Avail Your Offer
Unlock success this fall with our exclusive offer! Get 20% off on all statistics assignments for the fall semester at www.statisticsassignmenthelp.com. Don't miss out on expert guidance at a discounted rate. Enhance your grades and confidence. Hurry, this limited-time offer won't last long!
We Accept
- Key Concepts in Measures of Association
- Steps to Solving Measures of Association Assignments
- Step 1: Understand the Objective
- Step 2: Import the Dataset
- Step 3: Calculate Measures of Association
- Step 4: Interpret the Results
- Step 5: Report Your Findings
- Step 6: Provide Detailed Interpretation
- Additional Tips
- Conclusion
In the realm of statistical analysis, understanding the intricate relationship between variables is paramount. Variables often interact in complex ways, influencing outcomes and shaping our understanding of the world around us. Central to this understanding are measures of association, which serve as powerful tools for unraveling the connections between different variables. In this exploration, we delve deep into the realm of measures of association, unveiling their significance and shedding light on their application in statistical analysis. By uncovering these measures, we aim to provide clarity and insight into the intricate web of relationships that exist within datasets, ultimately empowering researchers to draw meaningful conclusions and make informed decisions based on their findings. This guide will offer essential help with your statistics assignment, ensuring you grasp the importance and application of these measures in your analyses.
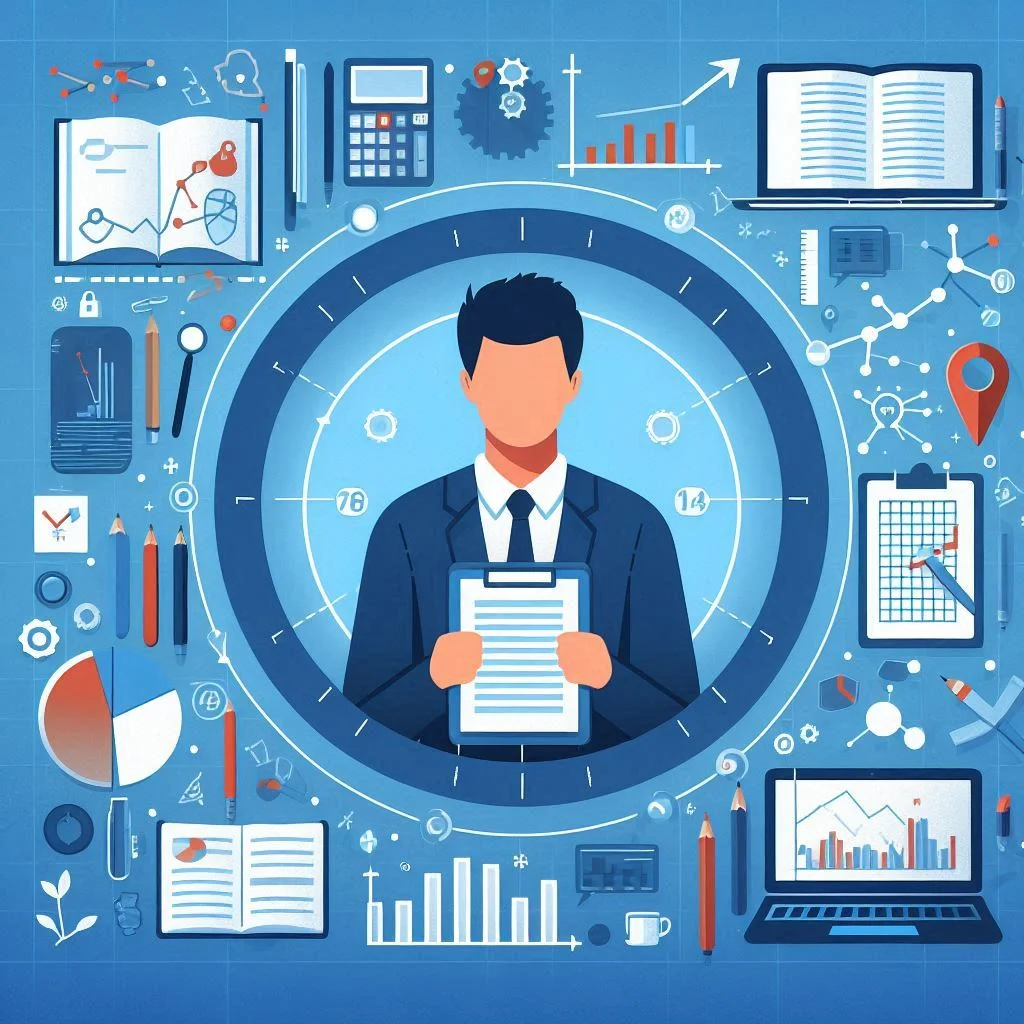
Measures of association serve as the compass in the vast landscape of statistical analysis, guiding researchers through the maze of data and illuminating the pathways between variables. From risk differences to odds ratios, these measures offer a comprehensive view of the strength and direction of associations, enabling researchers to discern patterns, identify trends, and make sense of complex datasets. Through this exploration, we embark on a journey to demystify these measures, unveiling their utility and relevance in various domains, from public health to social sciences. By shedding light on the nuances of measures of association, we equip researchers with the tools they need to navigate the intricacies of statistical analysis and uncover meaningful insights hidden within their data.
