Claim Your Offer
Unlock a fantastic deal at www.statisticsassignmenthelp.com with our latest offer. Get an incredible 10% off on all statistics assignment, ensuring quality help at a cheap price. Our expert team is ready to assist you, making your academic journey smoother and more affordable. Don't miss out on this opportunity to enhance your skills and save on your studies. Take advantage of our offer now and secure top-notch help for your statistics assignments.
We Accept
- Understanding Correlation and Causation
- Correlation vs. Causation
- Identifying Causation Fallacies
- Building a Strong Foundation: Basic Statistical Tests
- Practical Application: Hypothesis Testing
- Formulating Hypotheses
- Advanced Analysis: Multivariate Regression
- Introduction to Multivariate Regression
- Steps to Conduct Multivariate Regression
- Case Study: Job Satisfaction Analysis
- Step 1: Formulate Hypotheses
- Step 2: Prepare Data
- Step 3: Run the Regression
- Step 4: Interpret Results
- Exploring More Complex Scenarios
- Contingency Tables and Chi-Square Tests
- Example: Sex and Job Satisfaction
- Analyzing Variance with ANOVA
- Example: Job Satisfaction Across Age Groups
- Moving Towards Multivariate Analysis
- The Power of Multiple Regression
- Steps to Conduct Multiple Regression
- Example: Job Satisfaction Analysis
- Interpreting Regression Coefficients
- Example: Job Satisfaction Analysis
- Addressing Multicollinearity
- Detecting Multicollinearity
- Addressing Multicollinearity
- Practical Tips for Robust Statistical Analysis
- Conclusion
In the world of statistics, interpreting data correctly is paramount. One common pitfall that students and professionals alike encounter is mistaking correlation for causation. This blog will guide you through the journey from identifying misleading correlations to mastering robust multivariate regression analysis. By the end of this journey, you will be equipped with the tools and knowledge to solve your statistical Analysis assignments with confidence.
Understanding Correlation and Causation
Grasping the difference between correlation and causation is vital for accurate data analysis. While correlation shows relationships, causation indicates direct effects. Misinterpreting these can lead to flawed conclusions. Recognize the distinction to avoid analytical pitfalls and make informed, reliable decisions.
Correlation vs. Causation
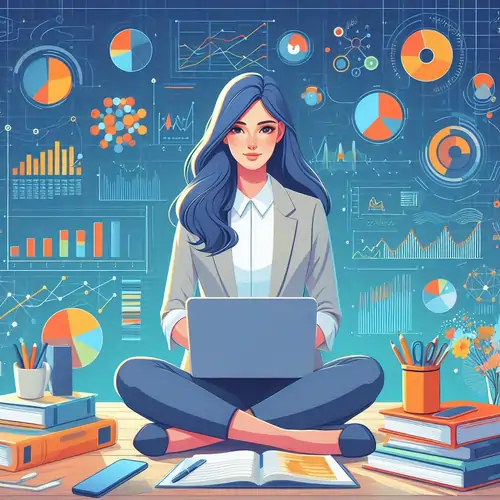
Correlation is a statistical measure that describes the extent to which two variables move in relation to each other. However, correlation does not imply causation. Just because two variables are correlated does not mean that one causes the other. For example, the correlation between ice cream sales and assaults may suggest a link, but introducing a third variable—such as weather—reveals that both are actually related to the time of year and not directly to each other.
In simpler terms, two things can move together (correlate) without one causing the other. This is why understanding the difference between correlation and causation is critical in statistical analysis. Misinterpreting these can lead to incorrect conclusions and flawed decision-making.
Identifying Causation Fallacies
- Spurious Correlation:This occurs when two variables appear to be related but are actually influenced by a third variable. In the ice cream and assault example, the weather is the lurking variable that affects both. Recognizing spurious correlations is crucial to avoid drawing incorrect conclusions from data.
- Tertium Quid Fallacy:This refers to the error of assuming a direct relationship between two variables without considering a third variable that may be influencing both. For example, if an increase in fitness and health is attributed solely to gym attendance without considering diet and lifestyle, it may lead to misleading conclusions.
- Large Sample Fallacy: Misinterpreting statistical significance as practical significance, especially in large datasets where even trivial correlations can appear significant. In large samples, almost any correlation can be statistically significant, but it doesn't mean the correlation is meaningful or practical.
Building a Strong Foundation: Basic Statistical Tests
Before diving into multivariate regression, it is essential to understand basic statistical tests and when to use them:
- Chi-Square Test:Used for testing relationships between categorical variables. For example, you might use a chi-square test to examine the relationship between gender (male/female) and voting preference (yes/no).
