Claim Your Offer
Unlock a fantastic deal at www.statisticsassignmenthelp.com with our latest offer. Get an incredible 10% off on all statistics assignment, ensuring quality help at a cheap price. Our expert team is ready to assist you, making your academic journey smoother and more affordable. Don't miss out on this opportunity to enhance your skills and save on your studies. Take advantage of our offer now and secure top-notch help for your statistics assignments.
We Accept
- Key Concepts and Techniques Essential in Solving SPSS Assignments
- Data Preparation
- Data Exploration
- Variable Recoding and Transformation
- Data Aggregation
- Data Filtering and Subsetting:
- Data Merging and Joins
- Conclusion
Key Concepts and Techniques Essential in Solving SPSS Assignments
In SPSS assignments, mastering key concepts and techniques is crucial for accurate analysis. Data preparation ensures the dataset is clean and ready for analysis. Data exploration uncovers patterns and relationships. Variable recoding and transformation enhance data usability. Data aggregation simplifies complex datasets for better understanding. Data filtering and subletting focus on relevant information, streamlining analyses effectively. Success in SPSS assignments hinges on proficiency in these essential practices.
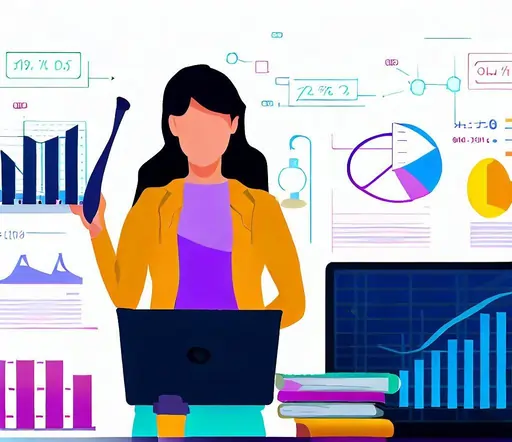
Data Preparation
Data Exploration
Variable Recoding and Transformation
Data Aggregation
Data Filtering and Subsetting:
Data Merging and Joins
- Combining Diverse Data Sources:In real-world scenarios, researchers often collect data from various sources, such as surveys, experiments, or public datasets. Each source may contain unique variables or information related to the research question. By mastering data merging and joins in SPSS, students can seamlessly integrate diverse data sources, ensuring a comprehensive dataset for analysis. This ability is especially valuable when conducting large-scale studies or when attempting to answer complex research questions that require information from multiple angles.
- Enhancing Sample Size: Some assignments may involve working with limited data, which can impact the statistical power of the analysis. Data merging allows students to combine datasets with similar structures but different samples, effectively increasing the sample size. A larger sample size often leads to more robust statistical findings, making the results more reliable and generalizable.
- Analyzing Longitudinal Data: Longitudinal studies involve collecting data over multiple time points from the same subjects. SPSS allows students to merge datasets with repeated measures, enabling them to analyze changes and trends over time. This capability is beneficial when studying the effects of interventions, tracking growth patterns, or examining behavior evolution across different time intervals.
- Handling Missing Data: Missing data is a common issue in research, and SPSS offers various data merging techniques to handle this challenge. By employing appropriate join types and handling missing values with care, students can avoid bias and ensure the validity of their results in SPSS assignments.
- Comparing Variables and Groups:Data merging and joins facilitate comparisons between variables and groups. In SPSS, students can merge datasets based on common variables to create combined datasets that allow for group-wise analysis. This feature proves valuable when examining the differences between various demographic groups, experimental conditions, or treatment responses.
- Preparing Data for Complex Analyses: Some statistical analyses require data in a specific format or structure. Data merging and joins enable students to manipulate the data to meet the requirements of advanced statistical procedures. By organizing data appropriately, students can carry out complex analyses like multilevel modeling, path analysis, or structural equation modeling, advancing their understanding of the research topic and expanding their statistical skills.
Data preparation is a critical phase in any statistical analysis, and it holds particular significance in statistics assignments. When providing statistics assignment help, data preparation becomes the foundation for accurate and reliable results. Proper data preparation ensures that the dataset is clean, organized, and ready for analysis in statistical software like SPSS.
