Avail Your Offer
Unlock success this fall with our exclusive offer! Get 20% off on all statistics assignments for the fall semester at www.statisticsassignmenthelp.com. Don't miss out on expert guidance at a discounted rate. Enhance your grades and confidence. Hurry, this limited-time offer won't last long!
We Accept
- 1. Formulating Hypotheses
- Example:
- 2. Developing Your Theory
- Tips:
- 3. Creating Cross-Tabulation Tables
- Components:
- Example Table:
- How to Interpret:
- 4. Generating Bar Charts
- Tips:
- Example Bar Chart:
- 5. Analyzing and Interpreting Results
- Descriptive Analysis:
- Making Decisions:
- Example Interpretation:
- Conclusion
When tackling statistical assignments that involve hypothesis testing, cross-tabulations, and graphical representation, it’s crucial to adopt a methodical and organized approach. This structured strategy ensures that each component of the analysis is thoroughly addressed and clearly communicated. By systematically following these steps, you can enhance your ability to manage complex data, derive meaningful insights, and present your findings with precision and clarity.
Begin by formulating clear and concise hypotheses that accurately reflect the expected relationships between variables. This foundational step sets the stage for the entire analysis, guiding your subsequent data exploration and interpretation. Next, develop a well-supported theory that explains why you anticipate a particular relationship, grounding your expectations in relevant research or theoretical frameworks.
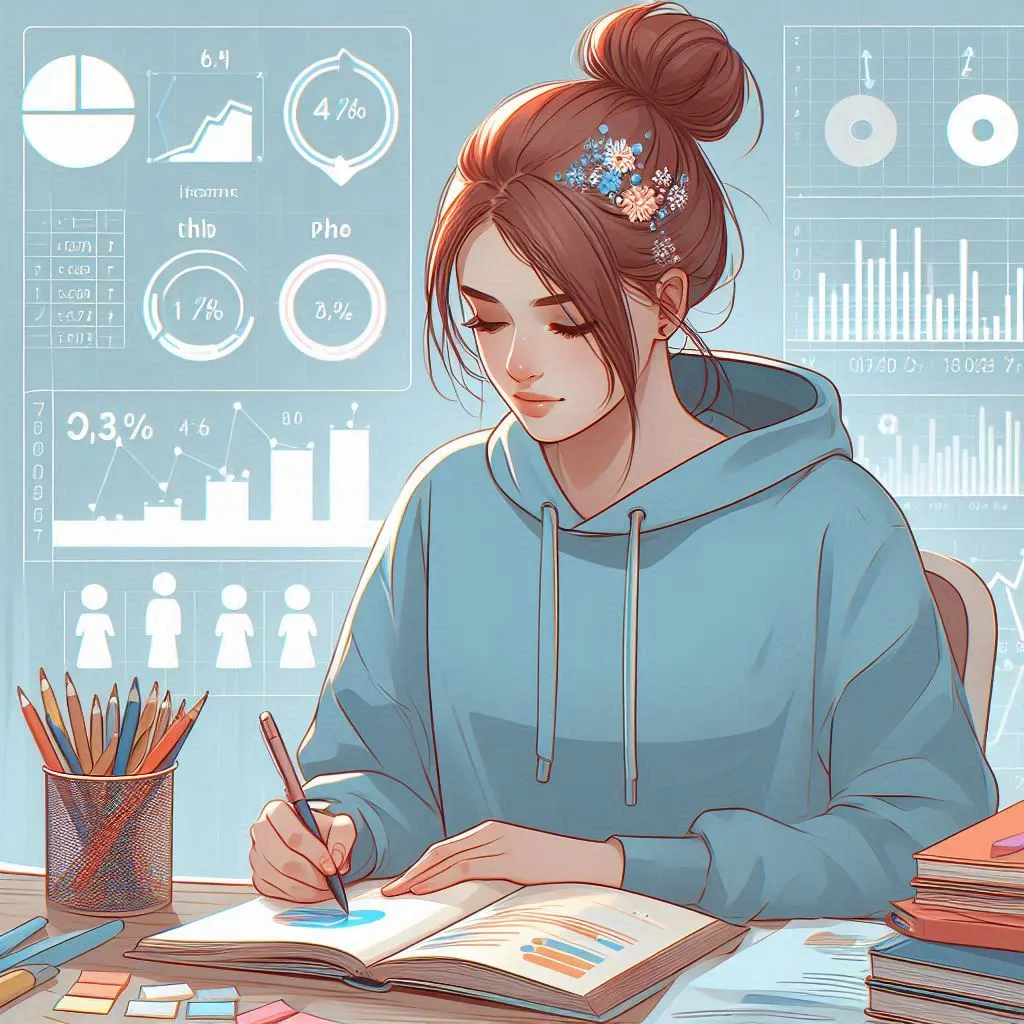
When preparing cross-tabulations, ensure that your tables are detailed and well-organized, providing a comprehensive view of the data. Include relevant statistics to support your hypotheses and make your tables easy to interpret. Complement your tabular data with graphical representations, such as bar charts, that visually convey the relationships and distributions within your data. These visual aids are crucial for making complex data more accessible and understandable.
