Avail Your Offer Now
Celebrate the festive season with an exclusive holiday treat! Enjoy 15% off on all orders at www.statisticsassignmenthelp.com this Christmas and New Year. Unlock expert guidance to boost your academic success at a discounted price. Use the code SAHHOLIDAY15 to claim your offer and start the New Year on the right note. Don’t wait—this special offer is available for a limited time only!
We Accept
- Understanding T-tests
- 1. Identify the Type of T-test:
- 2. Check Assumptions:
- 3. Formulate Hypotheses:
- 4. Calculate and Interpret Results:
- Exploring ANOVA
- 1. Choose the Right Type of ANOVA:
- 2. Check Assumptions:
- 3. Create Hypotheses:
- 4. Analyze Results:
- 5. Post-Hoc Tests:
- Practical Tips for Solving Assignments
- 1. Read Instructions Carefully:
- 2. Show Your Work:
- 3. Use Software Wisely:
- 4. Interpret Results Carefully:
- 5. Review and Revise:
- Conclusion
- Key Takeaways:
- Additional Support:
Statistical analysis is an essential skill for interpreting data and drawing meaningful conclusions across various fields of study. Whether you're involved in scientific research, evaluating business performance, or analyzing social trends, understanding statistical methods is crucial for deriving actionable insights from complex datasets. This blog aims to explore how to approach assignments involving T-tests and ANOVA—two foundational techniques in statistics that are widely employed for hypothesis testing and data analysis. These methods are instrumental in determining whether observed differences or relationships are statistically significant or if they might have occurred by chance.
While each statistical assignment may present unique scenarios or specific requirements, the core principles of T-tests and ANOVA remain fundamentally the same. Grasping these concepts allows you to effectively assess experimental results, make informed decisions, and validate research hypotheses. In this blog, we will delve into the practical application of these statistical tests, covering essential steps such as formulating hypotheses, checking assumptions, and interpreting results. Additionally, we will highlight common pitfalls and best practices to ensure accurate and reliable analysis. By mastering these techniques, you can enhance your analytical capabilities, contribute valuable insights to your field of study, and approach your assignments with greater confidence and precision. For those seeking additional support, our ANOVA assignment helper is available to guide you through complex scenarios and ensure accurate results.
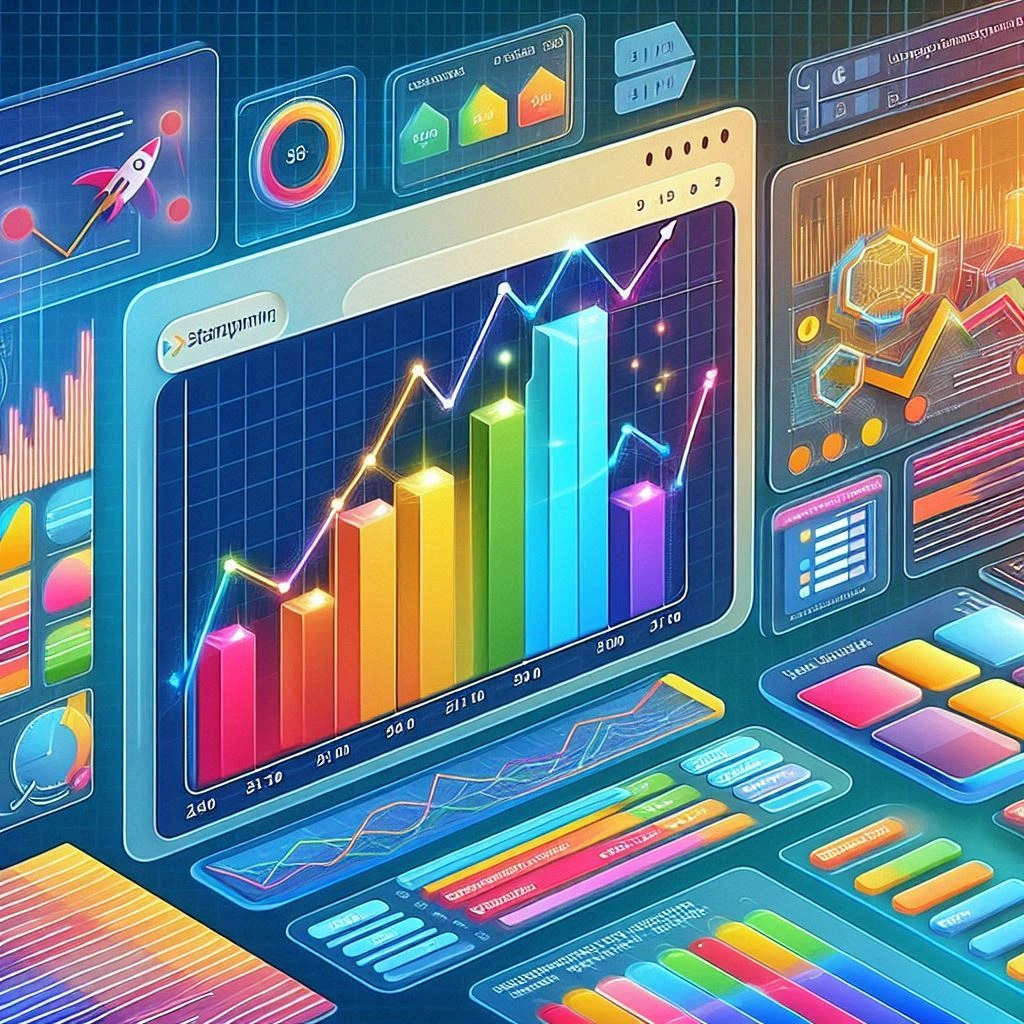
Understanding T-tests
