Claim Your Offer
Unlock a fantastic deal at www.statisticsassignmenthelp.com with our latest offer. Get an incredible 10% off on all statistics assignment, ensuring quality help at a cheap price. Our expert team is ready to assist you, making your academic journey smoother and more affordable. Don't miss out on this opportunity to enhance your skills and save on your studies. Take advantage of our offer now and secure top-notch help for your statistics assignments.
We Accept
- Types of Bias in Randomized Trials
- Selection Bias: The Sneaky Saboteur of Randomization
- Measurement Bias: Distorting Reality One Measurement at a Time
- Statistical Tools for Detecting Bias
- Regression Analysis: Unraveling Hidden Biases
- Propensity Score Matching: Balancing Act for Unbiased Comparisons
- Strategies to Minimize Bias in Randomized Trials
- Double-Blinding: Shielding Against Unconscious Biases
- Intention-to-Treat Analysis: Embracing Real-World Complexity
- Ethical Considerations in Randomized Trials
- Reporting and Publication Biases in Randomized Trials
- Conclusion
Randomized controlled trials (RCTs) represent the zenith of scientific inquiry, epitomizing methodological rigor and precision. Positioned as the gold standard, they promise clarity in discerning causal relationships. However, beneath the veneer of randomness lies a complex terrain where biases, often imperceptible, can weave their intricate web. These biases, if undetected and unaddressed, can surreptitiously warp the very fabric of research outcomes, leading to skewed interpretations and misguided conclusions.
In this in-depth exploration, we embark on a profound journey into the labyrinthine world of biases within randomized trials. Armed with statistical tools and ethical insights, we illuminate the shadowy corners where biases dwell. This knowledge transcends the realm of assignments, serving as a lighthouse guiding students through the stormy seas of scientific inquiry. It becomes a cornerstone upon which their analytical acumen is honed, empowering them not only to decode assignments but also to critically dissect research literature.
Understanding these biases elevates students' comprehension, nurturing astute minds capable of discerning the subtlest distortions in experimental designs. Through this exploration, students cultivate a deep-rooted understanding, transforming them into guardians of scientific integrity. This knowledge, woven intricately into their academic tapestry, shapes them into conscientious contributors to the ever-evolving landscape of scientific discovery. If you need assistance with your statistics assignment, this knowledge equips you with the tools to approach your tasks with clarity and precision.
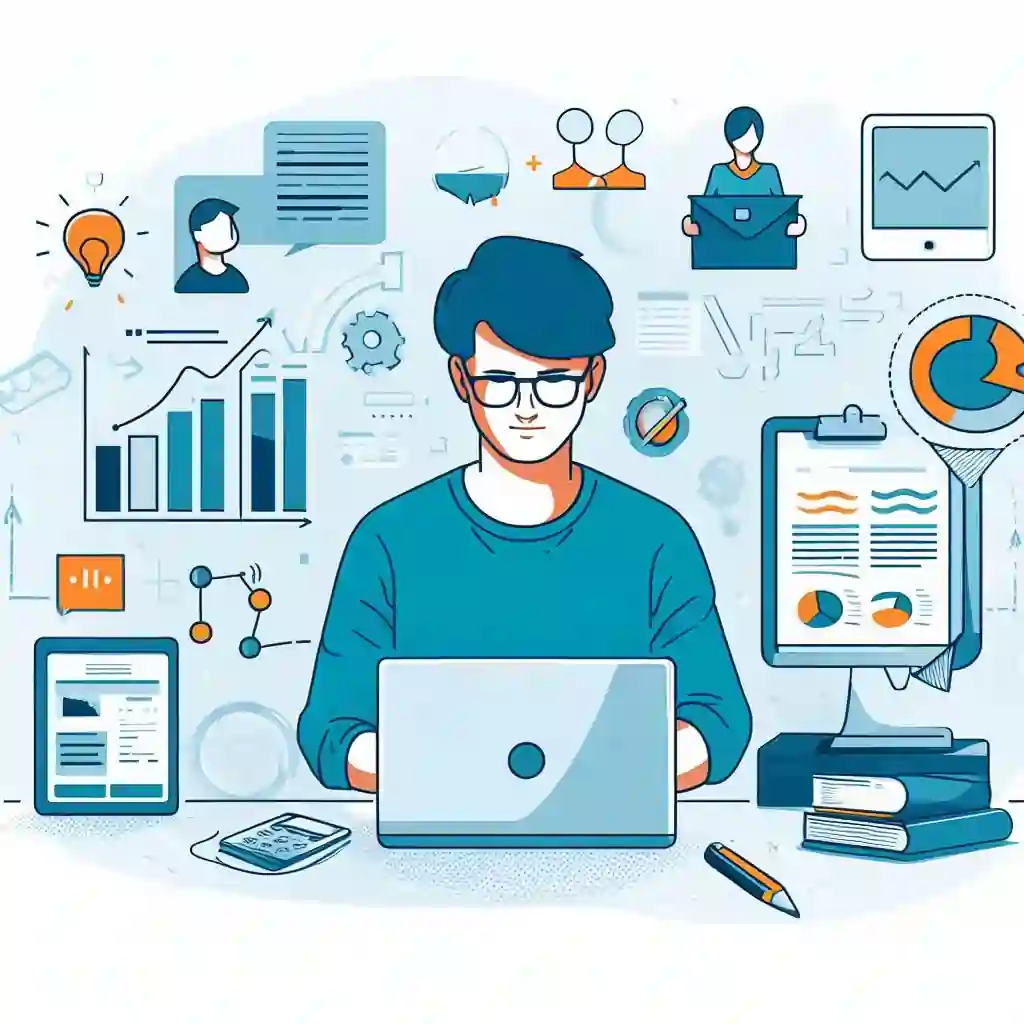
Types of Bias in Randomized Trials
Understanding the various types of biases that can infiltrate randomized trials is fundamental to comprehending the challenges and potential pitfalls in research design. In this section, we will delve into the two primary categories of biases that can undermine the validity of an RCT: selection bias and measurement bias.
