Avail Your Offer Now
Celebrate the festive season with an exclusive holiday treat! Enjoy 15% off on all orders at www.statisticsassignmenthelp.com this Christmas and New Year. Unlock expert guidance to boost your academic success at a discounted price. Use the code SAHHOLIDAY15 to claim your offer and start the New Year on the right note. Don’t wait—this special offer is available for a limited time only!
We Accept
- Understanding the Basics of Hypothesis Testing
- Population, Sample, and Variables
- Null and Alternative Hypotheses
- Choosing the Right Test for the Job
- Z-Tests and T-Tests
- Chi-Square Tests
- Key Concepts in Hypothesis Testing
- Significance Level (Alpha)
- P-Value
- Type I and Type II Errors
- Strategies for Solving Hypothesis Testing Assignments
- Define Your Hypotheses Clearly
- Choose the Appropriate Test
- Collect and Analyze Data
- Calculate the Test Statistic
- Calculate the P-Value
- Compare P-Value with Significance Level
- Interpret Results
- Tips for Success and Avoiding Common Pitfalls
- Conclusion
Understanding the Basics of Hypothesis Testing
Before delving into hypothesis testing intricacies, grasp the essentials. Recognize the difference between population and sample, categorical and continuous variables. Comprehend the significance of null and alternative hypotheses as foundations of the process. These foundational concepts form the bedrock upon which effective hypothesis testing is built. The fundamental concepts include:
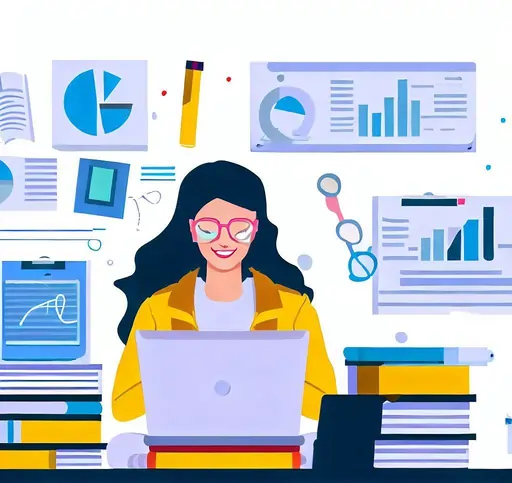
Population, Sample, and Variables
In the realm of hypothesis testing, a solid understanding of population, sample, and variables is crucial. A population represents the entire group under study, while a sample is a subset taken from that population. This distinction is pivotal as hypothesis testing involves drawing conclusions about populations based on sample data.
Moreover, variables play a pivotal role. Categorical variables divide data into distinct categories, like colors or gender. On the other hand, continuous variables encompass a range of values and allow for precise measurements, such as height or temperature. Distinguishing between these types is vital, as different hypothesis tests are designed for specific variable types.
In essence, comprehending populations, samples, and variables lays the groundwork for conducting hypothesis testing accurately. A misinterpretation of these concepts can lead to erroneous conclusions, highlighting their significance in any statistical analysis.
Null and Alternative Hypotheses
In the realm of hypothesis testing, null and alternative hypotheses serve as the guiding principles. The null hypothesis suggests that there is no significant difference or effect in the observed data. On the other hand, the alternative hypothesis (Ha) proposes the opposite: there is a meaningful difference or effect present. These two hypotheses create a dichotomy that guides the entire testing process.
Understanding their interplay is vital. Researchers typically seek evidence to reject the null hypothesis in favor of the alternative. The decision-making hinges on statistical tests and the comparison of calculated values with predetermined significance levels. This fundamental understanding allows you to interpret the outcomes of hypothesis tests more effectively, enabling you to draw meaningful conclusions from the data and make informed decisions that can have significant implications in various fields, from medicine to economics.
Choosing the Right Test for the Job
Selecting the appropriate hypothesis test is pivotal for accurate conclusions. Z-tests and T-tests cater to varying sample sizes and knowledge of population standard deviation. Chi-Square tests are tailored for categorical data. Knowing which test aligns with your data characteristics is essential. Making this informed decision ensures your analysis is robust and your results are trustworthy. Different scenarios call for different hypothesis tests.