In statistics assignments, students are often provided with raw data that may be incomplete, contain errors, or require restructuring to suit the analysis. Data manipulation techniques come into play during this stage to address these issues effectively. Students need to handle missing data appropriately, either through imputation or exclusion, to avoid biased results. SPSS provides various imputation methods that can be utilized based on the nature of the data and the assumptions of the analysis.
Moreover, data outliers can significantly impact statistical conclusions. By employing data manipulation techniques in SPSS, students can detect outliers and make informed decisions about how to handle them, either by removing them or transforming their values to reduce their influence on the analysis.
Data preparation also involves recoding variables, transforming data into appropriate scales, and aggregating data when necessary. These tasks ensure that the data aligns with the research question and analysis objectives. For instance, students might need to create categorical variables based on continuous data to facilitate comparison between groups or apply logarithmic transformations to normalize skewed distributions.
In addition to handling data anomalies, data manipulation in SPSS enables students to merge datasets from different sources, subset data for specific analyses, and perform data restructuring for complex statistical procedures. Students can manipulate the dataset to fit the chosen statistical models accurately, ensuring the validity of their statistical analyses.
In conclusion, data preparation is the crucial first step in solving statistics assignments with the help of SPSS. Through data manipulation techniques, students can clean, organize, and transform their datasets, setting the stage for meaningful statistical analyses. Proficient data preparation ensures that statistical models and tests are applied accurately, leading to reliable and insightful outcomes in statistics assignments.
Data Exploration plays a crucial role in statistics assignments and is closely tied to data manipulation. When seeking statistics assignment help, students often encounter datasets that require thorough exploration to gain insights and make informed decisions. In this context, data manipulation serves as a fundamental tool to facilitate meaningful data exploration.
Data manipulation allows students to preprocess the dataset by handling missing values, outliers, and organizing the data effectively. By cleaning and preparing the data, students ensure that their exploration is based on reliable and accurate information, which is vital for drawing valid conclusions.
SPSS, being a widely used statistical software, provides a plethora of data manipulation functions that seamlessly integrate with data exploration. Through various visualization tools like scatter plots, histograms, and boxplots, students can explore the distribution and patterns within the dataset. SPSS empowers students to customize visualizations based on different variables, enabling them to identify trends, correlations, and potential outliers.
Furthermore, data manipulation in SPSS allows students to categorize and group data, making it easier to compare and contrast different subsets of the dataset. This is particularly useful when exploring the relationship between variables or studying the characteristics of specific groups within the data.
During data exploration, students might discover the need to recode or transform variables to make them more suitable for analysis. SPSS simplifies this process through data manipulation, enabling students to apply mathematical operations, restructure data, and create new derived variables. Such transformations can be instrumental in revealing hidden insights and patterns within the data.
Data manipulation also facilitates interactive exploration, as students can quickly change parameters and filters in SPSS to observe how different variables interact and influence each other. This iterative process of data exploration through manipulation allows students to refine their analysis iteratively and narrow down their focus on the most relevant aspects of the assignment.
Variable recoding and transformation are vital aspects of data manipulation when it comes to SPSS assignment help. SPSS offers a wide range of tools and functions that allow students to modify, restructure, and recode variables to suit the specific requirements of their statistical analyses.
One crucial role of variable recoding and transformation is data preprocessing. Before performing any statistical analysis, it is essential to preprocess the data appropriately. This step involves handling missing values, outliers, and ensuring the data is in a format suitable for analysis. SPSS enables students to recode categorical variables, merge categories, or combine levels, ensuring the data is organized and ready for further analysis.
Moreover, variable recoding and transformation help simplify analyses, especially in complex datasets with numerous variables. By recoding variables into meaningful categories or grouping levels, students can observe patterns and draw clear conclusions, making it easier to communicate results effectively in their SPSS assignments.
Additionally, these techniques contribute to enhancing the statistical validity of the analyses. Certain statistical tests require data to meet specific assumptions, such as normality and linearity. SPSS empowers students to transform variables to meet these assumptions and ensure the accuracy of their analyses. By performing log transformations or scaling, students can normalize skewed data and achieve linear relationships between variables, which is crucial for accurate interpretation of results.
