Avail Your Offer Now
Celebrate the festive season with an exclusive holiday treat! Enjoy 15% off on all orders at www.statisticsassignmenthelp.com this Christmas and New Year. Unlock expert guidance to boost your academic success at a discounted price. Use the code SAHHOLIDAY15 to claim your offer and start the New Year on the right note. Don’t wait—this special offer is available for a limited time only!
We Accept
- Understanding Cluster Analysis
- Steps Involved in Cluster Analysis
- Step 1: Data Preprocessing
- Step 2: Selecting Variables
- Step 3: Choosing a Distance Metric
- Step 4: Selecting a Clustering Method
- Step 5: Determining the Number of Clusters
- Step 6: Running the Cluster Analysis
- Benefits of Cluster Analysis in SPSS Assignments
- Data Exploration and Visualization:
- Segmenting Data:
- Profile Identification:
- Hypothesis Generation:
- Decision-Making Support:
- Academic Research:
- Conclusion
Understanding Cluster Analysis
Cluster analysis is a multivariate statistical method that groups similar cases together based on selected variables, thereby creating homogenous clusters. The process involves dividing data points into clusters, with each cluster representing a group of similar cases. This grouping helps to identify patterns, associations, and structures in the data, ultimately leading to a deeper understanding of the underlying relationships.
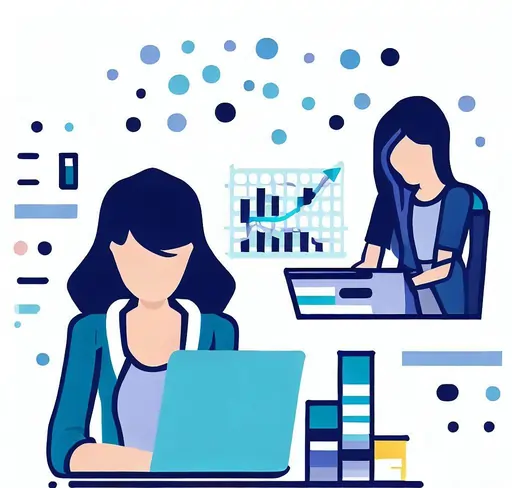
Steps Involved in Cluster Analysis
The process of cluster analysis involves several crucial steps. Data preprocessing ensures data quality, while variable selection is essential for relevant results. Choosing an appropriate distance metric measures data similarity. Selecting a clustering method impacts the formation of clusters. Running the analysis finalizes the groupings.
Step 1: Data Preprocessing
Data preprocessing is a fundamental step in cluster analysis to ensure accurate and reliable results. It involves data cleaning to handle missing values and remove any inconsistencies or errors. Standardizing variables is essential to give them equal weight during clustering, preventing biased results. By normalizing the data, variables with different scales can be brought to a common scale, enhancing the effectiveness of the clustering process. Proper data preprocessing minimizes the impact of noise and irrelevant information, resulting in meaningful and interpretable clusters. It lays the foundation for successful cluster analysis and facilitates the identification of patterns and relationships in the data.
Step 2: Selecting Variables
Selecting the right variables for cluster analysis is a critical step that significantly influences the outcome. The choice of variables should align with the research objectives and the nature of the data. It is essential to pick variables that carry meaningful information and contribute to the clustering process. Including irrelevant or redundant variables can lead to misleading results and hinder the identification of meaningful patterns. Researchers must carefully assess each variable's relevance and potential impact on the cluster formation to ensure the accuracy and validity of the analysis. Proper variable selection sets the foundation for a successful and insightful cluster analysis in SPSS assignments.
Step 3: Choosing a Distance Metric
In Step 3 of cluster analysis, choosing a distance metric is a critical decision that directly affects the outcome. The distance metric measures the similarity or dissimilarity between data points, influencing how the clusters are formed. Common distance metrics include Euclidean distance, which calculates the straight-line distance between points, and Manhattan distance, which measures the sum of the absolute differences in coordinates. Cosine similarity is often used for text or high-dimensional data. Selecting the appropriate distance metric depends on the nature of the data and the research objectives, as it can significantly impact the clustering results and the insights gained from the analysis.
