Avail Your Offer
Unlock success this fall with our exclusive offer! Get 20% off on all statistics assignments for the fall semester at www.statisticsassignmenthelp.com. Don't miss out on expert guidance at a discounted rate. Enhance your grades and confidence. Hurry, this limited-time offer won't last long!
We Accept
In the realm of health sciences, the pursuit of knowledge often hinges on the careful analysis of data. From identifying trends in disease prevalence to completing your health statistics assignment and evaluating the effectiveness of medical interventions, statistical analysis serves as the backbone of evidence-based decision-making. This blog delves into the crucial role statistical analysis plays in health sciences research, shedding light on its methodologies, challenges, and transformative potential.
Understanding Statistical Analysis
Statistical analysis is the process of collecting, organizing, interpreting, and drawing meaningful inferences from data. In health sciences research, this practice is essential for making informed decisions that impact patient care, public health policies, and medical advancements. Statistical techniques enable researchers to uncover patterns, relationships, and trends within datasets, providing a deeper understanding of the phenomena under investigation.
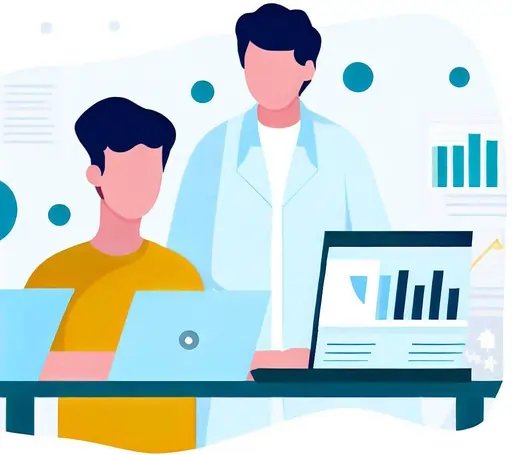
Key Methodologies in Statistical Analysis
- Descriptive Statistics: This initial step involves summarizing and describing the basic features of a dataset. Measures like mean, median, mode, and standard deviation help to provide a snapshot of the data's central tendency and variability.
- Inferential Statistics: This branch of statistical analysis involves drawing conclusions about a population based on a sample. Techniques such as hypothesis testing and confidence intervals aid researchers in making predictions and generalizations from limited data.
- Regression Analysis: Often used to explore relationships between variables, regression analysis helps researchers understand how one or more independent variables affect a dependent variable. In health sciences, this could mean examining how factors like age, diet, or genetics influence health outcomes.
- Survival Analysis: This methodology deals with time-to-event data, frequently applied in studies related to disease progression or patient survival rates. It accounts for the varying lengths of time that individuals are observed in a study.
- Meta-Analysis: Assembling and analyzing data from multiple studies, meta-analysis provides a comprehensive view of a particular research question. It helps identify consistent trends or discrepancies across various studies, enhancing the overall understanding of a topic.
Challenges in Health Sciences Statistical Analysis
Statistical analysis in health sciences research is a multifaceted endeavor that involves navigating a range of challenges to ensure the accuracy, reliability, and validity of the findings. Let's delve into four key challenges that researchers face when conducting statistical analysis in the field of health sciences:
- Biased Data:
- Selection Bias:If participants are not selected randomly, the sample might not be representative of the broader population. For instance, excluding certain demographics could lead to underrepresentation or overrepresentation, affecting the generalizability of results.
- Measurement Bias:Errors or inconsistencies in measurement methods can introduce bias. Researchers must ensure that measurements are accurate, reliable, and consistent across participants to avoid misleading conclusions.
- Data Collection Bias:Data collected in a non-standardized or inconsistent manner can introduce bias. Researchers should establish clear protocols for data collection and ensure that data are collected uniformly to mitigate this challenge.
Addressing bias often involves employing techniques such as random sampling, stratified sampling, and controlling for confounding variables. Transparent reporting of the methods used to mitigate bias enhances the credibility of research findings.
- Underpowered Studies: Studies with small sample sizes may lack the statistical power to detect true effects or relationships. As a result, significant findings might not emerge, leading to erroneous conclusions of no effect.
- Sample Size Considerations: Researchers must carefully determine the appropriate sample size based on factors such as the desired level of confidence, effect size, variability, and statistical tests to be employed. Power analysis aids in estimating the minimum sample size required for meaningful results.
- Resource Constraints: While larger samples enhance statistical power, managing and analyzing large datasets can be resource-intensive in terms of time, manpower, and computational resources.
Balancing sample size considerations involves trade-offs between statistical power and practical constraints. Researchers should strive to select a sample size that allows them to detect meaningful effects while considering available resources.
- Confounding Effects: When a confounding variable is not controlled for, it can lead to spurious relationships or associations. For example, if a study finds a correlation between a specific medication and improved health outcomes, but fails to account for factors like lifestyle or socioeconomic status, the observed relationship might be misleading.
- Controlled Studies: Researchers often use controlled studies or statistical techniques such as regression analysis to account for confounding variables. Matching, stratification, and randomization are strategies employed to minimize the impact of confounding.
- Causal Inference: Properly addressing confounding variables is crucial for making accurate causal inferences. Without proper control, researchers cannot confidently attribute observed effects to the manipulated variable.
