Claim Your Offer
Unlock an exclusive deal at www.statisticsassignmenthelp.com with our Spring Semester Offer! Get 10% off on all statistics assignments and enjoy expert assistance at an affordable price. Our skilled team is here to provide top-quality solutions, ensuring you excel in your statistics assignments without breaking the bank. Use Offer Code: SPRINGSAH10 at checkout and grab this limited-time discount. Don’t miss the chance to save while securing the best help for your statistics assignments. Order now and make this semester a success!
We Accept
- 1. Measures of Central Tendency
- 2. Understanding Outliers
- 3. Measures of Spread
- 4. Distribution Shapes
- 5. Measures of Location
- 6. Performing Calculations in SAS
- Conclusion
Statistics is a crucial field that plays an integral role in analyzing and interpreting data effectively across various disciplines, from social sciences to natural sciences, business analytics, and healthcare. In today's data-driven world, the ability to derive meaningful insights from raw data is more important than ever. Statistics equips individuals with the tools necessary to make sense of complex information, identify trends, and inform decision-making processes. When tackling statistics assignments, it’s essential to grasp a wide array of fundamental concepts, including measures of central tendency, measures of spread, and the location of data points within a dataset.
Each of these concepts provides valuable information that contributes to a comprehensive understanding of the dataset in question. For example, measures of central tendency help us summarize data by providing single representative values, while measures of spread reveal the degree of variability and dispersion within that data. Understanding the location of data points through percentiles and z-scores allows for a clearer interpretation of individual values in relation to the overall dataset.
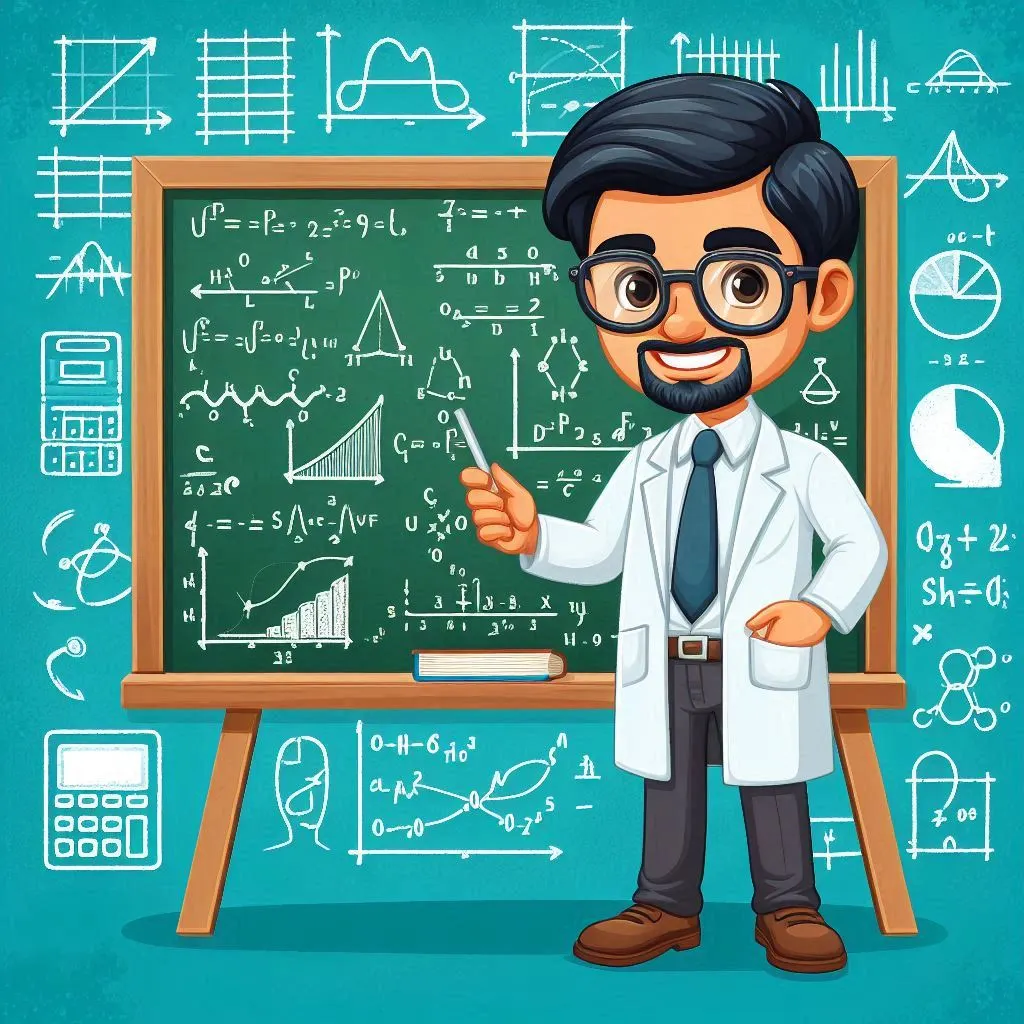
Moreover, mastering these principles enables students to engage in critical thinking and apply statistical reasoning to real-world problems, facilitating clearer communication of their findings. A solid foundation in statistics not only enhances academic performance but also prepares students for professional roles where data analysis is pivotal. This blog post will outline critical aspects of these statistical concepts, provide practical examples, and offer strategies to help you approach similar assignments with confidence and clarity. Additionally, this resource will provide help with SAS assignment, ensuring that you can effectively utilize SAS for your statistical analyses. By the end of this discussion, you will be better equipped to tackle your statistics assignments and apply these concepts in your academic and future professional endeavors.
