Claim Your Offer
Unlock an exclusive deal at www.statisticsassignmenthelp.com with our Spring Semester Offer! Get 10% off on all statistics assignments and enjoy expert assistance at an affordable price. Our skilled team is here to provide top-quality solutions, ensuring you excel in your statistics assignments without breaking the bank. Use Offer Code: SPRINGSAH10 at checkout and grab this limited-time discount. Don’t miss the chance to save while securing the best help for your statistics assignments. Order now and make this semester a success!
We Accept
- Understanding the Basics
- Key Multivariate Techniques
- Multiple Regression Analysis
- ANOVA and MANOVA
- Factor Analysis
- Cluster Analysis
- Practical Tips for Effective Assignment Completion
- Conclusion
Multivariate statistics can seem daunting at first, but with the right strategies and a solid understanding of the key concepts, you can solve your statistics assignment with confidence. This guide aims to provide you with the essential tools and techniques needed to successfully navigate through multivariate statistics assignments. Whether you are dealing with complex datasets, conducting multiple regression analyses, performing factor analysis, or interpreting the results of various multivariate tests, these tips will help you achieve better outcomes in your assignments. By breaking down complex concepts into manageable steps and offering practical advice, this guide ensures that you can approach your assignments methodically and efficiently. With a focus on understanding the underlying principles and applying the correct statistical methods, you will be well-equipped to handle any challenges that come your way. For those seeking help with multivariate statistics assignments, whether you are a beginner or looking to refine your skills, these strategies will help you excel.
Understanding the Basics
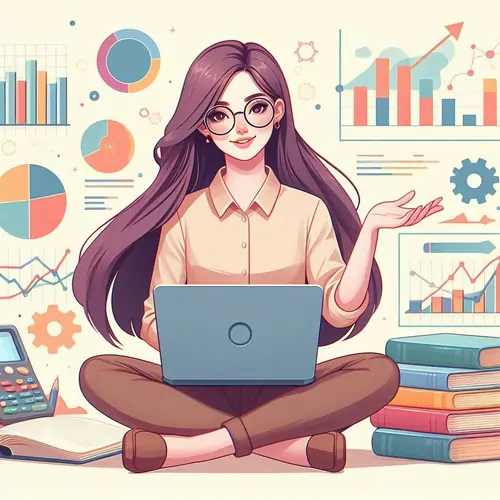
Before diving into the complexities of multivariate statistics, it's crucial to have a firm grasp of the basics:
- Variables and Data Types: Understand the types of variables you are dealing with—dependent variables (DVs) and independent variables (IVs). DVs are the outcomes you're trying to predict or explain, while IVs are the predictors or factors influencing the DVs. Also, be familiar with the data types (nominal, ordinal, interval, and ratio), as each type has specific characteristics and determines the kind of analysis you can perform.
- Data Cleaning and Preparation: Clean your data to remove any inconsistencies or outliers. This involves checking for missing values, identifying and handling duplicates, ensuring data integrity, and possibly transforming variables to suit your analysis. Proper data preparation is crucial as it enhances the accuracy and reliability of your statistical results.
- Descriptive Statistics: Start with basic descriptive statistics to summarize your data. This includes measures of central tendency (mean, median, mode) which provide insights into the average values, and measures of dispersion (range, variance, standard deviation) that reveal the spread or variability within your data. Descriptive statistics help in understanding the general characteristics of your dataset.
Key Multivariate Techniques
Here are some essential multivariate techniques you should be familiar with:
Multiple Regression Analysis
Multiple regression is used to predict the value of a dependent variable based on the values of two or more independent variables. Here’s a step-by-step approach:
- Model Specification: Define your dependent variable (DV) and independent variables (IVs). For example, if you want to predict group membership (DV) based on SAT scores and high school GPA (IVs), specify these variables in your model.
- Assumptions Check: Ensure that your data meets the assumptions of multiple regression, including linearity, independence, homoscedasticity, and normality of residuals.
