Claim Your Offer
Unlock a fantastic deal at www.statisticsassignmenthelp.com with our latest offer. Get an incredible 10% off on all statistics assignment, ensuring quality help at a cheap price. Our expert team is ready to assist you, making your academic journey smoother and more affordable. Don't miss out on this opportunity to enhance your skills and save on your studies. Take advantage of our offer now and secure top-notch help for your statistics assignments.
We Accept
- What is Linear Regression?
- Understanding Criterion Variables
- Performing Simple Linear Regression
- Performing Multiple Linear Regression
- Calculating Residuals
- Making Predictions with Linear Regression
- Practical Application with Stata
- Conclusion
Linear regression is a cornerstone of statistical analysis and an essential tool for uncovering meaningful patterns in data. Whether you're analyzing trends or predicting future outcomes, understanding linear regression can significantly enhance your ability to interpret and leverage data effectively. In this comprehensive exploration, we'll delve into both simple and multiple linear regression techniques, providing you with the insights needed to excel in data analysis. This blog aims to equip you with the knowledge to handle various aspects of regression analysis, offering clear explanations and practical examples. If you’re looking for help with linear regression assignment, you’ll find this guide particularly useful. By grasping the fundamental concepts and learning how to apply them in real-world scenarios, you'll be better prepared to tackle complex regression tasks with confidence. From calculating residuals to making accurate predictions, mastering these techniques will enable you to extract valuable insights and make data-driven decisions. Whether you're a student needing assistance with your coursework or a professional seeking to refine your analytical skills, this blog will provide the tools and understanding necessary to navigate the world of linear regression successfully.
What is Linear Regression?
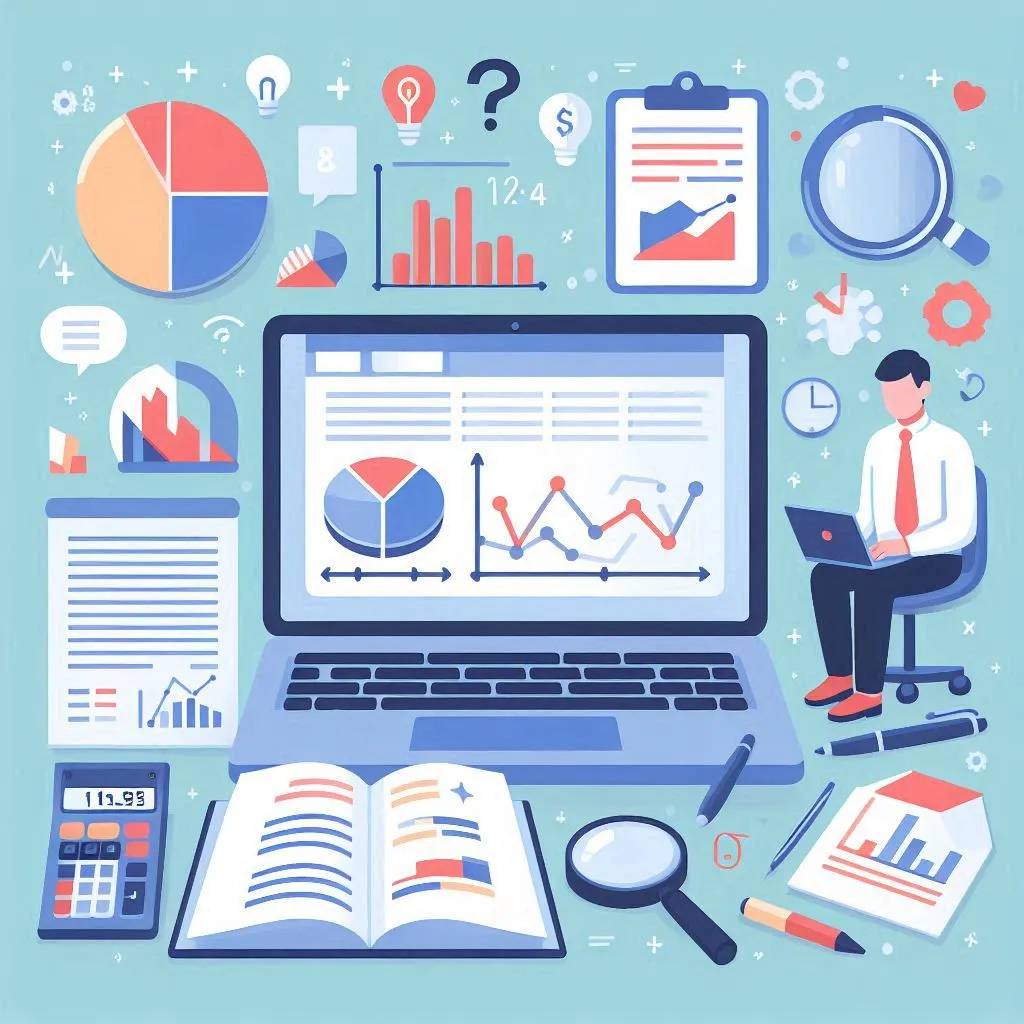
Linear regression is a statistical method used to understand the relationship between two or more variables. The primary goal is to model this relationship so you can predict one variable based on the other(s).
