Avail Your Offer Now
Celebrate the festive season with an exclusive holiday treat! Enjoy 15% off on all orders at www.statisticsassignmenthelp.com this Christmas and New Year. Unlock expert guidance to boost your academic success at a discounted price. Use the code SAHHOLIDAY15 to claim your offer and start the New Year on the right note. Don’t wait—this special offer is available for a limited time only!
We Accept
- Understanding the Assignment
- Familiarize Yourself with the Data
- Set Up Your Analysis
- Conduct Preliminary Data Visualization
- Performing the Analysis
- 1. Independent Samples T-Test
- 2. One-Way ANOVA
- 3. ANCOVA (Analysis of Covariance)
- 4. Additional Considerations
- Reporting Your Findings
- Conclusion
Quantitative analysis plays an integral role in the research process, enabling scholars and professionals to derive meaningful conclusions from numerical data, which can then be used to influence policy, guide decision-making, or advance scientific understanding. Across fields like business, health sciences, public administration, and engineering, the ability to effectively analyze and interpret statistical data is a fundamental skill. Whether dealing with hypothesis testing, investigating relationships between variables, or determining statistical significance, mastering core techniques such as independent samples t-tests, one-way ANOVA, or ANCOVA is essential for producing reliable outcomes.
In statistical assignments, students are often required to not only perform complex calculations but also interpret the results within the context of their research question. A well-organized approach ensures that each step—from data preparation to conducting tests and reporting findings—follows a logical sequence, minimizing the chance for error. This blog will outline comprehensive strategies to help students approach their quantitative assignments confidently, ensuring they understand the importance of choosing the appropriate test, correctly interpreting outputs (such as p-values, confidence intervals, and effect sizes), and accurately reporting their findings in APA format. By adhering to these best practices, students can enhance both the quality of their analyses and their ability to effectively communicate the results. For those seeking additional assistance, our quantitative data analysis assignment help can provide expert guidance throughout this process. Ultimately, this structured approach will empower students to handle a wide range of quantitative assignments with greater proficiency, regardless of the specific data set or research question they encounter.
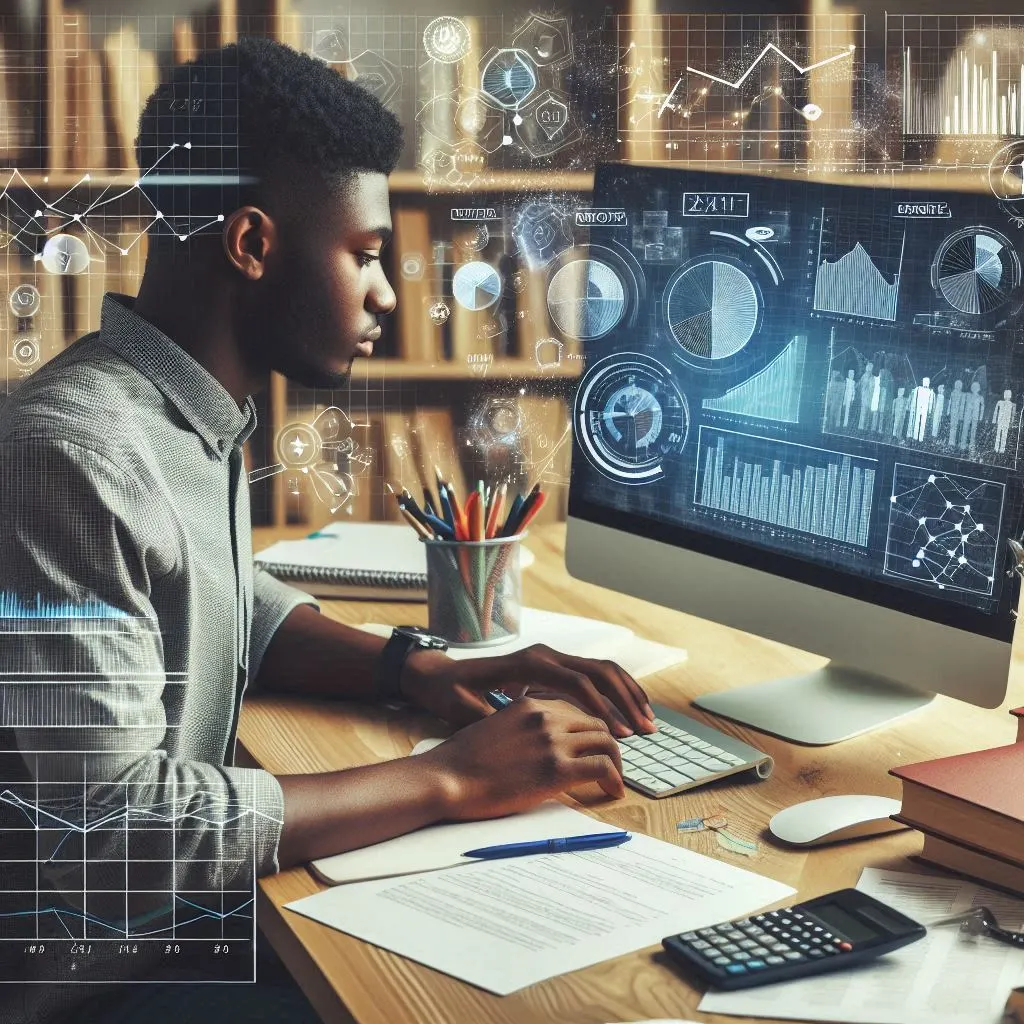
Understanding the Assignment
Before embarking on any statistical analysis, it is essential to have a thorough understanding of the assignment requirements, and the research questions you aim to address. Statistical assignments often revolve around comparing groups, testing hypotheses, and interpreting complex data to derive meaningful conclusions. As a student, it's important to approach these tasks methodically, ensuring that you not only perform the correct calculations but also understand the underlying concepts and reasoning. If you ever wondering, “How to complete my statistics assignment effectively”? Worry not,- this structured approach not only enhances the accuracy of your analysis but also improves your ability to clearly communicate the findings in your report.
