Claim Your Offer
Unlock an exclusive deal at www.statisticsassignmenthelp.com with our Spring Semester Offer! Get 10% off on all statistics assignments and enjoy expert assistance at an affordable price. Our skilled team is here to provide top-quality solutions, ensuring you excel in your statistics assignments without breaking the bank. Use Offer Code: SPRINGSAH10 at checkout and grab this limited-time discount. Don’t miss the chance to save while securing the best help for your statistics assignments. Order now and make this semester a success!
We Accept
- Understanding the Assignment
- Data Preparation and Exploration
- Checking for Missing Data
- Descriptive Statistics
- Conducting Factor Analysis
- Principle Components Analysis (PCA)
- Determining the Number of Factors
- Interpreting Factor Loadings
- Rotated Component Matrix
- Characterizing Factors
- Assessing Reliability with Cronbach’s Alpha
- Calculating Cronbach’s Alpha
- Creating Composite Scores
- Transform and Compute Variables
- Expected vs. Actual Scores
- Dimension Assessment
- Overall Green-ness Score
- Calculation
- Descriptive Statistics
- Interpretation
- Practical Application for Client Insights
- Segmentation
- Campaign Strategy
- Conclusion
Psychographic surveys are crucial for understanding consumer attitudes, values, and behaviors. These surveys assess psychological attributes, offering profound insights into consumer preferences. One vital application is gauging consumer environmentalism. This blog will guide you through creating composite scores from psychographic survey data to help solve your statistics assignment and derive meaningful insights.
Understanding the Assignment
Psychographic survey assignments typically involve a set of items that measure different aspects of consumer attitudes or behaviors. In the case of environmentalism, the survey might include items that assess preferences for organic food, recycling habits, and overall environmental consciousness. These items are usually rated on a scale (e.g., 1-7), where respondents indicate their level of agreement with each statement.
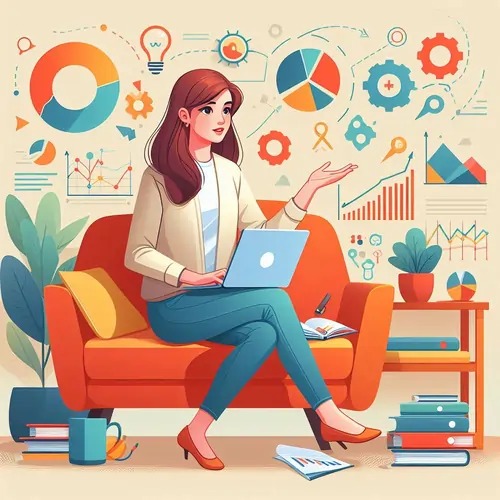
Your task in such assignments is to analyze the survey data to identify underlying dimensions of environmental attitudes and create composite scores that represent these dimensions. These composite scores can then be used to provide actionable insights for your client.
Data Preparation and Exploration
Before diving into complex analyses, it’s crucial to familiarize yourself with the data and ensure it’s ready for analysis.
Checking for Missing Data
The first step is to check for any missing data in your dataset. Missing data can skew your results and lead to inaccurate conclusions. In most statistical software, you can easily identify missing values and decide on an appropriate method to handle them. Common methods include imputation (filling in missing values with the mean or median) or listwise deletion (removing cases with missing values).
Descriptive Statistics
Next, calculate basic descriptive statistics for each survey item. This includes measures like the mean, median, standard deviation, and range. Descriptive statistics provide a snapshot of your data, helping you understand the distribution and central tendencies of each item.
For example, if you’re analyzing a survey item like “I prefer to eat organic when possible,” you might find that the mean score is 5.2 on a 1-7 scale, indicating a general preference for organic food among respondents.
Conducting Factor Analysis
Factor analysis is a statistical technique used to identify underlying relationships between variables. It helps you reduce a large number of items into a smaller set of factors, making it easier to interpret the data.
Principle Components Analysis (PCA)
Principle Components Analysis (PCA) is a common method for conducting factor analysis. PCA reduces the dimensionality of your data by transforming the original variables into a set of uncorrelated components.
- Varimax Rotation: Varimax rotation is used to make the output more interpretable. It maximizes the variance of squared loadings of a factor, making it easier to identify which variables are associated with each factor.
- Kaiser Normalization: This ensures that the factors are orthogonal, meaning they are uncorrelated with each other.
Determining the Number of Factors
To determine the number of factors, look at the eigenvalues and the scree plot. Eigenvalues indicate the amount of variance explained by each factor. Typically, factors with eigenvalues greater than 1 are considered significant.
