Avail Your Offer Now
Celebrate the festive season with an exclusive holiday treat! Enjoy 15% off on all orders at www.statisticsassignmenthelp.com this Christmas and New Year. Unlock expert guidance to boost your academic success at a discounted price. Use the code SAHHOLIDAY15 to claim your offer and start the New Year on the right note. Don’t wait—this special offer is available for a limited time only!
We Accept
- Understanding the Importance of Biostatistics in Public Health
- The Role of Biostatistics in Public Health
- Applications of Biostatistics in Public Health Assignments
- Key Biostatistics Techniques for Public Health
- Descriptive Statistics
- Measures of Central Tendency
- Measures of Dispersion
- Inferential Statistics
- Hypothesis Testing
- Confidence Intervals
- Advanced Statistical Methods
- Regression Analysis
- Linear Regression
- Logistic Regression
- Survival Analysis
- Kaplan-Meier Estimator
- Cox Proportional Hazards Model
- Practical Steps for Public Health Assignments
- Data Preparation
- Data Cleaning
- Variable Creation
- Data Analysis
- Descriptive Analysis
- Inferential Analysis
- Reporting Results
- Writing the Report
- Creating Appendices
- Conclusion
Biostatistics plays a crucial role in public health by providing the tools necessary to analyze data and draw meaningful conclusions. This blog will guide you through essential biostatistics techniques to help you solve assignments, particularly those involving the investigation of health outcomes like cholesterol levels in relation to socioeconomic variables and dietary intake. For those seeking help with biostatistics assignments, mastering these techniques can significantly enhance your ability to handle similar public health assignments effectively. The focus will be on understanding the importance of biostatistics, key biostatistical techniques, advanced statistical methods, and practical steps for public health assignments. Whether you are a student or a public health professional, this comprehensive guide will equip you with the knowledge and skills needed to analyze complex data, make informed decisions, and contribute to public health research. Ultimately, by mastering these biostatistical techniques, you will be better prepared to tackle various public health challenges and improve health outcomes on a broader scale.
Understanding the Importance of Biostatistics in Public Health
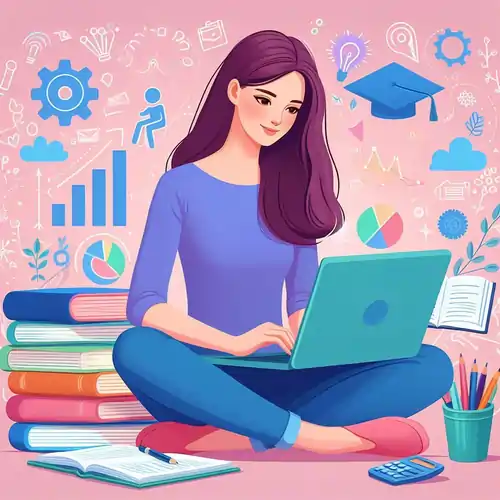
Biostatistics is essential in understanding and addressing various public health issues. By applying statistical methods to health data, public health professionals can identify trends, assess risks, and develop effective interventions. This field plays a crucial role in designing studies, analyzing data, and interpreting results, which are fundamental for evidence-based decision-making. Biostatistics helps in tracking disease outbreaks, evaluating the effectiveness of public health programs, and identifying health disparities among different populations. It also aids in predicting health trends and outcomes, allowing for proactive measures to be implemented. Furthermore, biostatistical analysis is vital for validating research findings and ensuring the reliability of health information. This section will discuss the role and applications of biostatistics in public health, providing a foundation for the techniques discussed later. Understanding these applications will help you approach your assignments with a clear framework and apply the appropriate statistical methods to derive meaningful insights.
The Role of Biostatistics in Public Health
Biostatistics is essential for public health research as it allows for the systematic collection, analysis, and interpretation of health data. This field supports evidence-based decision-making by providing statistical methods to understand and address health issues. In public health, biostatistics is used to:
- Design studies and surveys that collect relevant health data.
