Claim Your Offer
Unlock a fantastic deal at www.statisticsassignmenthelp.com with our latest offer. Get an incredible 10% off on all statistics assignment, ensuring quality help at a cheap price. Our expert team is ready to assist you, making your academic journey smoother and more affordable. Don't miss out on this opportunity to enhance your skills and save on your studies. Take advantage of our offer now and secure top-notch help for your statistics assignments.
We Accept
- Formulating Hypotheses
- Hypothesis Formation:
- Choosing the Analysis Method:
- Evaluating Assumptions
- Multicollinearity:
- Residuals Analysis:
- Outliers Detection:
- Results Interpretation
- Identifying Key Predictors:
- Writing the Results Section:
- Conclusion
When faced with multiple regression and correlation assignments, having a well-defined strategy is crucial for effective data analysis and interpretation. A systematic approach not only ensures that you follow a logical process but also helps you to thoroughly understand the relationships between variables and the underlying statistical principles. By adopting a clear framework, you can approach your assignment with greater clarity and purpose, minimizing errors and maximizing the reliability of your findings. This structured method allows you to dissect complex data into manageable parts, ensuring that each component of your analysis is addressed comprehensively.
This blog offers a comprehensive and detailed framework designed to guide you through the complexities of such assignments. It provides step-by-step instructions that cover all essential aspects, from formulating and testing hypotheses to evaluating assumptions and interpreting results. This structured approach is intended to demystify the process, allowing you to tackle each component with confidence and precision. By adhering to this method, you will be better equipped to handle the intricacies of multiple regression and correlation analysis, leading to more accurate, insightful, and meaningful conclusions.
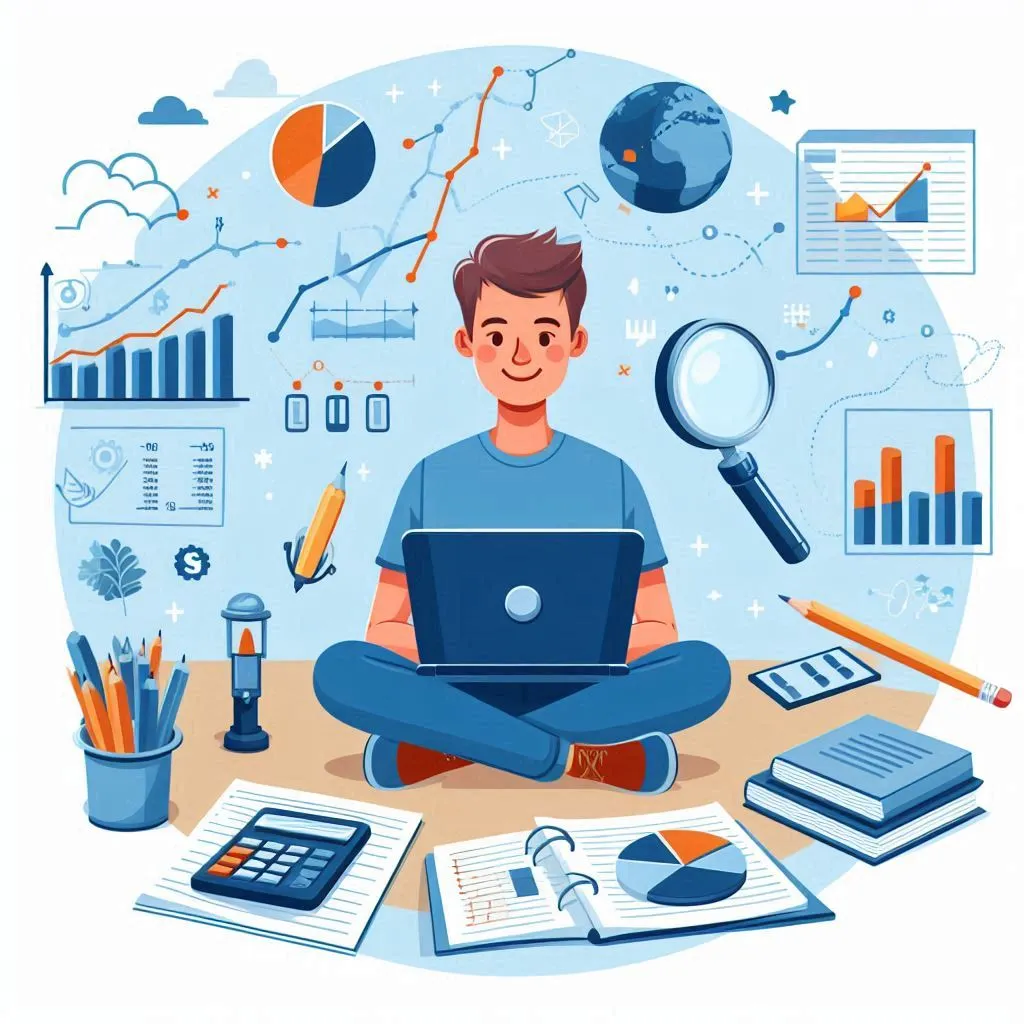
In addition, this blog aims to bridge the gap between theoretical knowledge and practical application, offering insights that will help you understand how to apply statistical concepts to real-world scenarios. Whether you are a novice seeking to build a strong foundation or an experienced student looking to refine your analytical skills, this guide is designed to enhance your understanding and ability to effectively solve a range of statistical problems. By following this approach, you will develop a robust analytical toolkit that will serve you well in your academic and professional endeavors. For those struggling with complex analyses, multiple regression assignment help can provide the support needed to master these techniques and achieve academic success.