Familiarize Yourself with the Data
One of the most critical initial steps in any statistical analysis is to familiarize yourself with the dataset you'll be working with. Take time to explore the data thoroughly, understanding the different variables, their types, and the relationships between them. This includes identifying whether the variables are categorical, continuous, or ordinal, and ensuring that you grasp key terms related to your research question. For example, if you're analyzing government expenditure data and county classifications, it's crucial to know the definitions of terms such as "environmental spending" and "county type" to contextualize your findings properly. Additionally, understanding the structure of your data—whether it's cross-sectional or longitudinal—will influence the types of analyses you perform.
Set Up Your Analysis
Once you are familiar with the dataset, the next step is to determine which statistical test is appropriate based on the research question at hand. This decision depends on the number of groups involved, the type of data you have, and the specific hypotheses you are testing. Below are three common statistical tests that may be applicable:
- Independent Samples T-Test: This test is used to compare the means of two independent groups to determine if there is a statistically significant difference between them. For instance, if you are tasked with examining whether coastal counties differ significantly from non-coastal counties in terms of environmental spending, the independent samples t-test would be the appropriate choice. It helps you determine whether any observed differences are likely due to random variation or represent a true difference in spending behavior.
- One-Way ANOVA (Analysis of Variance): A one-way ANOVA is suitable for comparing the means of three or more independent groups. If, for example, your assignment requires you to analyze whether the intergovernmental revenue growth rate differs across metro, suburban, and rural counties, a one-way ANOVA will allow you to assess whether there are statistically significant differences between these groups. This test is particularly useful when comparing multiple categories to see if any group stands out as significantly different.
- ANCOVA (Analysis of Covariance): ANCOVA is an extension of ANOVA that includes covariates, allowing you to control for the effects of additional continuous variables while evaluating the relationship between categorical variables and a dependent variable. For instance, if you want to examine the difference in revenue growth rates across county types while accounting for political orientation, ANCOVA would enable you to assess these relationships more accurately. By controlling for the covariate (e.g., political orientation), you can isolate the effect of county type on revenue growth.
Conduct Preliminary Data Visualization
Before conducting any formal statistical tests, it’s a good idea to visualize your data to gain preliminary insights into patterns, distributions, or group differences. Creating plots, such as error bar plots or histograms, can provide an intuitive understanding of the data and help you anticipate what you might find in your statistical tests. For example, an error bar plot comparing environmental spending between coastal and non-coastal counties can offer a visual sense of whether the means differ significantly, giving you an initial idea of what the independent samples t-test might reveal. Similarly, error bar plots for a one-way ANOVA can help highlight potential differences in revenue growth rates across multiple county types. By visualizing the data, you can also identify any potential outliers, trends, or anomalies that might affect your analysis.
Performing the Analysis
Once you've familiarized yourself with the data, set up your research framework, and visualized the key variables, it's time to conduct the statistical analysis. This step requires both a clear understanding of the chosen test and meticulous attention to detail to ensure accurate calculations. Below are some general guidelines for performing common statistical tests, as well as tips for interpreting the results:
1. Independent Samples T-Test
- Purpose: This test compares the means of two independent groups to assess whether they are statistically different from each other.
- Execution: Begin by formulating your null hypothesis (e.g., there is no significant difference in environmental spending between coastal and non-coastal counties). Run the t-test by calculating the difference in group means and dividing it by the standard error of the difference. Ensure that your data meets assumptions of normality and homogeneity of variance, as these affect the validity of your results.