T-tests are a fundamental statistical tool used to compare the means of two groups and determine whether any observed differences are statistically significant. These tests are essential in various research fields, from medical studies to market research, where understanding the impact of interventions or differences between groups is crucial. Mastery of T-tests allows researchers to make informed decisions based on empirical data, ensuring that conclusions drawn from experiments or surveys are reliable and valid. If you ever wondered. “How to complete my Statistics assignment”? or need assistance with your statistical tasks, including guidance on T-tests, consider reaching out to a service that can help you complete your statistics assignment efficiently and accurately.
Here’s a closer look at how to approach T-test questions effectively:
1. Identify the Type of T-test:
- Independent T-test: This test is employed when comparing the means from two separate groups. For instance, it can be used to assess the impact of a new teaching method by comparing test scores between two different classes.
- Paired T-test: Used for comparing means from the same group at different times or under different conditions. For example, it can evaluate the effectiveness of a treatment by comparing patients' health metrics before and after treatment.
- One-sample T-test: This test compares the mean of a single group to a known value or population mean. It’s useful when you want to assess whether a sample mean differs from a theoretical value or benchmark.
2. Check Assumptions:
- Normal Distribution: The data should follow a normal distribution. While some T-tests are robust to deviations from normality, significant deviations may affect the validity of the test.
- Equal Variances: Assumes that the variances of the two groups being compared are equal. This assumption is crucial for the independent T-test; if variances are unequal, adjustments or alternative tests might be needed.
- Independence of Observations: Each observation should be independent of others. This means that the data points in one group should not influence or be related to those in another group.
3. Formulate Hypotheses:
- Null Hypothesis (H0): States that there is no effect or no difference between the groups. For instance, it might claim that a new teaching method has no effect on students’ test scores compared to the traditional method.
- Alternative Hypothesis (H1): Suggests that there is an effect or a difference between the groups. In the previous example, it would propose that the new teaching method does have an impact on students' scores.
4. Calculate and Interpret Results:
- Compute the Test Statistic: Use the appropriate T-test formula to calculate the test statistic, which helps in assessing the magnitude of the difference between group means relative to the variability within the groups.