Step 4: Selecting a Clustering Method
Selecting the right clustering method is a pivotal step in the cluster analysis process. Each method has its own strengths and limitations, making it imperative to choose wisely based on the research objectives and data characteristics. K-means clustering is efficient for large datasets and well-separated clusters, while hierarchical clustering provides a more informative dendrogram. Twostep analysis is suitable for datasets with both categorical and continuous variables. Researchers must carefully assess the nature of their data and the desired level of granularity before proceeding with the most appropriate clustering method to obtain meaningful and actionable insights from the analysis.
Step 5: Determining the Number of Clusters
The goal is to find the optimal cluster count that best represents the underlying data structure without overfitting or underfitting. Several methods can aid in this process, such as the Elbow method, which looks for the "elbow point" where the within-cluster sum of squares starts to level off. The Silhouette method calculates a score for each data point, measuring its cohesion within the cluster. Gap statistics compare the clustering results to random data distributions. Properly selecting the number of clusters ensures meaningful and interpretable results from the analysis.
Step 6: Running the Cluster Analysis
After determining the number of clusters and selecting the appropriate method, the cluster analysis is executed to form distinct clusters. Running the analysis involves the application of the chosen clustering algorithm on the dataset. The algorithm assigns each data point to a cluster based on their similarity to other data points. The result is a set of clusters, each representing a group of similar cases. Researchers and students can then analyze the characteristics of each cluster and draw meaningful insights from the data. Proper execution of this step is crucial in obtaining accurate and valuable outcomes from the cluster analysis in SPSS assignments.
Benefits of Cluster Analysis in SPSS Assignments
Cluster analysis offers numerous benefits in SPSS assignments. It aids in data exploration, visualization, and segmentation, enabling students to identify patterns and outliers effectively. The technique also facilitates profile identification, hypothesis generation, and decision-making support. By incorporating cluster analysis, students can demonstrate their understanding of data analysis and draw valuable conclusions from real-world datasets, enhancing the quality of their academic research.
Data Exploration and Visualization:
Cluster analysis in SPSS provides an effective way to explore the underlying structure of the data. By identifying natural groupings, researchers can visualize patterns, trends, and outliers, helping them understand the data more intuitively.
Segmenting Data:
In market research and business analytics, cluster analysis can be used to segment customers or products based on various attributes. This segmentation helps in targeted marketing strategies and product customization, leading to better customer satisfaction and increased revenue.
Profile Identification:
Cluster analysis assists in profiling different groups within a dataset. In social sciences, researchers may use cluster analysis to identify different personality types or customer preferences, facilitating a deeper understanding of their characteristics and behavior.
Hypothesis Generation:
SPSS cluster analysis can help generate hypotheses for further investigation. It can uncover relationships between variables that were not previously considered, prompting researchers to explore new research directions.
Decision-Making Support:
Cluster analysis aids decision-making by providing insights into the underlying data structure. In business applications, it helps managers make informed choices, such as identifying the most profitable customer segment or optimizing inventory management.
Academic Research:
In academic settings, SPSS assignments often involve data analysis. By incorporating cluster analysis into their projects, students can demonstrate their understanding of the subject matter and draw meaningful conclusions from real-world datasets.
Conclusion
Cluster analysis is a valuable tool in SPSS for exploring data, identifying patterns, and aiding decision-making. For students working on SPSS assignments, incorporating cluster analysis can elevate the quality of their research and analysis. By seeking SPSS assignment help from experts, students can ensure they are utilizing cluster analysis effectively and enhancing their understanding of data analysis and its applications in various fields.
You Might Also Like
Experience the thrill of enlightenment as you delve into the depths of statistical theory and application through our blog's enriching content, fueling your intellectual curiosity and thirst for knowledge.