Formulating Hypotheses
When starting a multiple regression or correlation analysis, the first critical step is to formulate clear and testable hypotheses. This step lays the groundwork for your entire analysis and ensures that you are focused on addressing specific research questions. If you find this process challenging, seeking statistics assignment help can provide expert guidance in developing robust hypotheses and conducting a thorough analysis to achieve accurate and meaningful results.
Hypothesis Formation:
Formulating hypotheses involves clearly defining what you expect to discover through your analysis. For multiple regression analysis, you generally start with two types of hypotheses:
- Null Hypothesis (H₀): This hypothesis assumes that there is no significant relationship between the predictors (independent variables) and the outcome variable (dependent variable). In other words, the null hypothesis posits that the predictors do not contribute to explaining the variance in the outcome variable beyond what could be expected by chance.
- Alternative Hypothesis (H₁): Conversely, the alternative hypothesis suggests that there is a significant relationship between the predictors and the outcome variable. It indicates that the predictors do have an effect on the outcome variable and that this effect is unlikely to be due to random chance.
These hypotheses provide a foundation for testing whether the predictors collectively or individually contribute to explaining variations in the outcome variable.
Choosing the Analysis Method:
The choice between multiple regression and multiple correlation depends on the nature of your research question and the type of relationship you wish to explore:
- Multiple Regression Analysis: This method is used when you aim to predict the value of a dependent variable from multiple independent variables. It allows you to assess the individual contribution of each predictor, control for the effects of other predictors, and develop a prediction equation. Multiple regression is ideal when you want to understand the combined effect of several variables on an outcome and when you need to make predictions based on those variables.
- Multiple Correlation Analysis: This approach is used when you want to explore the strength and direction of the relationship between a set of predictors and a single outcome variable. It measures how well the set of predictors, taken together, correlates with the outcome variable. Multiple correlation is suitable for examining the overall association between predictors and an outcome, but it does not provide a predictive model like multiple regression.
Understanding the distinction between these methods helps you select the appropriate approach for your analysis and ensures that you can accurately address your research question.
Evaluating Assumptions
In multiple regression and correlation analysis, verifying certain assumptions is crucial for ensuring the validity and reliability of your results. Properly evaluating these assumptions helps you avoid common pitfalls and ensures that your analysis provides accurate and meaningful insights.
Multicollinearity:
Multicollinearity occurs when predictors in your model are highly correlated with each other, which can lead to unstable estimates of regression coefficients and make it difficult to determine the individual effect of each predictor. High multicollinearity can inflate the variance of coefficient estimates and lead to misleading conclusions.
To assess multicollinearity, you can use:
- Variance Inflation Factor (VIF): VIF quantifies how much the variance of an estimated regression coefficient increases due to multicollinearity. A VIF value greater than 10 (or in some cases, 5) typically indicates problematic multicollinearity.
- Tolerance Values: Tolerance is the inverse of VIF and provides a measure of how much of the variance of a predictor is not explained by other predictors. Low tolerance values (below 0.1) suggest high multicollinearity.
By checking these metrics, you can identify if multicollinearity is affecting your model and take appropriate steps to address it, such as removing or combining predictors.
Residuals Analysis:
Residuals are the differences between the observed values and the values predicted by your model. Analyzing residuals helps in evaluating how well your model fits the data and whether the assumptions of linear regression are met.
- Normality of Residuals: Residuals should be normally distributed for reliable hypothesis testing. You can use visual inspections, such as Q-Q plots, or statistical tests like the Shapiro-Wilk test to assess normality.
- Residuals vs. Predicted Values Plot: Plotting residuals against predicted values helps to identify patterns. Ideally, residuals should be randomly scattered around zero without forming any specific patterns. Systematic patterns might indicate model inadequacies, such as non-linearity.
Outliers Detection:
Outliers are data points that differ significantly from other observations and can disproportionately influence the results of your analysis. Identifying and addressing outliers is essential to ensure that they do not skew your results.
- Diagnostic Plots: Leverage plots such as leverage vs. residuals, and Cook's distance plots to identify outliers and influential data points. High leverage points are those that have extreme predictor values, while influential points are those that significantly affect the regression model's estimates.
- Influence Statistics: Cook's distance, DFBETAS, and DFFITS are commonly used statistics to detect influential observations. High values in these measures suggest that the data point has a considerable impact on the regression results.