- Analyze the relationship between health outcomes and various risk factors.
- Evaluate the effectiveness of public health interventions and policies.
By integrating biostatistical methods into public health research, professionals can derive insights that lead to improved health outcomes and policies.
Applications of Biostatistics in Public Health Assignments
When dealing with public health assignments, biostatistics helps in:
- Identifying trends and patterns in health data.
- Assessing the impact of socioeconomic and environmental factors on health.
- Predicting health outcomes and modeling disease spread.
- Designing and analyzing clinical trials and epidemiological studies.
Understanding these applications will help you approach your assignments with a clear framework and apply the appropriate statistical methods. These applications are crucial for translating data into actionable public health strategies.
Key Biostatistics Techniques for Public Health
Understanding the key biostatistical techniques is essential for analyzing public health data effectively. This section will cover descriptive statistics and inferential statistics, providing a basis for more advanced methods.
Descriptive Statistics
Descriptive statistics summarize and describe the main features of a dataset. They provide a simple overview of the data, which is essential for initial analysis.
Measures of Central Tendency
Measures of central tendency include the mean, median, and mode. These metrics help identify the typical value in a dataset:
- Mean:The average of all data points. It is sensitive to extreme values (outliers).
- Median:The middle value when the data is ordered. It is less affected by outliers.
- Mode: The most frequently occurring value in the dataset.
These measures help summarize the data, making it easier to understand the distribution of values in the dataset.
Measures of Dispersion
Measures of dispersion indicate the spread or variability of the data:
- Range:The difference between the highest and lowest values.
- Variance: The average squared deviation from the mean.
- Standard Deviation (SD): The square root of the variance, indicating the average distance from the mean.
These measures help you understand the distribution and variability of the data, which is crucial for further analysis. Understanding dispersion is essential for identifying potential outliers and the overall spread of the data.
Inferential Statistics
Inferential statistics allow you to make inferences and draw conclusions about a population based on a sample.
Hypothesis Testing
Hypothesis testing assesses the evidence against a null hypothesis. Common tests include:
- T-tests: Compare the means of two groups (e.g., comparing cholesterol levels between different dietary groups).
- Chi-square tests: Assess the association between categorical variables (e.g., the relationship between race and cholesterol levels).
These tests help determine if observed differences are statistically significant, providing a basis for further research or policy recommendations.
Confidence Intervals
Confidence intervals provide a range of values within which the true population parameter is likely to lie. They give an estimate of the uncertainty around the sample statistic.
Using these inferential techniques, you can determine whether observed differences or associations are statistically significant. Confidence intervals offer a clearer picture of the reliability and precision of your estimates.
Advanced Statistical Methods
Advanced statistical methods allow for more sophisticated analysis of complex public health data. This section covers regression analysis and survival analysis, which are essential for modeling relationships and analyzing time-to-event data.
Regression Analysis
Regression analysis models the relationship between a dependent variable and one or more independent variables. It is widely used in public health to identify risk factors and predict health outcomes.
Linear Regression
Linear regression examines the relationship between a continuous dependent variable and one or more continuous or categorical independent variables. For example, you might use linear regression to study the impact of dietary intake on cholesterol levels.
- Model fitting:Assess the fit of the regression model using R-squared and residual plots.
- Interpretation:Interpret the coefficients to understand the effect of each independent variable on the dependent variable.
Linear regression helps identify significant predictors and quantify their impact, making it a powerful tool for public health research.
Logistic Regression
Logistic regression is used when the dependent variable is binary (e.g., presence or absence of high cholesterol). It estimates the probability of an event occurring based on the independent variables.
- Odds ratios: Interpret the coefficients as odds ratios, indicating the change in odds of the outcome for a one-unit change in the predictor.
Logistic regression is essential for studying binary outcomes and identifying key risk factors in public health.
