Claim Your Offer
Unlock a fantastic deal at www.statisticsassignmenthelp.com with our latest offer. Get an incredible 10% off on all statistics assignment, ensuring quality help at a cheap price. Our expert team is ready to assist you, making your academic journey smoother and more affordable. Don't miss out on this opportunity to enhance your skills and save on your studies. Take advantage of our offer now and secure top-notch help for your statistics assignments.
We Accept
- Unraveling the Basics of the Kruskal-Wallis Test
- Introduction to Non-Parametric Tests
- When to Use the Kruskal-Wallis Test
- Hypotheses in the Kruskal-Wallis Test
- Assumptions and Limitations
- Conducting the Kruskal-Wallis Test: Step-by-Step Guide
- Data Preparation and Organization
- Calculation of the Test Statistic
- Interpretation of Results
- Reporting and Visualization
- Practical Tips and Common Pitfalls
- Check Your Data Distribution
- Beware of Sample Sizes
- Post Hoc Tests for Detailed Insights
- Conclusion
Statistics can often feel like navigating a complex labyrinth of formulas and methodologies, leaving students feeling overwhelmed and uncertain. Among the multitude of statistical tests, the Kruskal-Wallis test stands out as a versatile and powerful tool, especially when dealing with real-world data that doesn't conform to the assumptions of parametric tests. In this comprehensive guide tailored specifically for students, we embark on a journey to demystify the intricacies of the Kruskal-Wallis test. By breaking down its fundamental concepts, applications, and practical tips, this guide aims to empower students with the knowledge and confidence they need to excel in their Kruskal-Wallis Test assignment.
Understanding the Kruskal-Wallis test begins with grasping the essence of non-parametric statistics. Unlike their parametric counterparts, non-parametric tests like Kruskal-Wallis make minimal assumptions about the data, allowing for a broader applicability across diverse datasets. Through this guide, students will delve into the nuances of the Kruskal-Wallis test, learning when and how to implement it effectively. By equipping students with a clear understanding of hypotheses formulation, result interpretation, and potential pitfalls, this guide serves as a beacon, illuminating the path to statistical proficiency. With this knowledge in hand, students can confidently navigate the complexities of the Kruskal-Wallis test, transforming their statistical endeavors into insightful and meaningful analyses.
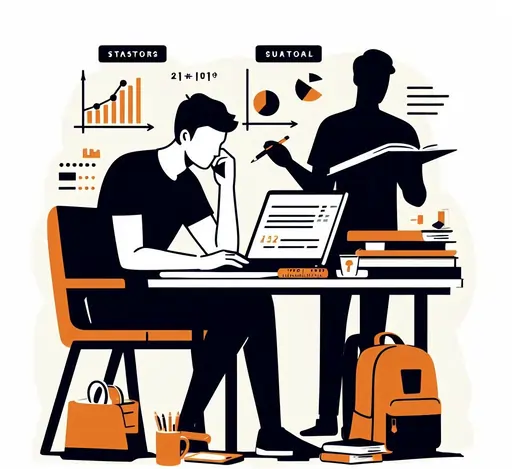
Unraveling the Basics of the Kruskal-Wallis Test
At the heart of statistical analysis lies the Kruskal-Wallis test, a powerful non-parametric tool designed to discern significant differences among multiple groups. To truly grasp its significance, students first need to comprehend the broader realm of non-parametric tests. Unlike their parametric counterparts, which hinge on specific data distribution assumptions, non-parametric tests, including the Kruskal-Wallis test, offer flexibility, making them indispensable when analyzing datasets with diverse and often unpredictable structures. The Kruskal-Wallis test, in particular, steps in when there are more than two groups involved, allowing researchers to explore variations in medians without demanding conformity to the constraints of normal distribution. By understanding these fundamental concepts, students can not only decipher the complexities of the Kruskal-Wallis test but also appreciate its versatility, paving the way for more nuanced and accurate statistical analyses in their academic pursuits.
Introduction to Non-Parametric Tests
To truly understand the Kruskal-Wallis test, it's essential to grasp the concept of non-parametric tests. Unlike parametric tests, which assume that the data follows a specific distribution (usually normal), non-parametric tests make minimal assumptions about the data. This flexibility makes non-parametric tests, including the Kruskal-Wallis test, invaluable when dealing with real-world datasets that might not meet the stringent assumptions of parametric tests.
When to Use the Kruskal-Wallis Test
The Kruskal-Wallis test is particularly useful when you have more than two groups and you want to determine whether there are any statistically significant differences among the groups. It's an extension of the Mann-Whitney U test, allowing you to compare multiple groups simultaneously. This test is ideal for scenarios where the data is ordinal, interval, or ratio, but not normally distributed.
Hypotheses in the Kruskal-Wallis Test
In the Kruskal-Wallis test, just like in any statistical analysis, formulating clear hypotheses is crucial. The null hypothesis (H0 ) posits that there are no significant differences among the groups, while the alternative hypothesis (H1 ) suggests that at least one group significantly differs from the others. Understanding and articulating these hypotheses accurately is the first step towards a successful Kruskal-Wallis test.