- Fit the Model: Use statistical software (e.g., SPSS, R, Python) to fit the regression model. Interpret the coefficients to understand the relationship between your IVs and DV.
- Model Evaluation: Evaluate the model’s performance using R-squared, adjusted R-squared, and other goodness-of-fit measures. Check for multicollinearity using Variance Inflation Factor (VIF).
ANOVA and MANOVA
ANOVA(Analysis of Variance) and MANOVA (Multivariate Analysis of Variance) are used to compare means across different groups.
- ANOVA: Use ANOVA when you have one dependent variable and want to compare means across multiple groups. For example, compare the effectiveness of different teaching methods on student performance.
- MANOVA: Use MANOVA when you have multiple dependent variables. This technique helps in understanding the combined effect of IVs on several DVs simultaneously.
- Assumptions Check: Ensure that your data meets the assumptions of ANOVA/MANOVA, including independence of observations, normality, and homogeneity of variances.
- Interpret Results: Look at the F-statistic and p-value to determine if there are significant differences between groups. For MANOVA, check the multivariate test statistics like Wilks' Lambda.
Factor Analysis
Factor analysis is used to identify underlying relationships between variables. It helps in data reduction by grouping related variables into factors.
- Identify Factors: Determine the number of factors to extract using techniques like Kaiser’s criterion (eigenvalue > 1) and scree plot analysis.
- Rotation: Use rotation methods (e.g., Varimax) to make the factors more interpretable.
- Interpret Factors: Label the factors based on the variables that load highly on them. This helps in understanding the underlying dimensions of your data.
Cluster Analysis
Cluster analysis groups similar observations into clusters. It’s useful in market segmentation, pattern recognition, and data exploration.
- Choose Clustering Method: Select a clustering method (e.g., K-means, hierarchical clustering) based on your data and research objectives. For instance, K-means is popular for its simplicity and efficiency.
- Determine Number of Clusters: Use techniques like the Elbow method or silhouette analysis to decide the optimal number of clusters. These methods help in finding the best number of clusters that balance within-cluster similarity and between-cluster dissimilarity.
- Interpret Clusters: Analyze the characteristics of each cluster to draw meaningful insights. For example, in market segmentation, clusters might represent different customer segments with distinct purchasing behaviors.
Practical Tips for Effective Assignment Completion
Here are some practical tips to help you effectively complete your multivariate statistics assignments:
- Understand the Problem Statement: Carefully read the assignment instructions and understand the research question. Clearly identify the DVs and IVs to determine the appropriate statistical techniques.
- Choose the Right Technique: Select the appropriate statistical technique based on your research question and data characteristics. Using the wrong technique can lead to incorrect conclusions.
- Use Statistical Software: Leverage statistical software like SPSS, R, or Python to perform your analyses. These tools offer a wide range of functions and are essential for handling complex data efficiently. Familiarize yourself with the software's features to streamline your analysis process.
- Interpret Results Carefully: Focus on interpreting the results accurately. Beyond p-values, consider effect sizes, confidence intervals, and practical significance to understand the real-world implications of your findings.
- Visualize Your Data: Use charts and graphs to visualize your data and results. Effective visualization can reveal patterns and trends that are not immediately apparent from raw data, helping you communicate your findings more clearly.
Conclusion
Multivariate statistics can indeed present significant challenges, but with the right strategies and tools at your disposal, you can confidently navigate and master any assignment. The journey starts with a solid understanding of the fundamental concepts and progresses through the careful selection of appropriate statistical techniques tailored to your specific research questions and data characteristics. Equally important is the meticulous interpretation of your results, ensuring that your conclusions are both accurate and meaningful. Embrace the complexities of multivariate analysis with patience and an open mind, as each step provides valuable insights into your data. Remember, proficiency in multivariate statistics doesn’t come overnight; it requires consistent practice, ongoing learning, and a willingness to adapt to new methods and technologies. By remaining dedicated and persistent, you’ll not only unlock the full potential of your data but also develop a deeper appreciation for the power and versatility of statistical analysis in addressing complex research problems. Happy analyzing!