Claim Your Offer
Unlock a fantastic deal at www.statisticsassignmenthelp.com with our latest offer. Get an incredible 10% off on all statistics assignment, ensuring quality help at a cheap price. Our expert team is ready to assist you, making your academic journey smoother and more affordable. Don't miss out on this opportunity to enhance your skills and save on your studies. Take advantage of our offer now and secure top-notch help for your statistics assignments.
We Accept
- Understanding the Basics of SPSS
- Data Entry and Data Import
- Descriptive Statistics
- Hypothesis Testing
- Correlation and Regression
- Data Visualization
- Data Transformation and Variable Recoding
- Conclusion
Understanding the Basics of SPSS
Understanding the basics of SPSS is crucial for any data analysis project. SPSS (Statistical Package for the Social Sciences) is a powerful software widely used in various fields to perform statistical analyses and interpret data. It provides an intuitive interface, making it accessible to both beginners and experienced researchers. By learning the fundamentals of data entry, importing, and cleaning, users can ensure accurate and reliable analyses. Moreover, mastering descriptive statistics, hypothesis testing, and data visualization will enable researchers to draw meaningful insights from their data. This foundational knowledge sets the stage for more advanced statistical analyses and a successful SPSS journey.
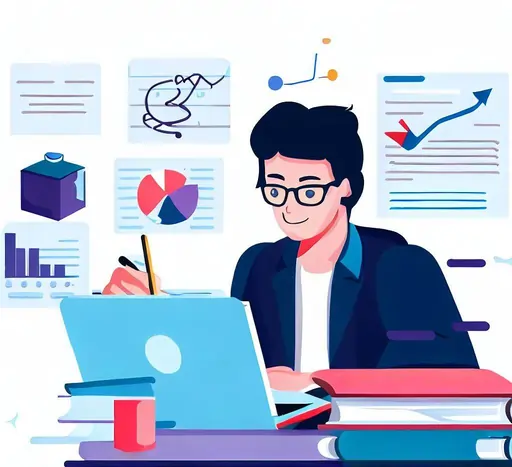
The following topics are essential to know:
Data Entry and Data Import
Data entry and data import are critical steps in the SPSS workflow. Properly organizing and entering data is essential for accurate analysis and valid results. SPSS offers various methods to input data, including manual entry or importing from external sources like Excel or CSV files. Understanding how to handle missing data and outliers during this process is crucial to ensure data integrity. Additionally, knowing how to label variables and assign value labels improves data clarity and interpretation. By mastering data entry and import, researchers can avoid data errors, save time, and lay a solid foundation for a successful SPSS assignment.
Some of the assignments you can expect on data entry and data import include:
- Data Entry Accuracy Assessment: To solve a data entry accuracy assessment assignment, carefully enter the provided dataset into SPSS while minimizing errors. Double-check the data for accuracy and correct any mistakes. Use validation techniques such as cross-referencing with the original data source. Analyze any discrepancies and document your approach to ensure transparency. This exercise helps improve data entry skills and emphasizes the importance of accurate data handling for reliable statistical analysis.
- Data Import and Cleaning: To solve a data import and cleaning assignment, start by importing the dataset into SPSS from various file formats (Excel, CSV). Address missing values, duplicates, and outliers. Check data consistency and validity. Employ functions for data cleaning, like recoding variables or imputing missing values. Document your steps clearly. Lastly, validate the cleaned dataset for accuracy and usability before proceeding with any further analysis.
- Merging Datasets: To solve an assignment on merging datasets in SPSS, follow these steps. First, ensure datasets have a common identifier (e.g., ID). Use the "Merge Files" function, select appropriate merge type (e.g., inner, outer), and identify the matching variable. Check for duplicate records and resolve inconsistencies. Use the "Split File" option for separate analyses. Validate the merged dataset by comparing results with the original files. A successful assignment requires understanding data relationships and using SPSS tools accurately for a comprehensive analysis.
- Longitudinal Data Handling: To solve an assignment on longitudinal data handling, first, understand the dataset's structure and time points. Organize the data in SPSS, ensuring it's in the appropriate format (wide or long). Use the "Restructure Data" or "Split File" functions to perform time-series analysis. Apply statistical techniques such as repeated measures ANOVA or growth curve modeling to examine trends and changes over time. Finally, interpret and present the findings, showcasing a clear understanding of the data's longitudinal nature and demonstrating analytical skills.