- Compare the p-value: The p-value indicates the probability of observing the data, or something more extreme, under the null hypothesis. Compare this p-value to your significance level (typically 0.05) to determine whether to reject the null hypothesis. A p-value less than 0.05 generally means that the observed differences are statistically significant.
By following these steps, you can systematically approach T-test questions and ensure that your analyses are thorough and reliable. Whether you're comparing the effectiveness of different treatments or assessing the impact of a new strategy, a solid understanding of T-tests will help you draw meaningful conclusions from your data.
Exploring ANOVA
ANOVA (Analysis of Variance) is a statistical technique used to compare the means of three or more groups to determine if there are significant differences among them. It is a powerful tool for analyzing data when you have multiple groups and want to understand how they differ in relation to one or more factors. ANOVA helps you to test hypotheses about the equality of group means and is widely used in various fields such as psychology, medicine, and social sciences.
Here’s a detailed approach to tackling ANOVA assignments:
1. Choose the Right Type of ANOVA:
- One-Way ANOVA: This method is used to compare the means of three or more groups based on a single factor or variable. For example, it can be used to compare the average test scores of students across different teaching methods. One-Way ANOVA helps determine whether any of the group means significantly differ from each other.
- Two-Way ANOVA: This technique extends the One-Way ANOVA by including two factors and assessing their individual and interactive effects on the dependent variable. For instance, you might use Two-Way ANOVA to evaluate how both teaching methods and student backgrounds (e.g., educational level) affect test scores, and whether there is an interaction between these two factors.
2. Check Assumptions:
- Normal Distribution: The data within each group should be approximately normally distributed. While ANOVA is robust to some deviations from normality, severe violations can affect the validity of the results.
- Equal Variances: Assumes that the variances among the groups are equal. This is crucial for the accuracy of ANOVA results. If variances are unequal, alternative methods such as Welch’s ANOVA or data transformations may be needed.
- Independence of Observations: Each observation should be independent of the others. This ensures that the data points in each group are not influenced by those in other groups.
3. Create Hypotheses:
- Hypothesis (H0): States that all group means are equal, implying that any observed differences are due to random chance. For example, if comparing test scores among different teaching methods, the null hypothesis would be that the mean scores for all teaching methods are the same.
- Alternative Hypothesis (H1): Proposes that at least one group mean is different from the others. This indicates that there is a statistically significant difference between at least two of the groups.
4. Analyze Results:
- F-statistic and p-value: Examine the F-statistic and the corresponding p-value from the ANOVA table. The F-statistic measures the ratio of between-group variance to within-group variance. A higher F-statistic suggests a greater likelihood that the group means are different. The p-value helps determine the statistical significance of this result.
- Significance Level: Compare the p-value to your chosen significance level (usually 0.05). If the p-value is less than 0.05, reject the null hypothesis, indicating that there are significant differences among the group means.
5. Post-Hoc Tests:
- Conduct Post-Hoc Tests: If the ANOVA indicates significant differences, perform post-hoc tests to identify which specific groups differ from each other. Common post-hoc tests include Tukey's Honestly Significant Difference (HSD), Bonferroni correction, and Scheffé's test. These tests help pinpoint which pairs of groups show significant differences.
By following these steps, you can effectively approach ANOVA assignments and ensure a comprehensive analysis of your data. Whether comparing different treatments, evaluating the impact of various conditions, or exploring interactions between multiple factors, a solid understanding of ANOVA will help you draw accurate and meaningful conclusions from your research.
Practical Tips for Solving Assignments
When tackling statistical assignments, precision and clarity are paramount. Statistical analysis often involves complex calculations and nuanced interpretations, and adhering to best practices can make a significant difference in your results and overall grade. Whether you are working on T-tests, ANOVA, or any other statistical method, following a systematic approach will enhance your accuracy and efficiency. Here are some practical tips to guide you through solving statistical assignments effectively:
1. Read Instructions Carefully:
- Understand the Requirements: Begin by thoroughly reviewing the assignment instructions to ensure you grasp the specific statistical test required. Misinterpreting the type of analysis needed can lead to incorrect conclusions and lower grades.