- T-test of Means:Compares the means of two groups to determine if they are significantly different. This test is useful when comparing the average scores of two different groups, such as the test scores of students from two different schools.
- ANOVA (Analysis of Variance): Compares means across three or more groups. This is particularly useful in experiments with multiple groups, such as comparing the effectiveness of three different teaching methods on student performance.
- Pearson's Correlation: Measures the linear relationship between two continuous variables. For example, Pearson's correlation can help determine the strength and direction of the relationship between hours of study and exam scores.
- Ordinary Least Squares (OLS) Regression:Models the relationship between a dependent variable and one or more independent variables. OLS regression is foundational in understanding how multiple factors can influence an outcome.
Practical Application: Hypothesis Testing
Hypothesis testing provides a structured approach to validating assumptions and drawing conclusions from data. By formulating clear hypotheses and selecting appropriate statistical tests, researchers can uncover meaningful insights and make informed decisions based on empirical evidence.
Formulating Hypotheses
A null hypothesis (H0) typically states that there is no effect or no difference, while an alternative hypothesis (HA) suggests the presence of an effect or difference. For example, in examining donations between Protestants and Catholics, the null hypothesis might state: "There is no difference between Protestants and Catholics in donations."
Hypothesis testing is a critical part of statistical analysis. It provides a structured approach to testing claims or assumptions about a population. Understanding how to formulate and test hypotheses is essential for conducting robust statistical analyses.
Advanced Analysis: Multivariate Regression
Introduction to Multivariate Regression
Multivariate regression allows you to analyze the relationship between one dependent variable and multiple independent variables. This approach helps in understanding the combined effect of several predictors on the outcome variable.
Multivariate regression is a powerful tool in statistical analysis. It helps in understanding the influence of multiple variables on a single outcome. This is particularly useful in fields like economics, social sciences, and health sciences, where multiple factors often influence outcomes.
Steps to Conduct Multivariate Regression
- Identify Variables:Choose your dependent variable (e.g., job satisfaction) and independent variables (e.g., age, sex, income). Identifying the correct variables is crucial as it lays the foundation for your analysis.
- Prepare Data: Clean your dataset by handling missing values and ensuring all variables are appropriately scaled. Data preparation involves several steps including data cleaning, transformation, and normalization.
- Run the Regression Model: Use statistical software (such as SPSS, R, or Python) to run the regression analysis. Running the regression involves using the right software and understanding the commands and functions.
- Interpret Results: Look at the coefficients, significance levels, and overall model fit (R-squared value) to interpret the results. Interpreting results correctly is crucial to draw meaningful conclusions from your analysis.
Case Study: Job Satisfaction Analysis
Let's walk through an example involving job satisfaction among college graduates:
Step 1: Formulate Hypotheses
- Null Hypothesis (H0):Growing older does not lead to increasing levels of job satisfaction.
- Alternative Hypothesis (HA):For college graduates, aging leads to a higher level of job satisfaction.
Formulating the right hypothesis is crucial as it directs the course of your analysis. In this case, we are interested in understanding the relationship between age and job satisfaction.
Step 2: Prepare Data
- Download the dataset and clean it by setting missing values appropriately.
- Create a composite index of job satisfaction if there are multiple related variables.
Data preparation is a critical step in any statistical analysis. It ensures that your data is ready for analysis and that your results will be accurate and reliable.
Step 3: Run the Regression
- Use age as the independent variable and job satisfaction index as the dependent variable.
- Control for other variables such as sex and income to see their effects on job satisfaction.
Running the regression involves using statistical software to analyze your data. In this case, we are using age as the independent variable and job satisfaction as the dependent variable.
Step 4: Interpret Results
- Check the coefficients for each independent variable to understand their impact.
- Look at the p-values to determine if the relationships are statistically significant.
- Assess the R-squared value to see how well your model explains the variability in job satisfaction.
Interpreting results is perhaps the most crucial step in the analysis. It involves understanding the coefficients, significance levels, and overall model fit.
Exploring More Complex Scenarios
Delve deeper into statistical analysis with contingency tables, ANOVA tests, and advanced regression techniques. These tools uncover intricate relationships and provide deeper insights into complex data, guiding comprehensive statistical exploration.
Contingency Tables and Chi-Square Tests
Contingency tables (or cross-tabulations) are a fundamental tool for analyzing the relationship between two categorical variables. They provide a visual representation of the frequencies of different combinations of variables.
For instance, if you want to explore the relationship between sex and job satisfaction, a contingency table can show you the frequency of males and females reporting different levels of job satisfaction.
The Chi-Square test is then used to determine whether there is a statistically significant association between the variables in the contingency table. This test compares the observed frequencies in the table to the frequencies we would expect if there were no association between the variables.