- Simple Linear Regression: This involves two variables: one dependent and one independent. The dependent variable is what you want to predict, while the independent variable is what you use to make the prediction. For example, if you’re looking at how the number of hours studied affects exam scores, exam scores are the dependent variable, and study hours are the independent variable.
- Multiple Linear Regression: This expands on simple linear regression by including multiple independent variables. This approach is useful when you want to understand how several factors collectively impact the dependent variable. For instance, if you want to study how hours studied, class attendance, and previous grades affect exam scores, you would use multiple linear regression.
Understanding Criterion Variables
In any regression analysis, the criterion variable, also known as the dependent variable, is the focus of your study. It’s the outcome you’re trying to predict or explain. For example, in a study examining how advertising spending influences sales, sales would be the criterion variable. Identifying and understanding your criterion variable is crucial for structuring your analysis and selecting appropriate predictor variables.
Performing Simple Linear Regression
Simple linear regression is straightforward: you assess the relationship between one dependent variable and one independent variable. The outcome is a line that best fits the data points on a graph. This line is used to make predictions about the dependent variable based on new values of the independent variable.
Example: Suppose you’re analyzing how study hours affect exam scores. By applying simple linear regression, you can determine a line that best represents this relationship. If a student studies for 5 hours, you can use this line to predict their likely exam score.
Performing Multiple Linear Regression
Multiple linear regression is used when you have more than one independent variable. It helps in understanding how several factors impact the dependent variable simultaneously. This technique is more complex but provides a more comprehensive view of the relationships in your data.
Example: If you’re looking at how study hours, class attendance, and previous grades affect exam scores, multiple linear regression allows you to see how each factor contributes to the outcome. This method provides a more nuanced view of how different variables work together to influence the dependent variable.
Calculating Residuals
Residuals are the differences between the observed values and the values predicted by your regression model. They are crucial for assessing the fit of your model. A smaller residual indicates that the model's predictions are closer to the actual values.
Example: If your regression model predicts that a student who studied for 5 hours should score 80 on an exam, but the actual score was 75, the residual would be 5. Analyzing these residuals helps you understand how well your model is performing and whether any adjustments are needed.
Making Predictions with Linear Regression
Once you have a regression model, you can use it to make predictions. This involves using the model's equation to forecast the dependent variable based on new values of the independent variables.
Example: If your model indicates that each additional hour of study improves exam scores by 2 points, you can predict that a student who studies for 7 hours will score 14 points higher than a student who studies for 5 hours.
Practical Application with Stata
Stata is a powerful software tool for performing regression analysis. To conduct simple linear regression in Stata, you would use commands to specify your dependent and independent variables. For multiple regression, you can include multiple predictors in your command. Stata also provides tools to visualize results, check model assumptions, and make predictions, making it a comprehensive option for regression analysis.
Conclusion
In conclusion, linear regression is a vital tool in data analysis, essential for uncovering relationships between variables and making accurate predictions. By delving into both simple and multiple linear regression, you gain valuable skills for analyzing how different factors influence outcomes. Simple linear regression focuses on the relationship between one independent and one dependent variable, providing a clear, straightforward model. Multiple linear regression expands on this by incorporating multiple predictors, offering a more comprehensive view of complex interactions.
Understanding the criterion variable, or the outcome you're predicting, is key to structuring your analysis effectively. Calculating residuals helps assess how well your model fits the data, while making predictions allows you to forecast future values based on your regression equations.
For those needing help with statistics assignment, applying these techniques will enhance your ability to interpret and utilize data. Tools like Stata simplify these processes, offering practical support for performing and analyzing regression models. Mastering these concepts equips you with the knowledge to tackle complex data analysis tasks and make informed, data-driven decisions.