Survival Analysis
Survival analysis examines the time until an event occurs (e.g., time to disease occurrence). It is useful for analyzing data with censored observations.
Kaplan-Meier Estimator
The Kaplan-Meier estimator estimates the survival function and provides a visual representation of survival probabilities over time.
- Survival curves: Plot survival curves to compare different groups (e.g., different treatment groups).
Kaplan-Meier curves offer a straightforward way to visualize survival data and compare groups over time.
Cox Proportional Hazards Model
The Cox model assesses the effect of multiple variables on survival time, allowing for adjustment of confounders.
- Hazard ratios:Interpret the coefficients as hazard ratios, indicating the change in hazard for a one-unit change in the predictor.
The Cox model is valuable for identifying and quantifying the impact of various factors on survival time, making it indispensable for longitudinal studies.
Practical Steps for Public Health Assignments
Applying biostatistical techniques to public health assignments involves several practical steps. This section provides guidance on data preparation, analysis, and reporting to ensure accurate and meaningful results.
Data Preparation
Data preparation is a critical step in any statistical analysis. It involves cleaning and organizing the data to ensure accurate and reliable results.
Data Cleaning
Data cleaning involves identifying and correcting errors or inconsistencies in the dataset:
- Missing values:Handle missing data appropriately (e.g., imputation or exclusion).
- Outliers:Detect and address outliers that may skew the results.
- Consistency:Ensure consistent coding and formatting of variables.
Proper data cleaning is essential for avoiding biased or inaccurate results, ensuring the integrity of your analysis.
Variable Creation
Creating new variables can help simplify the analysis and provide more meaningful insights:
- Categorical variables: Convert continuous variables into categorical ones if necessary (e.g., age groups).
- Composite scores:Combine multiple variables into a single score (e.g., a dietary index).
Variable creation allows for more nuanced analysis and can help reveal important patterns in the data.
Data Analysis
Once the data is prepared, you can proceed with the analysis using the appropriate statistical methods.
Descriptive Analysis
Begin with a descriptive analysis to summarize the data and identify patterns:
- Tables and charts: Create summary tables and charts to visualize the data.
- Descriptive statistics: Calculate measures of central tendency and dispersion for key variables.
Descriptive analysis provides an initial understanding of the data and highlights important characteristics and trends.
Inferential Analysis
Conduct inferential analysis to test hypotheses and draw conclusions:
- Hypothesis tests: Perform the relevant hypothesis tests (e.g., t-tests, chi-square tests).
- Confidence intervals:Calculate confidence intervals to assess the precision of the estimates.
Inferential analysis allows you to make data-driven decisions and validate your findings statistically.
Reporting Results
Effectively reporting your results is crucial for communicating your findings to a broader audience.
Writing the Report
Structure your report to clearly present your analysis and conclusions:
- Introduction: Provide background information and state the research question.
- Methods:Describe the data, variables, and statistical methods used.
- Results:Present the findings with appropriate tables and charts.
- Discussion:Interpret the results, discuss their implications, and acknowledge limitations.
A well-structured report ensures clarity and comprehensiveness, making it easier for readers to understand and evaluate your work.
Creating Appendices
Include appendices for additional tables, charts, and detailed results that support the main report.
- Tables and figures: Ensure all tables and figures are well-labeled and referenced in the report.
- Supplementary materials:Provide any additional analyses or data that support your conclusions.
Appendices provide detailed support for your main findings, allowing for a deeper understanding of the analysis.
Conclusion
Biostatistics is a powerful tool for public health research and analysis. By mastering descriptive and inferential statistics, regression analysis, and survival analysis, you can effectively tackle public health assignments and draw meaningful conclusions from health data. Remember to follow practical steps for data preparation, analysis, and reporting to ensure your work is accurate, reliable, and impactful. Whether you are investigating cholesterol levels, dietary intake, or other health outcomes, these biostatistics techniques will equip you with the skills needed to succeed in your assignments.