the ratio of between-group variance to within-group variance. A higher F-statistic indicates a greater likelihood that group means are different.
- Interpret the Results: Compare the F-statistic to a critical value from the F-distribution to determine if the observed differences are statistically significant.
Manual calculations provide valuable insights into the process of ANOVA and help in understanding how the statistical software performs these computations.
Omnibus Tests and Their Role
Omnibus tests, such as ANOVA, assess whether there are significant differences among multiple groups. The term "omnibus" refers to the broad nature of these tests—they determine whether any differences exist but do not specify which groups differ.
For example, if an ANOVA test shows a significant result, it means that there is evidence that at least one group mean is different. However, to pinpoint which specific groups differ, additional tests are required. These follow-up tests are often referred to as post-hoc tests or pairwise comparisons, which help in identifying exactly where the differences lie.
Performing One-Way ANOVA in R and Python
Both R and Python provide robust tools for performing ANOVA, making them invaluable for statistical analysis. In R, the oneway.test function can be used for Welch's ANOVA, while the aov function is typically used for traditional ANOVA. For Python users, the scipy.stats library offers the f_oneway function for conducting ANOVA.
The advantage of using these programming languages for ANOVA is their ability to handle large datasets and perform complex calculations efficiently. Both R and Python also offer extensive libraries and packages that facilitate statistical analysis, making it easier for students and researchers to conduct and interpret ANOVA.
Calculating the Grand Mean
The grand mean is a fundamental concept in ANOVA, representing the overall average of all observations across groups. To calculate the grand mean, sum all individual data points and divide by the total number of observations. This value is crucial for computing the variance between groups and understanding the overall distribution of your data.
In practice, the grand mean serves as a reference point against which the means of individual groups are compared. It helps in assessing whether the differences between group means are substantial enough to be considered statistically significant.
Conclusion
Understanding and applying ANOVA is crucial for analyzing complex data and drawing insightful conclusions. By delving into Welch's ANOVA, comparing T-tests and ANOVA, performing manual calculations, and utilizing statistical software, you develop a thorough understanding of how to assess differences between group means. This knowledge equips you with the skills necessary to tackle various statistical assignments and research challenges with confidence.
This blog provides a detailed overview of ANOVA and its applications, offering practical insights and explanations that can support your academic endeavors. Whether you’re seeking statistics assignment help or aiming to refine your analytical skills, this resource is designed to enhance your statistical analysis capabilities and guide you through the complexities of data evaluation.