- Check Assumptions: Each statistical test comes with its own set of assumptions that must be met for the results to be valid. Make sure you understand these assumptions and verify that your data meets them before proceeding with the analysis.
2. Show Your Work:
- Document Each Step: Provide a detailed account of each step involved in your calculations. This not only helps in earning full credit but also makes it easier for you or anyone reviewing your work to follow your thought process and identify any potential errors.
- Explain Your Reasoning: Clearly explain the rationale behind each decision you make during the analysis. For instance, if you choose a particular type of T-test or ANOVA, provide a brief explanation of why that choice is appropriate for your data and research question.
3. Use Software Wisely:
- Leverage Statistical Tools: Tools like Excel, R, SPSS, or other statistical software can significantly aid in performing complex calculations and generating results. They can save time and reduce the potential for manual errors.
- Verify Results Manually: Despite the convenience of software, always double-check your results manually when possible. This practice helps ensure that the software hasn’t introduced any errors and reinforces your understanding of the analysis process.
4. Interpret Results Carefully:
- Contextualize Findings: When interpreting results, always relate them back to the research question or hypothesis. Understand what the statistical findings mean in the context of your study and be able to explain their practical significance.
- Consider Significance Levels: Be mindful of the significance level you are using (usually 0.05) and how it impacts the interpretation of your p-values. This helps in making informed decisions about whether to reject the null hypothesis.
5. Review and Revise:
- Check for Consistency: Review your work to ensure that your calculations, interpretations, and conclusions are consistent and logical. Look for any discrepancies or errors and correct them before finalizing your assignment.
- Seek Feedback: If possible, get feedback from peers or instructors on your approach and findings. They can provide valuable insights and help you refine your analysis.
By incorporating these practical tips into your approach, you can enhance the quality and accuracy of your statistical assignments. Effective problem-solving in statistics requires not only technical skills but also attention to detail and a thorough understanding of the analytical methods you are using.
Conclusion
Mastering T-tests and ANOVA is essential for effectively analyzing and interpreting statistical data. These methods are powerful tools for comparing group means and uncovering significant differences, which can inform your conclusions and drive data-driven decision-making. By understanding their purposes and applications, you can confidently approach a wide range of statistical assignments and research questions.
Key Takeaways:
- Purpose and Application: Recognize the specific scenarios where T-tests and ANOVA are applicable. T-tests are ideal for comparing two groups, while ANOVA is designed for analyzing differences among three or more groups. Understanding these contexts helps in selecting the appropriate test for your data.
- Assumptions and Validity: Always check the assumptions underlying each test, such as normality, homogeneity of variance, and independence of observations. Valid results depend on meeting these assumptions, so ensure your data complies with them before proceeding.
- Methodology and Documentation: Clarity in your methodology is crucial. Document each step of your analysis meticulously, from hypothesis formulation to result interpretation. This transparency not only earns full credit but also facilitates a deeper understanding of the analytical process.
- Software and Manual Verification: While statistical software can streamline calculations, manual verification is important to ensure accuracy. Use software as a tool to assist in calculations but rely on manual checks to confirm results and reinforce your comprehension of the statistical concepts.
- Interpretation and Context: Effectively interpret your results in the context of your research question or hypothesis. Understand what your findings mean in practical terms and how they contribute to your overall analysis.
Additional Support:
If you find yourself needing further assistance or resources, don’t hesitate to seek help. Academic support is available in various forms, including tutoring services, online resources, and study groups. Leveraging these resources can provide you with additional insights and enhance your understanding of statistical analysis.
In summary, approaching T-tests and ANOVA with a clear methodology, rigorous attention to detail, and a strong understanding of their applications will help you excel in statistical assignments. With these practices, you can confidently analyze data, draw meaningful conclusions, and achieve success in your academic endeavors.