Avail Your Offer
Unlock success this fall with our exclusive offer! Get 20% off on all statistics assignments for the fall semester at www.statisticsassignmenthelp.com. Don't miss out on expert guidance at a discounted rate. Enhance your grades and confidence. Hurry, this limited-time offer won't last long!
We Accept
- Understanding Two-Factor ANOVA
- The Basics of Two-Factor ANOVA
- Pearson Correlation Analysis
- Key Points of Pearson Correlation
- Advantages and Disadvantages
- Spearman Correlation
- Approach to Spearman Correlation
- When to Use Spearman Over Pearson
- Linear Regression
- Approach to Linear Regression
- Understanding Regression Outputs
- General Tips for Solving Statistics Assignments
- Conclusion
Statistics assignments frequently require tackling intricate analyses and interpretations, encompassing various techniques such as Two-Factor ANOVA, Pearson Correlation, Spearman Correlation, and Linear Regression. Mastering these methods is crucial for effectively addressing and solving complex statistical problems. For instance, if you need to solve your ANOVA assignment, it is vital to grasp the underlying principles and methodologies that govern these analyses. Understanding how to approach and break down these problems can significantly enhance your ability to perform rigorous statistical evaluations and interpret results accurately. This blog provides a general framework designed to guide you through these statistical tasks, emphasizing essential steps and strategies to help you succeed in your assignments. By familiarizing yourself with these techniques, you'll be better equipped to handle diverse statistical problems and achieve reliable, insightful outcomes.
Understanding Two-Factor ANOVA
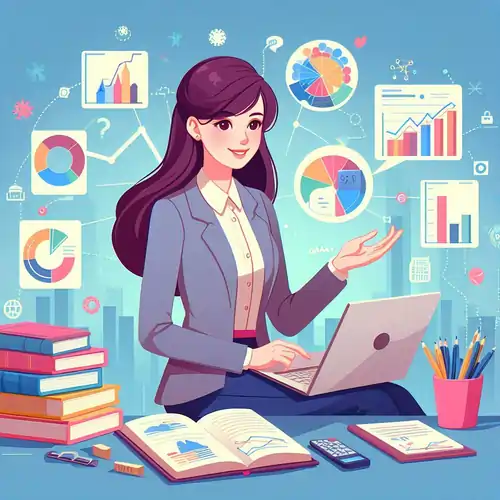
Two-Factor ANOVA is a powerful statistical technique that allows researchers to evaluate the influence of two independent variables on a dependent variable simultaneously. Unlike one-way ANOVA, which examines the impact of a single factor, Two-Factor ANOVA considers the combined effects of two different factors and their interaction. This method is particularly useful when you need to understand how different factors work together to affect an outcome.
In practical terms, Two-Factor ANOVA helps identify main effects (the individual impact of each factor) and interaction effects (how the factors influence each other). For instance, in a study examining how different teaching methods and study times affect student performance, Two-Factor ANOVA would reveal not only the impact of each teaching method and study time individually but also how these factors interact to influence the results. This comprehensive analysis is crucial for drawing nuanced conclusions in experimental research.
The Basics of Two-Factor ANOVA
Two-Factor ANOVA is a statistical method used to assess the effect of two independent variables on a dependent variable. It is particularly useful when you want to understand how different factors interact and influence the outcome. Here’s a general approach to tackle a Two-Factor ANOVA problem:
- Define the Research Question: Start by formulating a clear research question that involves two factors. For example, you might investigate how teaching methods and study time affect student performance.
- Identify Variables:
- Independent Variables:These are the factors you manipulate. For instance, teaching methods and study time.
- Dependent Variable:The outcome you measure, such as student performance.
- Understand Main Effects and Interaction:
- Main Effects: The individual impact of each factor on the dependent variable.
- Interaction Effect:Occurs when the effect of one factor depends on the level of the other factor.
- Perform the Analysis:
- Calculate sums of squares for each factor and their interaction.
- Compute mean squares by dividing sums of squares by degrees of freedom.
- Determine F-values and compare them to critical values to decide on significance.
- Compute effect sizes to understand the strength of the effects.
- Interpret Results: Summarize whether each factor has a significant effect and whether there is an interaction between factors.
Pearson Correlation Analysis
Pearson correlation analysis is a fundamental statistical technique used to assess the strength and direction of the linear relationship between two continuous variables. This method provides a correlation coefficient, rrr, which ranges from -1 to 1. A value of 1 indicates a perfect positive linear relationship, -1 signifies a perfect negative linear relationship, and 0 means no linear relationship. This analysis is useful for understanding how changes in one variable are associated with changes in another.
When conducting Pearson correlation, it's essential to check if your data meet the assumptions: normality, linearity, and homoscedasticity (constant variance of errors). Calculating the correlation coefficient involves finding the covariance of the variables divided by the product of their standard deviations. Interpreting the results requires comparing the coefficient against critical values to determine statistical significance, helping to ascertain whether observed relationships are likely due to chance or reflect real patterns.