Descriptive Statistics
Descriptive statistics play a fundamental role in data analysis by providing a concise summary of the main features within a dataset. These statistics, including measures like mean, median, mode, standard deviation, and variance, offer valuable insights into the central tendency, spread, and distribution of the data. Understanding descriptive statistics in SPSS allows researchers to gain a clear understanding of their data before moving on to more complex analyses. Additionally, visual representations, such as histograms and box plots, help researchers identify patterns and outliers, making it easier to make informed decisions and draw meaningful conclusions from the data at hand.
Here are the types of assignments you will get on descriptive statistics and how you can solve them:
- Central Tendency Assignment: To solve a central tendency assignment, import the dataset into SPSS, calculate the mean, median, and mode using the "Descriptive" option, and interpret the results. The mean represents the average, the median is the middle value, and the mode is the most frequent value in the dataset, providing insights into the central tendencies of the data.
- Measures of Dispersion Assignment: To solve a measures of dispersion assignment, import the dataset into SPSS, then calculate the range, standard deviation, and variance using the "Descriptive" option. Interpret the results to understand the spread of the data, identifying the variability and distribution characteristics.
- Frequency Distribution Assignment: To solve a frequency distribution assignment, import the dataset into SPSS, then use the "Frequencies" option to generate frequency tables for the variables of interest. Additionally, create histograms to visualize the distribution. Analyze the frequency tables and histograms to identify patterns and trends in the data.
- Correlation Assignment: To solve a correlation assignment, first, import the dataset into SPSS. Choose the variables you want to explore for correlation. Use the "Correlations" option to calculate correlation coefficients. Interpret the results to determine the strength and direction of the relationship between the variables, considering statistical significance using p-values.
Hypothesis Testing
Hypothesis testing is a fundamental concept in statistics and plays a pivotal role in research and decision-making processes. In SPSS, researchers can examine whether their hypotheses are supported or refuted based on empirical evidence. By setting up null and alternative hypotheses and using appropriate statistical tests like t-tests or ANOVA, analysts can draw conclusions about the population from a sample. Understanding p-values, significance levels, and the correct interpretation of results are essential to avoid drawing incorrect conclusions. Hypothesis testing in SPSS empowers researchers to make data-driven decisions and contributes to the validity and reliability of their research findings.
Types of Hypothesis Testing Assignments:
- One-Sample T-Test Assignment: In this assignment, you are given a dataset with a single sample, and you need to test whether the sample mean differs significantly from a hypothesized value. Use SPSS to perform a one-sample t-test. Enter the data, set the null hypothesis, select the t-test option, and interpret the result based on the p-value and significance level.
- Independent Samples T-Test Assignment: In this assignment, you are provided with two separate datasets representing independent groups, and you need to determine if there is a significant difference in the means of the two groups. Input the data, set the null hypothesis, select the t-test option, and interpret the outcome based on the p-value and significance level.
- Paired Samples T-Test Assignment: In this assignment, you are given two related datasets, and your task is to examine if there is a significant difference between the means of the paired samples. Use SPSS to execute a paired samples t-test. Enter the paired data, set the null hypothesis, select the t-test option, and interpret the results using the p-value and significance level.
- One-Way ANOVA Assignment: In this assignment, you are provided with a dataset containing multiple groups, and you need to ascertain if there are significant differences in means across those groups. Employ SPSS to perform a one-way ANOVA. Enter the data, set the null hypothesis, select the ANOVA option, and interpret the result based on the p-value and significance level. Additionally, post-hoc tests may be required to identify specific group differences.
Correlation and Regression
Correlation measures the relationship between two or more variables, while regression predicts the value of a dependent variable based on one or more independent variables. These topics are often encountered in research and data analysis. Knowing how to perform correlation and regression analyses in SPSS will enable you to explore relationships and make predictions from your data.
- Simple Correlation Analysis Assignment: For this assignment, calculate and interpret the correlation coefficient between two variables using SPSS. Identify the strength and direction of the relationship and present your findings in a clear and concise manner.
- Multiple Regression Assignment: In this task, perform multiple regression analysis in SPSS to predict a dependent variable based on two or more independent variables. Select relevant variables, run the regression, and interpret the coefficients to draw meaningful conclusions.
- Correlation and Regression Comparison Assignment: Compare and contrast correlation and regression analyses in SPSS. Explain their purposes, assumptions, and interpretations. Provide examples to demonstrate their applications in different scenarios.
- Real-Life Data Analysis Assignment: Obtain a dataset with variables suitable for correlation and regression analysis. Clean the data, perform the appropriate analysis in SPSS, and interpret the results. Discuss the practical implications of the findings in a real-world context.
