Avail Your Offer Now
Celebrate the festive season with an exclusive holiday treat! Enjoy 15% off on all orders at www.statisticsassignmenthelp.com this Christmas and New Year. Unlock expert guidance to boost your academic success at a discounted price. Use the code SAHHOLIDAY15 to claim your offer and start the New Year on the right note. Don’t wait—this special offer is available for a limited time only!
We Accept
- What is a P-Value?
- Interpreting P-Values in Regression Output
- The Role of P-Values in Decision-Making
- Limitations of P-Values
- Real-Life Application of P-Values in Regression
- Conclusion
When tackling regression analysis, one of the key concepts you'll encounter is the p-value. This statistical measure is crucial for understanding the significance of your model's variables and plays a vital role in determining whether your findings are robust and reliable. If you're looking to solve your regression analysis assignment, grasping the concept of p-values is essential. A p-value helps you evaluate whether the relationships identified in your model are likely to be genuine or if they might have occurred by random chance.
In simple terms, the p-value assesses the probability that the observed effect in your data could have happened if there were no actual relationship between the variables. This makes it a fundamental component in validating the results of your regression model. For instance, a low p-value indicates strong evidence against the null hypothesis, suggesting that the independent variable has a significant impact on the dependent variable. Conversely, a high p-value implies that there is insufficient evidence to support a meaningful relationship.
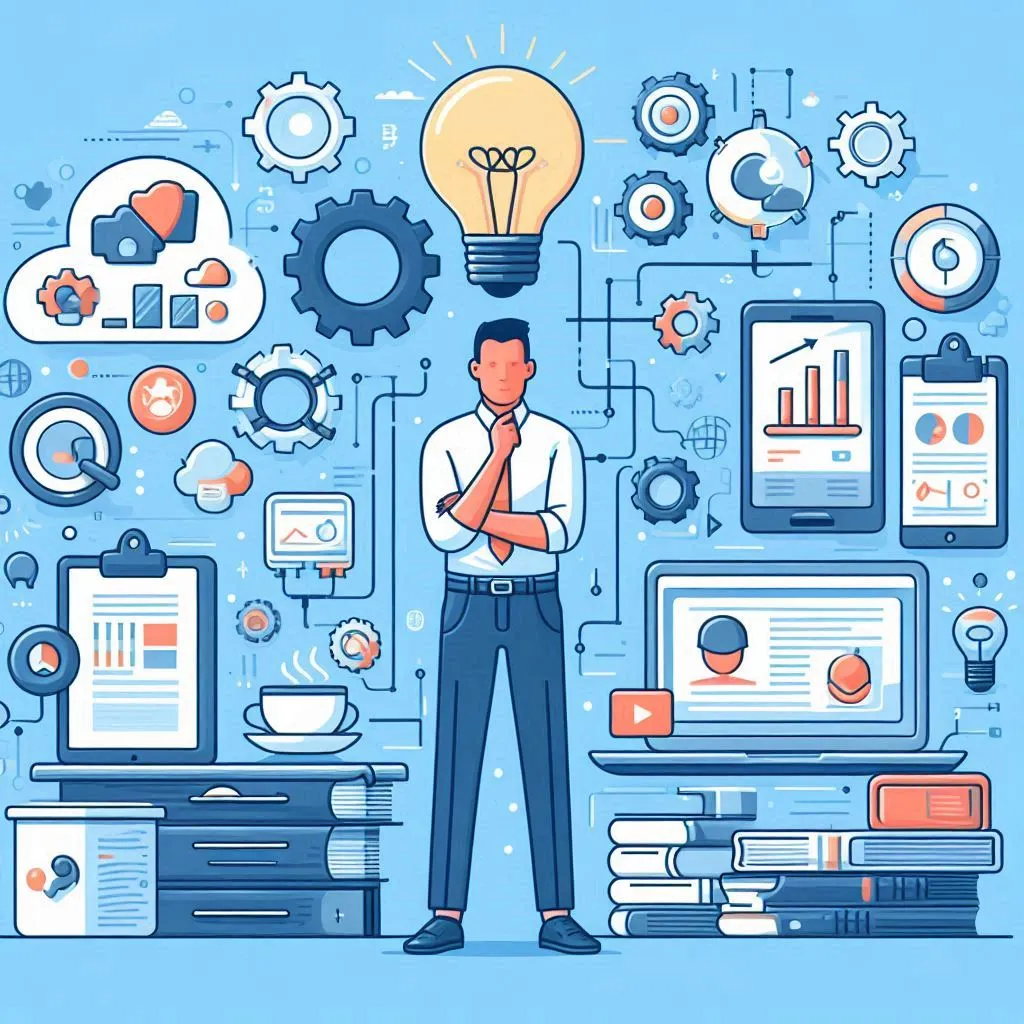
Understanding how to interpret p-values can significantly enhance your ability to analyze and present your regression results. It not only helps in identifying which variables are most influential but also guides decisions on which variables to include in your final model.
