Avail Your Offer Now
Celebrate the festive season with an exclusive holiday treat! Enjoy 15% off on all orders at www.statisticsassignmenthelp.com this Christmas and New Year. Unlock expert guidance to boost your academic success at a discounted price. Use the code SAHHOLIDAY15 to claim your offer and start the New Year on the right note. Don’t wait—this special offer is available for a limited time only!
We Accept
- Understanding the Basics of Text Analytics
- Decoding Unstructured Data
- Key Components of Text Analytics
- Unraveling the Magic of Natural Language Processing (NLP)
- Bridging the Gap Between Humans and Machines
- Statistical Approaches for Assignment Solving
- Machine Learning in Text Analytics
- Practical Tips for Students
- Embrace User-Friendly Tools
- Continuous Learning and Exploration
- Conclusion
In the ever-evolving landscape of technology, the significance of harnessing unstructured data has become increasingly evident. With the proliferation of information in the digital age, students find themselves grappling with assignments that are not only extensive but also brimming with unorganized and voluminous textual information. The complexity of these tasks often leaves students overwhelmed, searching for effective strategies to make sense of the data at hand.
Enter Text Analytics and Natural Language Processing (NLP), the unsung heroes in the realm of academic challenges. These formidable tools offer more than just a lifeline; they provide robust statistical approaches to untangle the intricacies embedded in unstructured data. As we embark on this exploration, our focus is not just on helping students decode assignments but also on empowering them to elevate the overall quality of their submissions.
In this blog, we delve into the transformative capabilities of Text Analytics and NLP, demonstrating how students can harness these technological marvels to navigate through the labyrinth of unstructured data with finesse. From unraveling the basics to deploying advanced statistical approaches, our journey aims to equip students with the knowledge and skills needed to transform their assignment-solving endeavors. Let's embark on this enlightening voyage into the world where Text Analytics and NLP redefine the boundaries of academic achievement. If you find yourself seeking assistance with your Statistics assignment, these tools can be valuable allies in your academic journey.
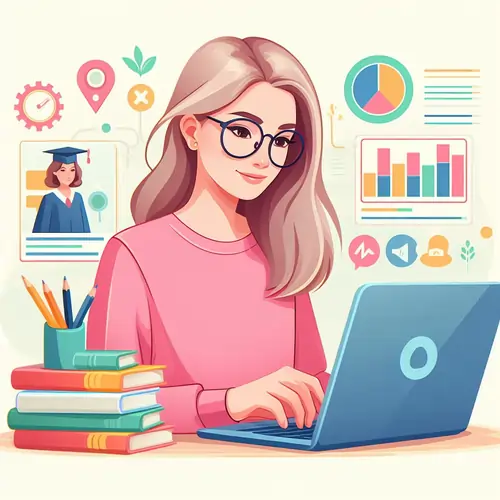
Understanding the Basics of Text Analytics
Embarking on the captivating journey of harnessing Text Analytics for assignment solving requires a solid grasp of foundational concepts. Text Analytics acts as the indispensable compass that steers students through the expansive sea of unstructured data. In the intricate process of decoding the basics, such as tokenization and sentiment analysis, students acquire the transformative ability to disentangle the complexity inherent in assignments. This skillset empowers them to break down the textual intricacies into manageable components, unlocking a deeper comprehension of the content.
Tokenization, a cornerstone of Text Analytics, involves dissecting the raw text into smaller units, or tokens. This meticulous dissection facilitates a granular examination, allowing students to discern patterns and extract nuanced meanings. Moreover, as students navigate the terrain of sentiment analysis, they develop a heightened sensitivity to the emotional undercurrents within the text. Understanding the emotional tone becomes a valuable tool, especially when assignments require an exploration of subjective viewpoints or opinions.
As the journey unfolds, these fundamental skills become the bedrock upon which a profound understanding of the content is constructed. Armed with the capability to deconstruct assignments into comprehensible fragments, students lay the groundwork for effective analysis and interpretation. This not only streamlines the assignment-solving process but also enhances the quality of insights derived from the textual tapestry.
Decoding Unstructured Data
Before delving into the intricacies of Text Analytics, it's crucial to grasp the concept of unstructured data. Assignments often come in the form of lengthy paragraphs, articles, or research papers, making it challenging for students to extract meaningful insights. Text Analytics, however, equips students with the ability to convert this unstructured data into structured information, facilitating a deeper understanding of the content.
Key Components of Text Analytics
Text Analytics involves several key components, each playing a pivotal role in the process of converting raw text into valuable insights. These components include:
- Tokenization
- Sentiment Analysis
Tokenization is the process of breaking down text into smaller units, such as words or phrases, known as tokens. This step is essential for further analysis as it allows the system to understand the context of each unit independently.
Sentiment analysis gauges the emotional tone expressed in the text. This can be particularly useful for assignments that require an understanding of the author's perspective or opinions, adding a layer of depth to the analysis.
Unraveling the Magic of Natural Language Processing (NLP)
In the fascinating domain of Natural Language Processing (NLP), a captivating journey unfolds as machines delve into the intricate nuances of human language. NLP serves as a key that unlocks unexplored dimensions in the realm of assignment solving, offering students a transformative toolset. As we embark on the enchanting exploration of NLP, its role as a seamless bridge between human communication and computational understanding becomes increasingly evident.