Accounting for confounding variables requires meticulous planning, careful study design, and the use of appropriate statistical techniques to isolate the true relationship between variables of interest.
- Multivariate Analysis: In situations where multiple variables interact, multivariate analysis techniques such as multivariate regression, factor analysis, and principal component analysis are employed to disentangle complex relationships.
- Machine Learning: Advanced machine learning algorithms, such as neural networks and decision trees, are capable of identifying intricate patterns in large and complex datasets that traditional methods might miss.
- Nonlinear Relationships:Many health-related phenomena exhibit nonlinear relationships, which require specialized statistical approaches like nonlinear regression or spline regression to accurately model and analyze the data.
Employing advanced statistical methods to address complex relationships can unlock deeper insights, allowing researchers to better understand intricate interactions and dependencies within health data.
The Transformative Potential of Statistical Analysis
- Precision Medicine:
- Genomic Medicine: By analyzing an individual's genetic data, statistical techniques can identify genetic markers associated with diseases, drug responses, and susceptibility to certain conditions. This information enables clinicians to make more informed decisions about treatment options, drug dosages, and potential adverse effects, minimizing trial and error in medication choices.
- Environmental and Lifestyle Factors: Statistical analysis integrates genetic data with information about a person's environment and lifestyle. This holistic approach helps identify environmental factors that may interact with genetic predispositions, informing interventions such as dietary recommendations, exercise plans, and preventive measures tailored to an individual's specific needs.
- Predictive Analytics: Machine learning algorithms, a subset of statistical analysis, can predict disease risks based on multiple variables, including genetics, environment, and behavior. These predictions allow healthcare providers to proactively manage health conditions and promote wellness through personalized interventions.
- Disease Surveillance:Statistical analysis facilitates the monitoring and early detection of disease outbreaks. By analyzing trends in healthcare data, epidemiologists can identify unusual patterns, track the spread of infectious diseases, and implement timely public health interventions.
- Risk Factor Identification:Through case-control studies and cohort studies, statistical analysis helps identify risk factors associated with diseases. For example, it can reveal the link between smoking and lung cancer or between high-sugar diets and diabetes.
- Intervention Assessment: Epidemiologists use statistical techniques to evaluate the effectiveness of public health interventions, such as vaccination campaigns or smoking cessation programs. This information informs policymakers and guides the allocation of resources for disease prevention and control.
- Treatment Efficacy and Safety: Statistical methods are employed to analyze clinical trial data, comparing outcomes between treatment and control groups. This analysis helps determine whether a new drug or therapy is safe and effective, guiding regulatory decisions and medical practice.
- Dose Optimization: Statistical modeling assists in finding the optimal dosage for medications by analyzing the dose-response relationship. This ensures that patients receive the most effective treatment while minimizing adverse effects.
- Patient Stratification: Statistical analysis identifies subgroups of patients who are more likely to respond positively to a treatment. This leads to the development of targeted therapies that maximize benefits while reducing side effects for specific patient populations.
- Health Disparities: Statistical analysis reveals disparities in health outcomes among different populations, such as racial or socioeconomic disparities.
Ethical Considerations
Sound statistical analysis requires ethical considerations to ensure the validity and credibility of research findings:
- Data Privacy:
- Transparency:
- Informed Consent:
Data protection involves not only safeguarding data against unauthorized access but also ensuring that data are securely stored, transmitted, and processed. This requires implementing encryption, access controls, and secure storage practices. Additionally, data anonymization techniques, such as removing personally identifiable information, help reduce the risk of re-identification.
Breaches of data privacy not only violate the trust of participants but can also have legal and reputational consequences for researchers and institutions. Adhering to ethical guidelines and regulatory frameworks, such as the Health Insurance Portability and Accountability Act (HIPAA) in the United States, demonstrates a commitment to data privacy and builds confidence in research integrity.
When reporting statistical methods, researchers should detail the procedures used for data collection, preprocessing, and analysis. This includes specifying the statistical tests employed, the software or tools used, and any modifications made to the original data. Assumptions and limitations must be openly acknowledged, as they provide context for the interpretation of results.
Transparent reporting extends to publishing research findings in reputable journals or platforms. Peer review processes help ensure the accuracy and validity of the statistical analysis. By adhering to transparent practices, researchers contribute to the robustness of the scientific literature and foster trust within the scientific community and among the general public.
Obtaining informed consent involves clear communication. Researchers should present information in a language and format that participants can understand, avoiding jargon or technical terms that might confuse them. Participants should have the opportunity to ask questions and seek clarification before providing their consent.
In cases where obtaining informed consent is challenging due to factors like cognitive impairment or limited capacity, researchers must involve legally authorized representatives while still respecting the rights and best interests of the participant.
Failure to obtain informed consent can lead to ethical violations and undermine the validity of research findings. Ethical review boards and institutional review boards (IRBs) play a crucial role in ensuring that research protocols adhere to ethical standards and safeguard the rights and well-being of participants.
Conclusion
In the dynamic landscape of health sciences research, statistical analysis is the guiding light that transforms raw data into actionable insights. From unraveling the intricacies of disease progression to informing critical medical decisions, statistical methodologies empower researchers and healthcare practitioners alike. By navigating challenges and upholding ethical principles, statistical analysis paves the way for groundbreaking discoveries that shape the future of healthcare.