Example: Sex and Job Satisfaction
- Step 1:Create a contingency table of sex by job satisfaction.
- Step 2:Use the Chi-Square test to evaluate the association.
- Step 3:Interpret the Chi-Square value and p-value to determine if the association is significant.
Analyzing Variance with ANOVA
ANOVA (Analysis of Variance) is used to compare means across three or more groups. It helps determine if at least one of the group means is significantly different from the others.
For example, you might use ANOVA to compare job satisfaction across different age groups.
Example: Job Satisfaction Across Age Groups
- Step 1: Define your groups (e.g., age groups).
- Step 2:Conduct the ANOVA test to compare means across the groups.
- Step 3: Interpret the F-statistic and p-value to determine if there are significant differences.
ANOVA is particularly useful in experiments where you want to compare the effects of different treatments or conditions.
Moving Towards Multivariate Analysis
As you progress towards multivariate analysis, you'll gain deeper insights into how multiple factors influence outcomes. This advanced approach allows for a comprehensive understanding of complex relationships, equipping you to conduct more sophisticated statistical analyses with confidence.
The Power of Multiple Regression
Multiple regression extends simple linear regression by allowing you to include multiple predictors. This provides a more comprehensive understanding of the factors influencing the dependent variable.
Steps to Conduct Multiple Regression
- Select Variables:Choose your dependent and multiple independent variables.
- Check Assumptions:Ensure your data meets the assumptions of multiple regression (e.g., linearity, homoscedasticity, multicollinearity).
- Run the Model:Use statistical software to run the regression.
- Interpret Results: Analyze the coefficients, significance levels, and overall model fit.
Example: Job Satisfaction Analysis
Suppose we want to analyze how job satisfaction is influenced by age, sex, and income.
- Step 1:Identify the dependent variable (job satisfaction) and independent variables (age, sex, income).
- Step 2: Prepare the data by handling missing values and ensuring variables are appropriately scaled.
- Step 3: Run the multiple regression model using statistical software.
- Step 4: Interpret the coefficients, p-values, and R-squared value to understand the relationships.
Interpreting Regression Coefficients
Understanding regression coefficients is crucial for interpreting the results of a regression analysis. Each coefficient represents the change in the dependent variable for a one-unit change in the independent variable, holding all other variables constant.
- Positive Coefficient:Indicates a positive relationship between the independent and dependent variables.
- Negative Coefficient: Indicates a negative relationship between the independent and dependent variables.
- Significance Levels (p-values):Indicate whether the relationship between the variables is statistically significant.
Example: Job Satisfaction Analysis
- Age Coefficient: A positive coefficient suggests that as age increases, job satisfaction increases.
- Sex Coefficient: A negative coefficient suggests that one sex has lower job satisfaction compared to the other, controlling for other variables.
- Income Coefficient:A positive coefficient suggests that higher income is associated with higher job satisfaction.
Addressing Multicollinearity
Multicollinearity occurs when independent variables in a regression model are highly correlated with each other. This can inflate the variance of the coefficient estimates and make the model unstable.
Detecting Multicollinearity
- Variance Inflation Factor (VIF): Measures how much the variance of an estimated regression coefficient increases if your predictors are correlated. A VIF above 10 indicates high multicollinearity.
- Correlation Matrix:Displays the pairwise correlations between independent variables. High correlations (e.g., above 0.8) indicate potential multicollinearity.
Addressing Multicollinearity
- Remove Highly Correlated Predictors: If two variables are highly correlated, consider removing one from the model.
- Principal Component Analysis (PCA):Reduces the dimensionality of the data by transforming the correlated variables into a smaller number of uncorrelated variables.
Practical Tips for Robust Statistical Analysis
- Data Quality: Ensure your data is accurate, complete, and relevant. Data quality is the foundation of reliable analysis.
- Appropriate Tests:Choose the right statistical tests based on your research questions and data type.
- Assumption Checks:Verify that your data meets the assumptions of the statistical tests you are using.
- Clear Interpretation:Focus on interpreting your results in the context of your research questions. Avoid over-interpreting non-significant results.
- Report Findings Transparently: Clearly report your findings, including limitations and potential biases.
Conclusion
Understanding the difference between correlation and causation is crucial for accurate data interpretation. By mastering basic statistical tests and progressing to multivariate regression, you can solve complex statistics assignments with ease. Remember, the key to robust analysis lies in careful data preparation, appropriate selection of tests, and thorough interpretation of results.
By embracing these statistical techniques and tools, you can enhance your analytical skills and make informed decisions based on data. This journey from misleading correlations to robust multivariate regression is not just about learning statistical methods; it's about developing a critical mindset that questions assumptions, seeks evidence, and draws valid conclusions. Happy analyzing!