Named Entity Recognition (NER) emerges as a potent wand in the magician's arsenal, capable of identifying and categorizing entities within the labyrinth of text. This magical capability proves invaluable when assignments demand the extraction of specific information about names, organizations, or locations, elevating the precision and depth of analysis.
Bridging the Gap Between Humans and Machines
Natural Language Processing (NLP) serves as the bridge between human language and computer understanding. When confronted with a text-heavy assignment, NLP enables students to interact with the content in a manner that goes beyond mere comprehension. Here's how:
- Named Entity Recognition (NER)
- Language Translation
NER is a subset of NLP that identifies and categorizes entities within the text, such as names, organizations, and locations. This can be a game-changer when assignments involve extracting information about specific entities.
For assignments that involve multilingual content, NLP can be used for language translation. This not only aids in understanding the text but also broadens the scope of research by accessing information in different languages.
Statistical Approaches for Assignment Solving
In the ever-evolving landscape of assignment solving, the application of statistical approaches, especially those deeply intertwined with machine learning, stands out as a beacon of guidance for students navigating through the intricacies of unstructured data. As the academic terrain becomes more data-centric, the importance of mastering these statistical methodologies cannot be overstated.
Text classification and clustering, within the realm of statistical approaches, emerge as indispensable tools, equipping students with the prowess to not just comprehend but strategically organize voluminous information. Text classification involves the systematic categorization of textual content, allowing students to effortlessly sift through diverse data and allocate it into predefined categories. On the other hand, clustering, another statistical gem, facilitates the grouping of similar information, unveiling patterns and relationships that might not be immediately apparent.
By delving into the nuances of these statistical approaches, students not only bolster their analytical prowess but also cultivate a structured framework for approaching assignments methodically. The mastery of these tools empowers students to unravel the complexities of assignments with confidence, transforming what once seemed like an overwhelming sea of data into a navigable landscape of insights and understanding. As the symbiotic relationship between education and technology deepens, proficiency in these statistical approaches becomes not just an academic advantage but a key competency for success in the data-driven professional world of the future. So, let's embark on a journey through the statistical realm, where each algorithm is a compass guiding students toward assignment-solving excellence.
Machine Learning in Text Analytics
Machine Learning (ML) algorithms play a crucial role in the statistical approaches adopted in Text Analytics. These algorithms learn from patterns and structures within the data, enabling them to make predictions or categorize information. In the context of assignments, ML can be applied in various ways:
- Text Classification
- Clustering
Text classification involves categorizing text into predefined categories. This can be immensely helpful when assignments require sorting information into distinct topics or themes.
Clustering involves grouping similar pieces of text together based on patterns and similarities. This approach is beneficial when dealing with large volumes of unstructured data, helping students identify common threads and themes.
Practical Tips for Students
Navigating the landscape of Text Analytics and NLP requires not just theoretical knowledge but also practical wisdom. In this section, we offer invaluable tips to students, acting as their digital compass in the vast sea of information. From embracing user-friendly tools like NLTK and spaCy to advocating for continuous learning and exploration, these practical insights equip students with the skills and mindset needed to confidently navigate through the dynamic world of text analysis. As students incorporate these tips into their academic arsenal, they not only solve assignments more efficiently but also cultivate a lifelong learning mindset in the ever-evolving field of technology.
Embrace User-Friendly Tools
In the dynamic and ever-evolving landscape of Text Analytics and Natural Language Processing (NLP), an array of user-friendly tools awaits students, catering to diverse levels of technical expertise. These tools not only simplify the complexities of unstructured data but also empower students to tackle assignments with confidence. Among these, NLTK (Natural Language Toolkit), spaCy, and TextBlob stand out as invaluable allies in the assignment-solving journey. NLTK, with its comprehensive suite of libraries, provides a robust foundation for tasks such as tokenization and sentiment analysis. spaCy, known for its efficiency, offers advanced capabilities like Named Entity Recognition (NER), enhancing the precision of information extraction. TextBlob, a simplified library, serves as an accessible entry point for beginners, facilitating a seamless initiation into the realm of text analytics.
Continuous Learning and Exploration
The field of Text Analytics and NLP is a dynamic ecosystem, continually pushing boundaries and evolving. To navigate this ever-changing landscape effectively, students are not merely encouraged but urged to stay abreast of the latest developments. This involves active participation in workshops, where hands-on experiences can deepen their understanding of emerging techniques. Engaging in online forums and communities dedicated to NLP fosters a collaborative environment, providing a platform for sharing insights, troubleshooting challenges, and discovering novel approaches. As students immerse themselves in continuous learning and exploration, they cultivate a mastery of these technologies, enabling them to navigate through assignments with not just efficiency but a profound understanding of the cutting-edge methodologies shaping the field.
Conclusion
In conclusion, the symbiotic relationship between Text Analytics and Natural Language Processing (NLP) unfolds an unparalleled opportunity for students to triumph over the challenges presented by assignments rich in unstructured data. By delving deeper into the basics of Text Analytics, unraveling the enchanting capabilities of NLP, strategically adopting statistical approaches, and wholeheartedly embracing practical tips, students can not only decode assignments with heightened efficiency but also significantly enhance the caliber of their analyses. As we hurtle further into the digital era, these acquired skills transcend the confines of academic settings; they metamorphose into invaluable assets within the professional realm. Thus, I fervently encourage students to immerse themselves in the vast expanse of Text Analytics and NLP, poised to witness the transformative evolution of their assignment-solving endeavors.