By carefully evaluating these assumptions, you can ensure that your regression or correlation analysis is robust and that the conclusions drawn from your model are valid and reliable.
Results Interpretation
After completing your multiple regression or correlation analysis, the next critical step is interpreting the results to draw meaningful conclusions from your data. This involves not only identifying which predictors are most influential but also presenting your findings in a clear and professional manner. Effective interpretation is crucial as it helps in understanding the practical implications of your analysis and communicating your results in a way that is both accessible and impactful.
Identifying Key Predictors:
Once your analysis is complete, the next step is to interpret the results and identify which predictors are most influential. This involves examining the standardized coefficients and significance levels:
- Standardized Coefficients: These coefficients, often denoted as beta weights, are essential for comparing the relative importance of each predictor in your model. They are scaled to have a mean of zero and a standard deviation of one, allowing for straightforward comparison across different predictors. Larger absolute values of standardized coefficients indicate a greater influence on the outcome variable. Understanding these coefficients helps to pinpoint which predictors have the most significant effect and thus play a crucial role in the outcomes you are investigating.
- Significance Levels: Assess the p-values associated with each predictor to determine whether their effects are statistically significant. Predictors with p-values below a commonly used threshold (e.g., 0.05) are considered significant contributors to the outcome variable. This evaluation helps to distinguish between predictors that have a meaningful impact on the outcome and those that do not, allowing you to focus on the most important factors in your analysis.
By focusing on predictors with both large standardized coefficients and significant p-values, you can identify which variables have the most substantial role in predicting the outcome. This process aids in understanding the key drivers of your results and highlights which factors are most critical for achieving your research objectives.
Writing the Results Section:
Crafting a results section involves summarizing and presenting your findings in a clear, comprehensive, and concise manner. This section should provide a detailed overview of your analysis and its implications. Follow these guidelines to ensure that your results are communicated effectively:
- Summary of Findings: Start with a succinct summary of the main results from your regression analysis. Highlight key statistics such as the R-squared value, which indicates the proportion of variance in the outcome variable explained by the model, and significant predictors. This summary provides a snapshot of your findings and helps to set the stage for a deeper discussion.
- APA-Style Table: Incorporate an APA-style table to present your regression results clearly. The table should include essential statistics such as coefficients, standard errors, t-values, p-values, and other relevant metrics. Proper formatting according to APA guidelines ensures that the table is professional and easy to understand. A well-structured table not only presents your results but also enhances the readability and credibility of your report.
- Interpretation of Results: Following the table, interpret the findings comprehensively. Discuss the significance of each predictor, the overall fit of the model, and any notable patterns or anomalies observed in the data. Explain how the results address your research question and their implications for the field. This interpretation helps to translate statistical findings into meaningful insights and practical implications.
- Professional Presentation: Ensure that all statistical findings are presented in a clear and professional manner. Use precise language and avoid jargon to make your results accessible to readers who may not have an extensive background in statistics. A well-presented results section not only conveys the outcomes of your analysis but also enhances the overall impact of your research.
By following these steps, you will create a well-organized and informative results section that clearly conveys the outcomes of your analysis. This approach provides valuable insights into your research question and ensures that your findings are communicated effectively, making your analysis both robust and comprehensible.
Conclusion
By systematically addressing each step—formulating hypotheses, evaluating assumptions, conducting your analysis, and interpreting results—you can tackle multiple regression and correlation assignments effectively. This structured approach ensures that you are not only adhering to best practices but also gaining a deep understanding of the statistical principles underlying your analysis.
Starting with clear and precise hypotheses establishes a solid foundation for your research, allowing you to focus on specific questions and predictions. Formulating well-defined null and alternative hypotheses directs your analysis and guides the interpretation of your results. Evaluating assumptions critically is crucial for ensuring that the statistical methods you apply are appropriate and that your results are valid. Checking for multicollinearity, examining residuals, and identifying outliers helps in confirming the robustness of your model and mitigating potential issues that could skew your findings.
Conducting your analysis with meticulous attention to detail helps in uncovering meaningful patterns and relationships within your data. Employing the correct statistical techniques and interpreting the results accurately is essential for drawing reliable conclusions. By examining standardized coefficients, significance levels, and overall model fit, you gain insights into which predictors are most influential and how well your model explains the outcome variable.
Finally, writing a clear and professional results section enables you to communicate your findings effectively. Presenting your results in an APA-style table and discussing their implications in a straightforward manner ensures that your conclusions are both accessible and impactful.
This comprehensive and methodical approach equips you to handle similar assignments with confidence and skill, whether you are working with complex datasets or exploring intricate relationships between variables. By adhering to these steps, you not only enhance the quality of your analysis but also build essential skills for tackling diverse statistical challenges. This structured methodology fosters a deeper understanding of data analysis, ultimately leading to more accurate, insightful, and actionable conclusions. It also prepares you for future assignments and professional work, where rigorous and thoughtful analysis is key to achieving meaningful results.