- Interpreting Results: A significant p-value (typically < 0.05) indicates that the group means differ more than would be expected by chance. If the p-value is greater than 0.05, you fail to reject the null hypothesis, meaning there is no statistically significant difference.
2. One-Way ANOVA
- Purpose: This test is used to compare the means of three or more independent groups to see if at least one group mean is significantly different from the others.
- Execution: As with the t-test, start by formulating a null hypothesis (e.g., revenue growth rates are the same across metro, suburban, and rural counties). Calculate the F-statistic, which is the ratio of the variance between groups to the variance within groups. Larger F-statistics suggest greater differences between group means relative to within-group variability.
- Interpreting Results: If the p-value associated with the F-statistic is below the significance level (usually 0.05), you reject the null hypothesis, meaning that at least one group mean is significantly different. Follow up with post-hoc tests (e.g., Tukey's HSD) to determine exactly which groups differ from one another.
3. ANCOVA (Analysis of Covariance)
- Purpose: ANCOVA is used to assess the impact of categorical independent variables on a continuous dependent variable while controlling for the effects of one or more continuous covariates.
- Execution: Like ANOVA, ANCOVA starts with testing a null hypothesis, but here you also include a covariate to control for its effect. For example, if you're investigating the difference in revenue growth across county types while controlling for population size, include population size as a covariate in your model. Ensure that the relationship between the covariate and the dependent variable is linear, as nonlinearity can affect the accuracy of your results.
- Interpreting Results: The output will provide an adjusted F-statistic for the main effect (e.g., county type) after controlling for the covariate. A significant result (p-value < 0.05) indicates that the categorical variable still has an effect on the dependent variable, even after accounting for the covariate.
4. Additional Considerations
- Assumption Testing: Each of these tests relies on certain assumptions, such as normality (data should follow a normal distribution) and homogeneity of variance (equal variances across groups). Ensure you test these assumptions using diagnostics like Levene's test for equal variances or Shapiro-Wilk for normality. Violating assumptions can invalidate your results or require the use of alternative statistical methods (e.g., Welch's ANOVA for unequal variances).
- Effect Size: Beyond p-values, consider calculating the effect size (e.g., Cohen's d for t-tests, eta-squared for ANOVA). Effect size measures how large the observed differences are in practical terms, which provides more context to the significance of the findings.
By conducting these tests carefully, you will be able to quantify relationships and differences in your data with greater precision. Moreover, clear interpretation of the results will allow you to meaningfully discuss the implications of your findings.
Reporting Your Findings
Once you've completed the analysis, the final step is to effectively communicate your results. A well-structured report not only demonstrates your understanding of the analysis but also ensures that your findings are presented in a clear, concise, and professional manner. Below is a suggested structure for reporting your findings, along with guidelines for adhering to APA formatting.
1. Structure Your Report
- Abstract: Provide a brief summary of the research question, methodology, results, and conclusions.
- Introduction: Introduce the research question and the relevance of the analysis.
- Methods: Detail the statistical tests used, including assumptions and procedures.
- Results: Present the findings with tables, figures, and statistical outputs.
- Discussion: Interpret the results in the context of your research questions and literature review. Discuss implications and limitations.
- Conclusion: Summarize key findings, their significance, and potential areas for further research.
2. Follow APA Formatting
Ensure your report adheres to APA guidelines, including formatting for tables and figures, in-text citations, and reference lists.
Conclusion
Effectively analyzing quantitative data involves not only technical proficiency but also a deep understanding of the underlying research questions and the data itself. The ability to choose the appropriate statistical methods, such as t-tests, ANOVA, or ANCOVA, depends on a solid grasp of the dataset and the specific hypotheses being tested. A structured approach ensures that each step of the analysis—from data visualization and testing assumptions to conducting the statistical procedures and interpreting the results—is done meticulously and with clarity.
Beyond technical execution, the process of reporting your findings is equally important. A well-structured report helps communicate the results in a way that is accessible to a broad audience, from academic peers to decision-makers in the field. By clearly explaining your methods, presenting your results with relevant visual aids, and discussing the implications, you demonstrate both the reliability of your findings and their broader relevance.
Moreover, adhering to established reporting guidelines, such as APA formatting, not only ensures professionalism but also lends credibility to your work. Whether you are analyzing data for a research project, an academic assignment, or a professional report, the careful application of statistical tools combined with a thoughtful, methodical approach will lead to more accurate, insightful, and impactful conclusions. By mastering these elements, you can build a strong foundation for tackling a wide range of statistical assignments, enhancing both your analytical skills and your ability to communicate complex data-driven insights effectively.