1. Measures of Central Tendency
When analyzing data, calculating measures of central tendency is essential for summarizing and understanding the dataset. These measures include the mean, median, and mode, each offering unique insights into the data's characteristics. Seeking statistics assignment help can enhance your understanding of these concepts and improve your ability to apply them effectively in your analyses.
Mean: The arithmetic average of a data set, the mean is calculated by summing all values and dividing by the number of observations. It provides a quick snapshot of the dataset and is widely used in various fields such as economics, psychology, and the natural sciences. However, while the mean is a valuable measure, it can be significantly influenced by outliers—values that are substantially higher or lower than the rest of the data. This sensitivity makes the mean less robust in datasets with extreme values, potentially leading to misleading interpretations.
Median: The median represents the middle value in a dataset arranged in ascending or descending order. To find the median, one must identify the central number, which may require averaging the two middle values if the dataset contains an even number of observations. The median is particularly useful in situations where data is skewed or contains outliers, as it is resistant to such extreme values. Consequently, the median provides a more accurate reflection of the dataset's central tendency in non-normally distributed data.
Mode: The mode is defined as the value that appears most frequently in a dataset. A dataset can be classified as unimodal (one mode), bimodal (two modes), or multimodal (multiple modes) depending on the frequency of values. The mode is especially useful for categorical data, where it can help identify the most common category or response. However, in numerical datasets, the mode may not always be informative, particularly if all values occur with similar frequency or if there are multiple modes.
Understanding these measures of central tendency is fundamental for effective data analysis, enabling researchers and students to draw meaningful conclusions and make informed decisions based on their findings.
2. Understanding Outliers
Outliers are data points that deviate significantly from other observations within a dataset. Their presence can greatly impact statistical analyses and the conclusions drawn from data, which makes it crucial to assess whether to keep or remove outliers based on valid reasons. Understanding outliers not only helps in making informed decisions about data but also plays a vital role in improving the accuracy of your statistical interpretations.
- Natural Variability: Outliers can sometimes represent legitimate observations that reflect natural variability within the data. For instance, in a study of human heights, a very tall individual may be considered an outlier; however, they still provide a valid representation of human height diversity. In such cases, retaining these outliers can enrich your analysis and provide insights into the extremes of the data set. They can reveal trends, such as those in extreme sports or exceptional academic performance, that would otherwise be overlooked.
- Errors: Outliers may also arise from data entry errors, measurement inaccuracies, or other human errors. Identifying these outliers is crucial, as they can skew results and lead to incorrect conclusions. For example, if a participant’s weight is recorded as 500 pounds when it should be around 150, this outlier should be flagged for correction or removal to maintain data integrity. It’s essential to employ tools and techniques to detect these anomalies, such as box plots or scatter plots, which can help visualize and pinpoint problematic data points.
- Different Groups: Sometimes, an observation may belong to a different group or category than the rest of the dataset. For example, if you’re analyzing test scores of high school students and a score comes from a college student, it might skew the results and affect your overall analysis. In such cases, excluding or separately analyzing these outliers can enhance the clarity of your analysis. Additionally, considering the context of your dataset can help you determine whether an outlier should be investigated further or excluded to maintain the focus on your target population.
3. Measures of Spread
Understanding the variability in your data is crucial for a comprehensive analysis. Measures of spread help quantify the extent to which data points differ from one another. They provide insights into the distribution and characteristics of your dataset, which is essential for making informed decisions based on the data. Key measures include:
- Range: The range is the simplest measure of spread, calculated as the difference between the maximum and minimum values in your dataset. While it provides a quick overview of data dispersion, it can be influenced by outliers, which might distort the overall picture. For instance, in a dataset of exam scores, if one student scores exceptionally low due to illness, the range can give a misleading representation of the typical performance. Thus, while the range is useful, it should be supplemented with other measures of spread for a more accurate understanding.
- Standard Deviation: This measure indicates how much individual data points differ from the mean, providing insight into the typical distance of data points from the average. A smaller standard deviation suggests that the data points are closely clustered around the mean, while a larger standard deviation indicates greater variability within the dataset. Standard deviation is particularly useful in assessing consistency in data, such as the reliability of measurement tools or the stability of a process over time.
- Variance: Variance is the square of the standard deviation and serves as a measure of how spread out the data points are within a dataset. It quantifies the degree of variation and is particularly useful in statistical modeling and hypothesis testing. High variance indicates that data points are spread out over a wider range of values, which can affect the results of inferential statistics. Understanding variance helps in comparing the spread of different datasets, aiding in the selection of appropriate statistical methods.