The scree plot is a graphical representation of the eigenvalues. Look for an “elbow” point where the plot levels off, indicating the optimal number of factors.
Interpreting Factor Loadings
Once you have determined the number of factors, the next step is to interpret the factor loadings. The factor loadings indicate the strength and direction of the relationship between each item and the underlying factors.
Rotated Component Matrix
The rotated component matrix shows the factor loadings after rotation. Highlight the variables with high loadings (usually above 0.4) on each factor. These are the items that strongly correlate with the underlying factor.
For example, in a survey assessing environmentalism, you might find that items like “I recycle” and “I prefer to eat organic” load highly on the same factor. This factor could represent a dimension of “environmentally friendly behaviors.”
Characterizing Factors
Describe each factor based on the items that load onto it. For instance, a factor characterized by high loadings on items related to recycling and organic food preferences might be labeled as “Recycling and Organic Preferences.”
Characterizing the factors helps in understanding the dimensions of environmental attitudes represented in the survey. It also provides a basis for creating composite scores that summarize these dimensions.
Assessing Reliability with Cronbach’s Alpha
Cronbach’s alpha is a measure of internal consistency, indicating how well the items within each factor measure the same underlying construct. Higher alpha values suggest greater reliability.
Calculating Cronbach’s Alpha
Run a reliability analysis for each set of items associated with a factor. In most statistical software, this involves selecting the items and calculating the alpha coefficient.
- Acceptable Range: Cronbach’s alpha values above 0.7 are generally considered acceptable. This indicates that the items within each factor are reliably measuring the same underlying dimension.
For example, if the items related to “Recycling and Organic Preferences” have a Cronbach’s alpha of 0.85, it suggests high internal consistency and reliability.
Creating Composite Scores
Composite scores are average scores for items loading on each factor. These scores simplify data interpretation and provide a single value representing each dimension.
Transform and Compute Variables
In statistical software like SPSS, use the transform function to create new variables representing each factor. This involves computing the average score for the items loading on each factor.
- Descriptive Statistics for New Variables: Calculate the mean, standard deviation, minimum, and maximum for each composite score. This provides a summary of the new variables and helps in understanding their distribution.
For example, if the composite score for “Recycling and Organic Preferences” has a mean of 5.4, it indicates a high level of environmental friendliness among respondents.
Expected vs. Actual Scores
Compare the expected and actual scores for each composite score. The expected scores are based on the range of the original items, while the actual scores are calculated from the data.
- Discrepancies: Discuss any discrepancies between expected and actual scores and possible reasons. For example, if the actual scores are lower than expected, it could indicate a more moderate level of agreement with environmental statements.
Dimension Assessment
Explain what each composite score assesses. For instance, a composite score for “Recycling and Organic Preferences” indicates respondents’ overall inclination towards recycling and preferring organic products.
Overall Green-ness Score
The overall green-ness score is an average of the original items, providing a single measure of environmental orientation.
Calculation
Use the transform function to compute this variable by averaging the original items.
Descriptive Statistics
Provide the minimum, maximum, mean, and standard deviation for the overall green-ness score. This summary helps in understanding the general level of environmentalism among respondents.
Interpretation
Interpret the overall green-ness score to provide insights. A higher score indicates a stronger environmental orientation, while a lower score suggests a more moderate stance.
Practical Application for Client Insights
Once you have your composite scores, consider how these insights can help your client.
Segmentation
Identify different consumer segments based on their green-ness scores. Tailor marketing messages to each segment’s environmental attitudes.
For example, consumers with high scores on “Recycling and Organic Preferences” can be targeted with campaigns emphasizing eco-friendly packaging and organic products.
Campaign Strategy
Use the composite scores to highlight key environmental issues in your client’s campaign. This ensures the messaging resonates with the target audience’s values and behaviors.
Conclusion
Psychographic survey assignments require a systematic approach to uncover meaningful insights. By following these steps, you can confidently analyze similar assignments, providing valuable data-driven recommendations. This process ensures you understand the data from preparation to practical application, empowering you to excel in psychographic analysis assignments.
By mastering the techniques outlined in this blog, you can handle any psychographic survey assignment with confidence. The ability to analyze and interpret survey data is crucial for providing actionable insights and supporting your clients in making informed decisions. Whether you are a student or a professional, these skills are invaluable in the field of market research and consumer analysis.
Remember, the key to success lies in thorough data preparation, careful factor analysis, reliable composite score creation, and insightful interpretation. With these tools at your disposal, you can tackle any psychographic survey assignment and deliver meaningful results.