Assumptions and Limitations
While the Kruskal-Wallis test is robust against many assumptions, it's essential to be aware of its limitations. One limitation is that it assumes the groups have similar shapes of distributions. Additionally, it doesn't identify which specific group(s) differ; it only tells you that there is a significant difference somewhere among the groups. Understanding these limitations helps you interpret the results more effectively.
Conducting the Kruskal-Wallis Test: Step-by-Step Guide
Conducting the Kruskal-Wallis test requires a systematic approach, and understanding each step is pivotal for accurate analysis. The journey commences with meticulous data organization, where disparate groups are defined and data is structured for comparison. Once the groundwork is set, the calculation of the test statistic takes center stage. This involves ranking the combined data, computing average ranks for each group, and deriving the essential test statistic. As students embark on this process, attention to detail is paramount. Proper interpretation of results follows, where the calculated test statistic and its corresponding p-value unveil the presence of significant differences among the groups. Armed with this knowledge, students can confidently report their findings, employing visualization tools to enhance the clarity of their results. Through this step-by-step guide, students gain not just the technical prowess to conduct the Kruskal-Wallis test but also the confidence to apply this valuable statistical tool to real-world scenarios, making their assignments not just tasks to complete but opportunities for meaningful analysis and insights.
Data Preparation and Organization
Before diving into the Kruskal-Wallis test, you need to ensure your data is organized correctly. Arrange your dataset into groups, with each group representing a distinct category or treatment. Remember, the Kruskal-Wallis test compares medians, so ensure your data is ordinal, interval, or ratio.
Calculation of the Test Statistic
The Kruskal-Wallis test involves ranking the combined data from all groups, calculating the average rank for each group, and then using these ranks to compute the test statistic. This test statistic follows a chi-square (χ2 ) distribution, enabling you to determine the p-value. The smaller the p-value, the stronger the evidence against the null hypothesis.
Interpretation of Results
Once you have your test statistic and corresponding p-value, it's time to interpret the results. If the p-value is below your chosen significance level (commonly set at 0.05), you can reject the null hypothesis. This rejection indicates that there is a significant difference among at least one pair of groups. Remember, you might need to perform post hoc tests to pinpoint these specific differences, depending on your research questions.
Reporting and Visualization
In your statistics assignments, clear reporting is as crucial as the analysis itself. Present your findings concisely, including the test statistic, degrees of freedom, and the p-value. Additionally, using visual aids like box plots or bar charts can enhance the clarity of your results, making it easier for your audience to grasp the differences among the groups.
Practical Tips and Common Pitfalls
Navigating the realm of statistical analysis, especially when dealing with non-parametric tests like the Kruskal-Wallis, requires a keen eye for detail and an awareness of potential challenges. One crucial practical tip is to meticulously assess your data's distribution. While the Kruskal-Wallis test is robust against deviations from normality, extreme departures might still impact the results. Employ visual aids such as histograms and normality tests to gauge the data's distribution accurately. Additionally, keep a watchful eye on sample sizes; in smaller datasets, skewed rank sums can distort outcomes, emphasizing the importance of considering sample sizes while interpreting results. Another key aspect is understanding the significance of post hoc tests. When the Kruskal-Wallis test highlights significant differences among groups, employing post hoc tests becomes vital. These tests, such as Dunn's test or Conover's test, unravel specific group disparities, providing a nuanced perspective. By embracing these practical tips and being mindful of common pitfalls, students can enhance the precision of their analyses, ensuring robust and insightful interpretations of their data.
Check Your Data Distribution
Before opting for the Kruskal-Wallis test, examine your data distribution. While this test is robust against deviations from normality, extreme deviations might still affect the results. Visualization tools like histograms and normality tests can assist you in this initial assessment.
Beware of Sample Sizes
In smaller sample sizes, the Kruskal-Wallis test might not provide accurate results. Small samples can lead to skewed rank sums and affect the overall outcome. Always consider the sample sizes of your groups and, if necessary, explore alternative methods if your sample sizes are too small.
Post Hoc Tests for Detailed Insights
If your Kruskal-Wallis test yields a significant result, consider performing post hoc tests (e.g., Dunn's test or Conover's test) to identify specific group differences. These tests can be invaluable in deciphering complex datasets with multiple groups, providing a more nuanced understanding of the relationships between variables.
Conclusion
In the realm of statistics, the Kruskal-Wallis test stands as a stalwart tool for comparing multiple groups without the constraints of normal distribution. Armed with a solid understanding of its principles, applications, and nuances, you can confidently tackle your statistics assignments. Remember, practice and persistence are key; the more you engage with this test, the more adept you'll become at deciphering complex datasets and drawing meaningful conclusions. So, embrace the Kruskal-Wallis test, and let it empower your statistical endeavors, paving the way for academic success and a deeper understanding of the intricate world of statistics.