Data aggregation is a crucial aspect of SPSS assignments as it enables students to extract valuable information from complex datasets and draw insightful conclusions. In SPSS, this process involves summarizing data, merging datasets, and performing calculations to gain a better understanding of the underlying patterns and trends. Here's how data aggregation plays a significant role in SPSS assignments:
One of the primary advantages of data aggregation in SPSS is its ability to simplify complex datasets. When dealing with a large volume of data, aggregation allows students to condense the information, making it more manageable and easier to work with. By computing summary statistics like means, medians, or sums, students can focus on the essential aspects of the data without getting lost in the overwhelming details.
Furthermore, data aggregation in SPSS facilitates comparative analysis. Students can compare groups or categories by aggregating data, which provides a clearer picture of the similarities and differences between them. For example, when analyzing survey responses from different demographic groups, aggregating the data based on relevant criteria allows for more meaningful comparisons and insightful interpretations.
Time-series analysis is another area where data aggregation proves invaluable. Time-series data often contains a large number of data points, making it challenging to discern long-term trends and patterns. By aggregating the data into specific time intervals or summarizing it over periods, students can reveal meaningful insights that might otherwise remain obscured in raw data.
Data aggregation also plays a key role in preparing data for visualization. Effective data visualization is essential for conveying results and findings in a comprehensible manner. By aggregating data, students can create charts, graphs, and visual representations that offer a clear depiction of trends and patterns, enhancing the overall impact of their analysis in SPSS assignments.
In some cases, SPSS assignments may involve combining data from different sources. Data aggregation enables students to merge datasets based on common variables, facilitating a comprehensive analysis that incorporates relevant information from various sources. This ensures a more comprehensive and holistic approach to solving statistical problems.
Data filtering and subsetting are essential data manipulation techniques in SPSS that play a crucial role in statistics assignments. These techniques allow students to extract specific subsets of data from a larger dataset, enabling them to focus on relevant information and streamline their analysis process.
In SPSS, data filtering involves the process of selecting cases or variables based on certain criteria. This is particularly useful when assignments require analysis for specific groups or time periods. By filtering the data, students can exclude irrelevant cases or observations, making the dataset more manageable and suitable for their analysis objectives. For instance, in a survey dataset, filtering responses from a particular age group or region can help target specific research questions, providing more accurate and meaningful results.
Subsetting, on the other hand, involves creating a smaller subset of data from the original dataset. This technique is valuable when assignments involve comparing subsets or conducting separate analyses on different groups. For example, students can create subsets based on gender, ethnicity, or any other categorical variable to analyze and compare responses across these groups efficiently.
Data filtering and subsetting are not only effective for isolating relevant data but also for handling missing data. SPSS allows students to filter out missing values or create separate subsets to examine and address missing data issues separately. By managing missing data appropriately, students can ensure the robustness of their analyses and avoid biased results.
Moreover, data filtering and subsetting are particularly advantageous when dealing with longitudinal or time-series data. Students can use these techniques to select specific time intervals or time points, facilitating time-based comparisons and trend analysis.
Data merging and joins are essential techniques in statistical analysis, enabling researchers to combine data from multiple sources and perform comprehensive analyses. In SPSS assignments, the skillful application of data merging and joins can significantly enhance the depth and accuracy of the results. Here's why data merging and joins are crucial in SPSS assignments and how they contribute to providing SPSS assignment help:
Mastering data merging and joins in SPSS is essential for students seeking to excel in their statistics assignments. These techniques empower them to combine, clean, and prepare data from diverse sources, enriching the dataset and paving the way for comprehensive statistical analyses. By effectively utilizing data merging and joins, students can contribute to providing SPSS assignment help with more robust, insightful, and accurate results, ultimately elevating the quality of their academic work.
Conclusion
In conclusion, data manipulation plays a fundamental role in solving statistics assignments using SPSS. From data preparation to presentation, the effective use of SPSS's data manipulation tools ensures that statistical analyses are accurate, reliable, and well-structured. By mastering data manipulation techniques, students can efficiently tackle complex assignments, draw meaningful conclusions from data, and provide comprehensive statistics assignment help to others in need.