4. Distribution Shapes
Understanding the shape of your data distribution is vital for selecting appropriate statistical methods and interpreting results accurately. Different distributions can indicate specific characteristics about the data, guiding the choice of analysis techniques and helping to predict future outcomes. Key shapes include:
- Right Skewed: In right-skewed distributions, the data has a longer tail on the right side. Typically, the mean is greater than the median, which in turn is greater than the mode (Mean > Median > Mode). This indicates that there are some higher-than-typical values pulling the mean upwards. Right skewness often occurs in datasets related to income or home prices, where a few high values can significantly impact the average. Recognizing this skewness is essential for selecting the right statistical tests, as many assume normality.
- Left Skewed: Conversely, left-skewed distributions feature a longer tail on the left side, where the mean is less than the median, which is less than the mode (Mean < Median < Mode). This suggests that lower-than-typical values are affecting the average. Left skewness can be observed in datasets like age at retirement, where most individuals retire around a certain age but a few retire significantly earlier. Identifying left skewness allows analysts to choose non-parametric tests, which are more suitable for skewed data.
- Symmetric: Symmetric distributions, such as the normal distribution, have the same value for the mean and median, indicating a balanced spread of data around a central point. In this case, if unimodal, the mode will also align with the mean and median. Symmetric distributions are foundational in statistics, as many statistical methods rely on the assumption of normality. Understanding whether your data follows a symmetric distribution helps in determining the applicability of parametric tests.
5. Measures of Location
Percentiles, quartiles, and standardized values (z-scores) are essential for understanding the position of data points within a dataset, allowing for more nuanced interpretations of the data. These measures help in comparing data points and understanding their relative standing within the dataset:
- Percentiles: A percentile is a value below which a certain percentage of the data falls. For example, the 90th percentile indicates that 90% of the data points are below that value, providing a clear view of the data's distribution. Percentiles are particularly useful in educational testing and performance metrics, allowing for comparisons across different populations or cohorts.
- Quartiles: Quartiles divide the data into four equal parts, giving insight into the data's spread and center. Specifically, Q1 (the first quartile) represents the 25th percentile, Q2 (the second quartile) is the median or 50th percentile, and Q3 (the third quartile) corresponds to the 75th percentile. Quartiles help summarize data distributions, revealing not just the central tendency but also the range of values, which can inform decision-making in fields like finance and quality control.
- Z-scores: A z-score is a standardized measure that indicates how many standard deviations a data point is from the mean.
6. Performing Calculations in SAS
To implement these statistical concepts in SAS effectively, follow these general steps to analyze your dataset and derive meaningful insights:
- Import Your Dataset: Begin by loading the necessary data files into SAS Studio. This step involves selecting the correct file formats and ensuring that your data is structured appropriately for analysis. You can use the PROC IMPORT procedure to facilitate the data import process, making sure that data types are correctly assigned.
- Calculate Summary Statistics: Utilize procedures like PROC MEANS to compute essential statistics such as the mean, median, standard deviation, and range. This step will provide a foundational understanding of your data and allow for initial assessments of central tendency and spread. You can also use PROC UNIVARIATE for more detailed statistics and visualizations of your data distribution.
- Identify and Handle Outliers: Employ statistical methods, such as box plots or the interquartile range (IQR) method, to detect outliers. The IQR method calculates the first (Q1) and third quartiles (Q3) to establish the IQR (Q3 - Q1), identifying values that lie outside the range of Q1 - 1.5 * IQR to Q3 + 1.5 * IQR. Make informed decisions on whether to include or exclude these values based on their impact on your analysis.
- Visualize Data Distribution: Create visual representations of your data, such as histograms, box plots, or scatter plots, to help illustrate the shape of your data distribution. Visualizations can provide immediate insights into the characteristics of your dataset and help identify patterns, trends, and potential anomalies.
- Generate Reports: Compile your results and findings in a clear and professional format. Use Output Delivery System (ODS) statements in SAS to produce well-structured reports that effectively communicate your analysis to your audience. Tailor your reports to meet the needs of your stakeholders, emphasizing key findings and recommendations based on the statistical analyses conducted.
Conclusion
In conclusion, mastering statistical concepts is vital for effectively analyzing and interpreting data. By understanding measures of central tendency, variability, outliers, distribution shapes, and measures of location, you can develop a robust framework for approaching statistics assignments. These concepts not only enhance your analytical skills but also empower you to make informed decisions based on data.
As you navigate through your statistics assignments, remember that practice and familiarity with tools like SAS can significantly streamline your analytical processes. By implementing the techniques discussed in this blog, you will be well-equipped to tackle complex data analyses with confidence and precision. Embrace the power of statistics, and let it guide your understanding of the world through data-driven insights. Whether you're a student, researcher, or professional, the ability to analyze and interpret data effectively will be an invaluable asset in today’s data-centric landscape.