Key Concepts in Measures of Association
Before diving into the steps, it’s essential to understand some fundamental concepts:
- Risk Difference (RD): The absolute difference in risk between two groups.
- Risk Ratio (RR): The ratio of the risk of an event occurring in the exposed group to the risk in the unexposed group.
- Rate Ratio (IRR): The ratio of incidence rates between two groups.
- Odds Ratio (OR):The ratio of the odds of an event occurring in one group to the odds of it occurring in another group.
- Confidence Intervals (CI): A range of values that is likely to contain the true measure of association, usually reported at a 95% confidence level.
Steps to Solving Measures of Association Assignments
Step 1: Understand the Objective
Read the assignment carefully to understand what is required. Typically, you will need to calculate and interpret various measures of association and their confidence intervals using a given dataset. Ensure you grasp the context and the variables involved.
Step 2: Import the Dataset
Using Stata or any other statistical software, import the provided dataset. Here’s a brief on how to do it in Stata:
Import the Data: Use the import excel command if your data is in Excel format.
stata
import excel "path/to/your/dataset.xlsx", sheet("Data") firstrow clear
- Explore the Data:Familiarize yourself with the dataset by using commands like list, describe, and summarize.
Step 3: Calculate Measures of Association
For calculating measures of association in Stata, you will typically use commands like tabulate, ci, cc, and logistic. Here’s an example workflow:
Calculate Risk Difference and Risk Ratio:
stata
cs high_bp exposure_variable
- This command will give you the risk difference and risk ratio between groups with high blood pressure and the exposure variable (e.g., traditional cookstove usage).
Calculate Rate Ratio (Incidence Rate Ratio):
stata
stset time_variable, failure(event_variable)
stcox exposure_variable
- This is used for survival analysis, which can help in calculating the rate ratios.
Calculate Odds Ratio:
stata
logistic high_bp exposure_variable
- This command provides the odds ratio for the association between high blood pressure and the exposure variable.
Step 4: Interpret the Results
Once you have your measures, interpret them in the context of your study. For example:
- Risk Difference (RD):If RD is 0.05, it means there is a 5% higher risk in the exposed group compared to the unexposed group.
- Risk Ratio (RR): If RR is 1.5, the exposed group has a 50% higher risk of the outcome compared to the unexposed group.
- Rate Ratio (IRR): If IRR is 2.0, the incidence rate in the exposed group is twice that of the unexposed group.
- Odds Ratio (OR): If OR is 3.0, the odds of the outcome are three times higher in the exposed group.
Step 5: Report Your Findings
Create a table to report your findings, including estimates and 95% confidence intervals. Here’s a template:
Measure | Estimate | Lower 95% CI | Upper 95% CI |
---|---|---|---|
Risk Difference | 0.05 | 0.02 | 0.08 |
Risk Ratio | 1.5 | 1.2 | 1.8 |
Rate Ratio | 2.0 | 1.6 | 2.5 |
Odds Ratio | 3.0 | 2.0 | 4.5 |
Step 6: Provide Detailed Interpretation
For each measure, provide a detailed interpretation. For instance:
- Risk Difference:The risk of high blood pressure is 5% higher in households using traditional cookstoves.
- Risk Ratio: Households using traditional cookstoves are 1.5 times more likely to have high blood pressure.
- Rate Ratio: The incidence rate of high blood pressure is twice as high in the exposed group.
- Odds Ratio:The odds of high blood pressure are three times higher in households using traditional cookstoves.
Additional Tips
- Review Literature: To enhance your understanding, review related literature on measures of association. This will give you insights into common pitfalls and advanced interpretation techniques.
- Practice with Data: The more you practice with different datasets, the more proficient you will become. Try to work on datasets from various domains to broaden your experience.
- Use Stata Help and Tutorials: Stata provides extensive help files and tutorials. Use commands like help cs, help stcox, and help logistic to explore more.
Conclusion
In conclusion, navigating the intricacies of statistical measures of association is integral to understanding the relationships between variables in research. Through this exploration, we've shed light on the significance of measures like risk difference, risk ratio, rate ratio, and odds ratio in quantifying these associations. By comprehensively analyzing data sets and interpreting the results, researchers gain valuable insights into the factors influencing various outcomes, from health conditions to social phenomena. This understanding not only enhances the quality of research but also informs evidence-based decision-making in fields such as public health, epidemiology, and sociology.
Moreover, delving into measures of association extends beyond mere calculation; it involves a nuanced interpretation that considers context, significance, and implications. By critically evaluating findings and discerning patterns in statistical outputs, researchers can draw meaningful conclusions that contribute to the advancement of knowledge and inform policy and practice. Thus, this journey through statistical measures of association serves not only to deepen our understanding of research methodologies but also to underscore the vital role of statistical analysis in elucidating complex relationships in the world around us.