What is a P-Value?
A p-value is a statistical measure that helps you determine whether your data supports your hypothesis. In the context of regression analysis, the p-value indicates whether the relationship between an independent variable (predictor) and the dependent variable (outcome) is statistically significant.
In simpler terms, the p-value tells you how likely it is that any observed relationship between variables happened by chance. A lower p-value suggests that the relationship is not just a fluke and that your independent variable truly has an effect on your dependent variable.
Interpreting P-Values in Regression Output
When you perform regression analysis, you'll typically receive an output table that includes the p-values for each of the independent variables in your model. Here's how to interpret them:
- Significance Threshold: By convention, a p-value of less than 0.05 is often considered statistically significant. This means that there is less than a 5% chance that the relationship between the variables occurred by random chance. However, this threshold can vary depending on the field of study or the specific context of the analysis.
- High P-Value: If the p-value is greater than 0.05, the relationship between the variables is considered statistically insignificant. This means that you cannot confidently say that the independent variable has an effect on the dependent variable. For example, if you’re analyzing the effect of education level on income and the p-value for education is 0.08, it would suggest that education level does not significantly impact income in your model.
- Low P-Value: A p-value less than 0.05 indicates statistical significance, meaning you can reject the null hypothesis (which states that there is no relationship between the variables). For instance, if you find a p-value of 0.03 for the effect of work experience on salary, you can conclude that work experience has a significant impact on salary.
- P-Value Near Zero: A p-value that is extremely close to zero (e.g., 0.001) suggests a very strong relationship between the variables. In such cases, the likelihood that the relationship is due to chance is exceptionally low. This indicates a very high level of confidence in the results.
The Role of P-Values in Decision-Making
P-values play a critical role in decision-making, especially when it comes to determining which variables to include in your final regression model. Here's how they influence the process:
- Variable Selection: In regression analysis, you might start with a model that includes multiple independent variables. P-values help you decide which of these variables are significant enough to retain in the model. Variables with high p-values (e.g., greater than 0.05) may be excluded, as they do not significantly contribute to explaining the variation in the dependent variable.
- Model Refinement: As you refine your regression model, p-values guide you in assessing the strength of the relationships between variables. By focusing on variables with low p-values, you can create a more precise model that better explains the dependent variable.
- Understanding Relationships: Beyond just building a model, p-values help you understand the underlying relationships in your data. For example, if you’re analyzing the impact of various factors on house prices, p-values allow you to determine which factors (e.g., location, square footage, age of the house) are genuinely influencing prices and which are not.
Limitations of P-Values
While p-values are powerful, they do have limitations. It's essential to be aware of these to avoid over-relying on them in your analysis:
- Sample Size Sensitivity: P-values can be sensitive to sample size. In large datasets, even trivial relationships might appear statistically significant (i.e., low p-value), while in small datasets, important relationships might be missed due to a higher p-value.
- Not a Measure of Effect Size: A p-value tells you whether a relationship exists, but it doesn’t tell you the strength of that relationship. For instance, a small p-value might indicate significance, but the effect size (i.e., the actual impact of the independent variable on the dependent variable) could still be minimal.
- Dependence on Thresholds: The conventional threshold of 0.05 is somewhat arbitrary. In different contexts, more stringent thresholds (e.g., 0.01) might be appropriate, especially in fields like medicine, where false positives can have serious consequences.
- Multiple Comparisons Problem: When testing multiple hypotheses simultaneously, the chances of finding at least one statistically significant result due to chance increase. This is known as the multiple comparisons problem, and it can lead to misleading conclusions if not properly accounted for.
Real-Life Application of P-Values in Regression
To illustrate the importance of p-values, consider a scenario in the field of economics. Suppose a researcher is studying the factors that influence consumer spending. They include variables such as income, education level, and interest rates in their regression model.
- Income: The p-value for income might be extremely low (e.g., 0.001), indicating a strong and significant relationship between income and consumer spending. This would suggest that as income increases, consumer spending is likely to increase as well.
- Education Level: If the p-value for education level is high (e.g., 0.2), the researcher might conclude that education level does not have a significant direct impact on consumer spending in their model.
- Interest Rates: A moderate p-value (e.g., 0.04) for interest rates could indicate a significant, though less strong, relationship with consumer spending. This might lead the researcher to explore this variable further or consider it alongside other factors.
Conclusion
P-values are a fundamental aspect of regression analysis, offering crucial insights into the significance of relationships between variables. Understanding how to interpret p-values can greatly help with statistics assignment, allowing you to make informed decisions about which variables to include in your model and refine your analysis. By evaluating p-values, you can draw meaningful conclusions from your data and enhance the accuracy of your results. However, it's essential to be aware of their limitations and use them alongside other statistical measures. This balanced approach ensures that you are well-equipped to analyze data effectively and uncover the true nature of the relationships within it.