Selection bias arises from issues in the randomization process itself, causing participants to be unevenly distributed between control and experimental groups. This can skew the results and lead to inaccurate conclusions. On the other hand, measurement bias pertains to issues in how the outcomes are measured, introducing inaccuracies that can also distort the study's findings. Recognizing the nature of these biases and how they manifest is crucial for students and researchers alike as it forms the foundation for deploying the right tools to identify and address these challenges.
Selection Bias: The Sneaky Saboteur of Randomization
Selection bias, often insidious and difficult to detect, threatens the very essence of randomization. It materializes when the random assignment process is compromised, leading to a non-random distribution of participants between the control and experimental groups. This can occur due to various reasons, such as flawed randomization techniques or inadvertent systematic differences between the groups. In essence, selection bias tips the scale, making it appear as though the intervention has an effect when, in fact, the observed differences are a result of the biased selection process.
Measurement Bias: Distorting Reality One Measurement at a Time
Measurement bias, also known as information bias, emerges when the tools used to measure outcomes are flawed or when there is human error in the measurement process. Biased assessments, faulty instruments, or even participants misreporting their experiences can introduce measurement bias. In the context of RCTs, this form of bias can lead to erroneous conclusions, making it imperative for researchers to ensure meticulous measurement procedures to maintain the trial's integrity.
Statistical Tools for Detecting Bias
To maintain the integrity of randomized trials, it's essential to have robust tools that can pinpoint biases. In this section, we'll explore two such tools: regression analysis and propensity score matching.
Regression analysis serves as a statistical workhorse, capable of uncovering hidden biases by examining the relationship between the treatment variable and various covariates. This method is invaluable for identifying variables that significantly impact outcomes but were not adequately controlled during randomization.
Propensity score matching, on the other hand, is a sophisticated technique for balancing covariates between treatment and control groups, effectively mitigating selection bias. These statistical tools are the magnifying glasses researchers use to scrutinize the data, ensuring the trial's objectivity.
Regression Analysis: Unraveling Hidden Biases
Regression analysis, a cornerstone of statistical methodology, plays a pivotal role in identifying biases within randomized trials. By modeling the relationship between the treatment variable and various covariates, researchers can pinpoint potential biases. Significant variables influencing outcomes, which were not adequately controlled during randomization, serve as red flags, indicating the presence of bias. Regression analysis acts as a discerning lens, allowing researchers to scrutinize the data and unveil patterns that might otherwise remain obscured.