Z-Tests and T-Tests
When it comes to comparing sample means with population parameters, Z-tests and T-tests are invaluable tools. Z-tests are advantageous when dealing with a large sample size and when the population standard deviation is known. Conversely, T-tests are preferred for smaller samples or instances where the population standard deviation is unknown.
Z-tests utilize the standard normal distribution for calculations, while T-tests employ the Student's t-distribution, which accounts for the uncertainty introduced by the smaller sample size. Both tests yield a test statistic that is then compared to critical values to determine statistical significance. The choice between these tests hinges on the characteristics of your data and the assumptions you can make.
Mastering the nuances of these tests equips you with the skills to accurately assess hypotheses, providing insights that underpin sound decision-making. Whether in scientific research or business analytics, understanding when and how to use Z-tests and T-tests ensures your statistical analyses stand on a solid foundation.
Chi-Square Tests
When confronted with categorical data, Chi-Square tests emerge as a crucial tool. By comparing observed frequencies with expected frequencies, Chi-Square tests reveal whether the observed deviations are due to chance or suggest a genuine relationship.
This method is employed in various contexts, such as survey responses, genetics, and market research. It aids in investigating patterns and dependencies within categorical data sets. Understanding the mechanics of Chi-Square tests empowers you to analyze data where numerical measurements are impractical or irrelevant. A clear grasp of this test helps you unveil hidden insights within categorical variables, making it an indispensable asset for hypothesis testing assignments and real-world applications alike.
Key Concepts in Hypothesis Testing
Significance level (alpha), p-value, and Type I and Type II errors are pivotal concepts in hypothesis testing. Understanding Type I and Type II errors aids in interpreting test outcomes accurately. Proficiency in these concepts ensures sound decision-making in hypothesis testing scenarios.
Significance Level (Alpha)
The significance level, denoted as alpha (α), plays a critical role in hypothesis testing. It determines the probability of committing a Type I error, which involves incorrectly rejecting a true null hypothesis. Selecting an appropriate significance level is a balance between being cautious of false positives and detecting genuine effects. Commonly set at 0.05 or 0.01, alpha influences the stringency of evidence required to reject the null hypothesis. A lower alpha demands stronger evidence, reducing the likelihood of drawing false conclusions. Conversely, a higher alpha makes it easier to reject the null, increasing the chance of Type I errors. Understanding the significance level and its impact empowers researchers to make informed decisions about hypothesis testing outcomes and the credibility of their findings.
P-Value
The p-value is a vital statistical metric that holds the power to sway hypotheses. It quantifies the evidence against the null hypothesis by indicating the probability of observing results as extreme as the ones obtained, assuming the null hypothesis is true. A smaller p-value suggests stronger evidence against the null hypothesis, potentially leading to its rejection in favor of the alternative.
Interpreting p-values demands a nuanced approach. Comparing the p-value to the chosen significance level helps make informed decisions. If the p-value is less than the significance level (α), the evidence is deemed significant, prompting rejection of the null hypothesis. Conversely, a larger p-value suggests a lack of compelling evidence to reject the null hypothesis. A solid understanding of p-values ensures you can accurately evaluate the strength of your findings and draw valid conclusions in hypothesis testing scenarios.
Type I and Type II Errors
Navigating the world of hypothesis testing demands a firm grasp of Type I and Type II errors. Type I error occurs when you mistakenly reject a true null hypothesis, essentially seeing an effect that doesn't exist. On the other hand, Type II error involves failing to reject a false null hypothesis, missing a real effect.
Balancing these errors is crucial. Lowering the significance level (α) to minimize Type I errors might increase the risk of Type II errors, and vice versa. By understanding the trade-offs and implications of these errors, you can make informed decisions about the appropriate significance level for your analysis. This nuanced comprehension safeguards against drawing incorrect conclusions, equipping you with the skills needed to conduct hypothesis tests meticulously and interpret their outcomes accurately.
Strategies for Solving Hypothesis Testing Assignments
Approaching hypothesis testing assignments requires a systematic approach. Clearly defining hypotheses, choosing the suitable test, collecting and analyzing data meticulously, and calculating the test statistic and p-value are integral steps. Comparing the p-value with the significance level and providing a well-rounded interpretation of results ensures a comprehensive and accurate analysis, enhancing your assignment's quality.