Data Visualization
Data visualization plays a pivotal role in understanding complex datasets and communicating insights effectively. SPSS offers a wide range of visualization options, such as histograms, scatter plots, and bar charts, allowing researchers to present data in a visually engaging manner. By choosing the appropriate charts, researchers can identify patterns, trends, and outliers, making it easier to draw conclusions from the data. Furthermore, visualizations aid in conveying findings to a broader audience, making complex statistical information more accessible and comprehensible. A skillful use of data visualization in SPSS enhances the clarity and impact of research results, thereby strengthening the overall research narrative.
Types of data visualization assignments:
- Creating Descriptive Visualizations: In this type of assignment, you may be asked to generate descriptive visualizations for a given dataset using SPSS. Start by importing the data and exploring its variables. Use appropriate chart types such as histograms, bar charts, and pie charts to visualize the distribution of categorical and numerical variables. Customize the visuals by adding labels, titles, and color schemes to improve clarity. For numerical data, consider box plots and scatter plots to identify outliers and patterns. Present the visualizations along with a brief interpretation of the main insights.
- Comparative Visualizations: In a comparative visualization assignment, you might need to compare two or more groups or variables. Use grouped bar charts, stacked bar charts, or line graphs to demonstrate the differences between the groups. Apply color coding and legends to make the visualizations more informative. For more advanced analyses, consider using heatmaps or radar charts to display multivariate comparisons. Explain the key findings and any significant trends or patterns observed in the data.
- Time-Series Visualizations: Time-series visualizations involve displaying data points over time. Use line graphs or area charts to represent the trends and changes in the data over specific time intervals. Pay attention to the x-axis labels and format to ensure the time is displayed accurately. Utilize different line styles or colors for multiple time series. If applicable, add annotations or callouts to highlight important events or occurrences during the time period. Analyze the visualizations to draw conclusions about any temporal patterns or fluctuations.
- Geospatial Visualizations: In geospatial visualization assignments, you will be working with spatial data and representing it on maps. Import the geographic data into SPSS and link it with your dataset. Use choropleth maps to display numerical data for different regions or territories. You can also use bubble maps to show variations in data based on the size of the bubbles in different locations. Customize the map legend, color scales, and data ranges to enhance the visualization's clarity. Analyze the geospatial visualizations to draw insights about spatial patterns and regional differences in the data.
Data Transformation and Variable Recoding
Data transformation and variable recoding are vital skills in SPSS for preparing data for analysis. Data transformation involves converting variables into different formats or scales, such as logarithmic or square root transformations, to meet statistical assumptions. Variable recoding allows researchers to combine or modify existing variables, simplifying the analysis. These techniques are useful when dealing with skewed data or categorical variables. By mastering these methods, researchers can enhance the accuracy and reliability of their analyses and derive more insightful results from their data.
- Log Transformation for Skewed Data: To solve an assignment on log transformation for skewed data, first, identify the skewed variable. Calculate the natural logarithm (ln) of each value in the variable to create a new transformed variable. This process helps normalize the data, making it suitable for analysis that requires normally distributed data.
- Recoding Categorical Variables: To solve an assignment on recoding categorical variables, start by identifying the specific categorical variable and the desired outcome (e.g., binary or multi-category recoding). Create a new variable, assign codes to each category accordingly, and recode the data. Validate the recoded variable's accuracy and use it in subsequent analyses for simplified interpretations.
- Standardization of Variables: To solve an assignment on standardization of variables, calculate the mean and standard deviation for each variable. For each data point, minus the mean and divide the answer by the standard deviation. This process will transform the variables into a common scale with a mean of 0 and a standard deviation of 1, allowing for fair comparisons and unbiased analysis.
- Binning Continuous Variables: To solve an assignment on binning continuous variables, first, determine suitable bin intervals based on the data's distribution and context. Then, divide the range of the continuous variable into these intervals and create a new categorical variable. Assign data points to the corresponding bins, facilitating analysis and interpretation in distinct groups.
Conclusion
Mastering the essential topics in SPSS and knowing how to approach SPSS assignments will empower you to handle various data analysis tasks confidently. By understanding the basics of SPSS, data entry, hypothesis testing, correlation, regression, data visualization, and data transformation, you will be well-prepared to tackle a wide range of statistical problems. Through practice and hands-on experience with SPSS, you can enhance your analytical skills and become proficient in using this powerful statistical software for research and data analysis.