Propensity Score Matching: Balancing Act for Unbiased Comparisons
Propensity score matching stands as a sophisticated statistical technique designed to mitigate biases in randomized trials. By calculating the propensity score, representing the likelihood of receiving the treatment, researchers can match individuals in the control group with counterparts in the treatment group who share similar propensity scores. This meticulous matching process balances the covariates, effectively neutralizing selection bias. Propensity score matching serves as a powerful tool, ensuring that the compared groups are as similar as possible, allowing for a more accurate estimation of treatment effects.
Strategies to Minimize Bias in Randomized Trials
While detecting biases is crucial, it is equally vital to implement strategies that minimize their impact. This section introduces two powerful strategies: double-blinding and intention-to-treat analysis.
Double-blinding ensures that neither participants nor researchers are aware of who is receiving the treatment, shielding the study from subconscious biases. It represents a crucial shield against unintended influences.
Intention-to-treat analysis, on the other hand, acknowledges the realities of real-world scenarios, where participants may not always adhere perfectly to their assigned interventions. It offers a pragmatic approach to data analysis, preserving the randomized nature of the trial and providing results that align with the complexities of everyday life. These strategies are indispensable for maintaining the accuracy and credibility of randomized trials.
Double-Blinding: Shielding Against Unconscious Biases
Double-blinding, a cornerstone of rigorous experimental design, stands as a robust defense against biases in randomized trials. In a double-blind study, both participants and researchers are unaware of who receives the treatment and who receives the placebo. This intentional ignorance eliminates the potential for subconscious cues to influence either the participants' responses or the researchers' assessments. By shrouding the entire experimental process in secrecy, double-blinding ensures that the study's outcomes are a genuine reflection of the intervention's efficacy, devoid of any unintended biases.
Intention-to-Treat Analysis: Embracing Real-World Complexity
Intention-to-treat (ITT) analysis, a methodological approach that includes all participants in the groups to which they were originally assigned, regardless of their adherence to the treatment, is a formidable weapon against biases stemming from dropouts or non-compliance. In the unpredictable landscape of real-world scenarios, participants may not always adhere perfectly to the assigned interventions. ITT analysis acknowledges this complexity, offering a pragmatic perspective. By analyzing participants based on their initial randomization, ITT preserves the trial's randomized nature, providing a robust estimation of the intervention's effects in a manner that aligns with real-world challenges.
Ethical Considerations in Randomized Trials
In this section, we will explore the ethical dimensions of conducting randomized trials. Ethical considerations are paramount in research, and they can significantly impact the design, execution, and interpretation of RCTs. Students must be aware of the ethical challenges that may arise, such as informed consent, potential harm to participants, and the equitable distribution of benefits. Understanding these ethical nuances not only aids in completing assignments but also prepares students to conduct research responsibly and with the welfare of participants in mind.
Reporting and Publication Biases in Randomized Trials
The dissemination of research findings is a critical aspect of the scientific process. However, reporting and publication biases can distort the overall scientific knowledge. In this section, we'll delve into how these biases occur and why they are problematic. Students will gain insights into the importance of complete and transparent reporting of results, the impact of selective publication, and potential strategies to mitigate these biases. Understanding the potential distortions introduced by reporting and publication biases is crucial for students to critically evaluate the existing literature and contribute to a more accurate and balanced body of scientific knowledge.
Conclusion
In the intricate tapestry of scientific research, where the pursuit of truth is both an art and a science, understanding and mitigating biases in randomized trials emerge as fundamental and indispensable skills. These skills serve as the bedrock upon which the edifice of reliable scientific inquiry stands. For students embarking on the complex terrain of statistics and research methodology, mastering the subtleties of bias detection and mitigation becomes an intellectual rite of passage. It equips them not merely with knowledge but with a discerning eye, a tool invaluable in deciphering the often convoluted maze of empirical studies.
These acquired skills transcend the confines of classroom assignments, extending into the realm of critical thinking. Students, armed with this profound understanding, develop a critical mindset that is essential for evaluating the quality of research studies encountered in their academic journey. This discernment empowers them to question methodologies, identify potential biases, and engage deeply with the nuances of scientific investigations.
Moreover, as the torchbearers of future research, their ability to identify, address, and prevent biases becomes paramount. By honing these skills, they become guardians of the integrity of scientific inquiry. Their vigilant scrutiny ensures that discoveries, innovations, and breakthroughs are forged in the crucible of rigorous scrutiny, standing resilient against the tests of skepticism and inquiry. In this evolving landscape, their expertise becomes not just a personal achievement but a cornerstone of advancing knowledge, fostering an environment where scientific truth triumphs.