Key Points of Pearson Correlation
Pearson correlation measures the strength and direction of the linear relationship between two continuous variables. Here’s how to approach a Pearson correlation problem:
- State the Research Question: Formulate a question that examines the relationship between two variables. For instance, you might explore how hours of study correlate with exam scores.
- Calculate the Correlation Coefficient:
- Use the Pearson correlation formula to find the coefficient rrr, which indicates the strength and direction of the relationship.
- Assess Significance:
- Compare the calculated rrr with critical values from the correlation table to determine if the relationship is statistically significant.
- Interpret Results: Discuss the strength, direction, and significance of the relationship between the variables.
Advantages and Disadvantages
Advantages:
- Provides a clear measure of the linear relationship.
- Easy to calculate and interpret.
Disadvantages:
- Only measures linear relationships.
- Sensitive to outliers.
Spearman Correlation
Spearman correlation is a powerful tool for evaluating the relationship between two variables, especially when dealing with ordinal data or non-normally distributed continuous data. Unlike Pearson correlation, which measures linear relationships between continuous variables, Spearman's method assesses how well the relationship between two variables can be described by a monotonic function. This means it is particularly useful when the data does not meet the assumptions required for Pearson's correlation.
To use Spearman correlation effectively, you first convert your data into ranks. This process involves ordering the data and assigning ranks to each value, which helps in dealing with non-linear relationships and ordinal data where the exact distances between values are not meaningful. Spearman’s rs coefficient ranges from -1 to 1, indicating the strength and direction of the monotonic relationship between the variables. This makes it a versatile tool for various types of data analysis.
Approach to Spearman Correlation
- Rank the Data: Assign ranks to your data points. If there are tied ranks, adjust accordingly.
- Calculate Spearman's rs:
- Use the formula for Spearman's rank correlation to compute the coefficient rsr_srs.
- Determine Significance:
- Compare the computed rsr_srs with critical values to assess significance.
- Interpret Results: Discuss the direction and significance of the relationship, noting that Spearman correlation is used for ordinal data or non-linear relationships.
When to Use Spearman Over Pearson
- Spearman is preferred when data are not normally distributed or are ordinal.
- Pearson is used for continuous, normally distributed data with a linear relationship.
Linear Regression
Linear regression is a statistical technique that explores the relationship between two continuous variables. The primary goal is to model the dependent variable as a function of the independent variable, providing insights into how changes in the independent variable affect the dependent variable.
In practice, linear regression helps in predicting outcomes based on known data. For instance, if you have data on hours studied and corresponding exam scores, linear regression can help predict scores based on the number of hours studied. This technique involves fitting a straight line through the data points to minimize the differences between the observed values and the values predicted by the model.
For those seeking assistance with linear regression assignments, understanding the process involves interpreting coefficients to determine the nature of the relationship between variables. Analyzing the fit of the model helps assess how well it explains the variability in the dependent variable, guiding decisions and predictions based on the model’s results.
Approach to Linear Regression
- Formulate the Research Question: Define a question where you predict one variable based on another. For example, predicting exam scores from hours of study.
- Calculate the Regression Coefficients:
- Determine the slope (bbb) and intercept (aaa) of the regression line using statistical formulas.
- Assess the Model’s Fit:
- Perform an ANOVA test on the regression model to check if the model significantly predicts the dependent variable.
- Compute Standard Error: This measures the accuracy of predictions made by the regression model.
- Interpret Results: Discuss the regression equation, its significance, and how well it predicts the dependent variable.
Understanding Regression Outputs
- Slope (b): Indicates the change in the dependent variable for each unit change in the independent variable.
- Intercept (a): Represents the value of the dependent variable when the independent variable is zero.
General Tips for Solving Statistics Assignments
- Understand the Requirements: Carefully read the assignment to ensure you understand what is being asked.
- Organize Your Data: Structure your data and calculations clearly to avoid confusion and errors.
- Show All Work: Document each step of your calculations to ensure transparency and facilitate easier grading.
- Use Statistical Software: Tools like SPSS, R, or Excel can simplify complex calculations and analyses.
- Review Your Results: Double-check your calculations and interpretations to ensure accuracy.
- Seek Feedback: If possible, discuss your approach with peers or instructors to validate your methodology.
Conclusion
By applying these general strategies, you can effectively handle a wide range of statistics assignments. Whether you're working on ANOVA, correlation, or regression, understanding the core principles and following a structured approach will help you tackle these tasks with confidence. Remember, the key to success in statistics is a solid grasp of the concepts, attention to detail, and practice. With these strategies, you'll be well-equipped to complete your statistics assignment efficiently and accurately.