Define Your Hypotheses Clearly
Articulating your null and alternative hypotheses with precision is the foundational step in hypothesis testing. Clear hypotheses guide your entire analysis, helping you remain focused and avoid ambiguity. Well-defined hypotheses also assist readers in understanding your research goals. Whether you're exploring a new drug's efficacy or the impact of a marketing campaign, a succinct and unambiguous statement of hypotheses lays the groundwork for a coherent analysis, making your assignment more organized and your conclusions more robust.
Choose the Appropriate Test
Selecting the right hypothesis test is akin to using the right tool for a task. Z-tests and T-tests cater to different sample sizes and information availability. Chi-Square tests are tailored for categorical data analysis. Opting for the appropriate test hinges on the nature of your data and the research question at hand. A well-chosen test not only ensures accurate results but also showcases your understanding of the data's characteristics and the statistical methods best suited to address your research objectives.
Collect and Analyze Data
Gathering accurate and relevant data forms the bedrock of hypothesis testing. Ensure your data collection method aligns with your research question. After data acquisition, meticulous analysis is imperative. Calculate descriptive statistics, such as means and standard deviations, to understand data distribution. For categorical data, construct contingency tables. This step not only aids in choosing the appropriate test but also provides insights into potential trends or patterns. A thorough analysis guarantees the validity of your conclusions, reinforcing the significance of data integrity in hypothesis testing assignments.
Calculate the Test Statistic
Calculating the test statistic is a pivotal step in hypothesis testing. This statistic summarizes the data's behavior in relation to the null hypothesis and helps quantify the evidence for or against it. It serves as the basis for making informed decisions. The choice of test statistic depends on the hypothesis test being used and the nature of the data. A correctly calculated test statistic ensures the accuracy and reliability of your analysis, enabling you to draw valid conclusions and contribute meaningfully to the field of study.
Calculate the P-Value
Calculating the p-value is a pivotal step in hypothesis testing. By comparing the p-value to the chosen significance level (α), usually 0.05, you determine whether to reject or fail to reject the null hypothesis. Accurate calculation of the p-value demands a solid understanding of statistical distributions and the specific test being employed. It's the bridge that connects your data analysis to the ultimate conclusion, making mastering this process essential for confident hypothesis testing.
Compare P-Value with Significance Level
When evaluating the results of a hypothesis test, comparing the calculated p-value with the chosen significance level (α) is pivotal. If the p-value is lower than α, you have substantial evidence to reject the null hypothesis. Conversely, if the p-value surpasses α, you lack sufficient grounds to reject it. This step ensures a well-informed decision-making process and guards against rash conclusions. Precision in this comparison is vital, as it dictates whether your findings are statistically significant, thereby steering the direction of your analysis and contributing to robust conclusions.
Interpret Results
Interpreting hypothesis testing results goes beyond numbers. It involves translating statistical outcomes into meaningful insights. Explain the implications of rejecting or failing to reject the null hypothesis in the context of your study. Address the practical significance of your findings. Effective interpretation showcases your understanding of the real-world implications of the statistical analysis, ensuring that your audience comprehends the broader implications of your work and the potential actions that might stem from your conclusions.
Tips for Success and Avoiding Common Pitfalls
Here are a few additional tips to excel in hypothesis testing assignments
- Practice regularly:Work through various examples and problems to reinforce your understanding.
- Double-Check Assumptions:Hypothesis tests often come with assumptions about the data distribution and other factors. Ensure these assumptions are met before proceeding.
- Visualize Your Data:Creating graphs and plots can help you better understand your data and identify any trends or outliers that might influence your analysis.
- Seek Help When Needed:Don't hesitate to reach out to your instructor, peers, or online resources if you're struggling with a particular concept or problem.
Conclusion
Embarking on an assignment involving hypothesis testing might initially seem daunting, but with a solid foundation in key concepts and a strategic approach to problem-solving, you'll be well-equipped to tackle any challenge. Remember to grasp the basics, choose the right test, understand significance levels and p-values, and apply effective strategies for solving hypothesis testing problems. By investing time and effort into mastering these concepts, you'll not only excel in your assignments but also develop a valuable skillset for making informed decisions based on data in various real-world scenarios.