As you analyze your results, focus on verifying whether your theoretical expectations align with the observed data. Perform statistical tests meticulously and report their results clearly, including test statistics, degrees of freedom, and p-values. This step is essential for validating your hypotheses and making informed decisions based on statistical evidence.
In your interpretation, discuss the implications of your findings in relation to your theory and hypotheses. Address any unexpected results or anomalies and consider their impact on your overall conclusions. This thorough analysis not only enhances the accuracy of your results but also improves your ability to communicate them effectively.
By adopting this comprehensive and structured approach, you can tackle statistical assignments with confidence and competence. This methodology not only helps you navigate the complexities of data analysis using SPSS but also ensures that your findings are presented in a clear, coherent, and convincing manner. Whether you're seeking hypothesis testing assignment help or aiming to enhance your analytical skills, this approach will ultimately lead to academic success and a deeper understanding of statistical principles.
1. Formulating Hypotheses
When approaching a statistical assignment, the initial step involves formulating hypotheses that will guide your analysis. Hypotheses provide a foundation for investigating relationships between variables and setting the stage for empirical testing. A well-defined hypothesis helps to clarify the focus of your research and directs your analytical efforts. For those seeking statistics assignment help, creating clear and specific hypotheses is essential in ensuring that your analysis is both targeted and effective, leading to more accurate and insightful results.
- Research Hypothesis: This hypothesis is a specific, predictive statement about the relationship between two or more variables. It should clearly articulate the expected direction or nature of the relationship based on theoretical reasoning or prior research. The research hypothesis guides the analysis and sets the foundation for testing whether the anticipated relationship exists.
- Null Hypothesis: The null hypothesis represents the default position that there is no effect or relationship between the variables. It serves as a baseline against which the research hypothesis is tested. By assuming no relationship, the null hypothesis allows for a statistical evaluation of whether any observed effects are likely due to chance.
Example:
- Research Hypothesis: Individuals with higher levels of education are more likely to support environmental policies compared to those with lower levels of education. This hypothesis suggests that as education increases, so does support for environmental policies, indicating a positive relationship between education level and environmental advocacy.
- Null Hypothesis: There is no significant relationship between education level and support for environmental policies. This hypothesis posits that variations in education level do not influence or predict support for environmental policies, serving as a basis for comparing against the research hypothesis to determine if any observed differences are statistically significant.
2. Developing Your Theory
Before diving into the data, it's crucial to develop a clear and well-grounded theory that explains the expected relationship between your variables. This theoretical framework provides context for your analysis and helps to justify why you anticipate observing a particular outcome. A robust theory not only informs your hypothesis but also guides the interpretation of your results.
Tips:
- Ensure a Strong Foundation: Your theory should be based on a thorough understanding of both the independent and dependent variables involved. It should be informed by relevant literature, empirical studies, or established theoretical frameworks. This grounding demonstrates that your hypothesis is not just speculative but is based on sound reasoning and evidence.
- Consider Previous Research: Review existing studies and theoretical models related to your topic. Understanding prior findings and theoretical perspectives can help you construct a theory that aligns with or builds upon established knowledge. This background knowledge can provide insights into the mechanisms underlying the relationship between your variables and strengthen your hypothesis.
- Use Logical Reasoning: When developing your theory, apply logical reasoning to explain why you expect the relationship you are hypothesizing. Consider how the independent variable might influence the dependent variable based on the nature of the variables and their interactions. A well-reasoned theory enhances the credibility of your hypothesis and provides a clear rationale for your expected results.
By carefully developing your theory, you set a solid foundation for analyzing your data and interpreting your findings. This theoretical grounding helps to ensure that your analysis is both meaningful and relevant, making your conclusions more robust and well-supported.
3. Creating Cross-Tabulation Tables
Cross-tabulation is an essential method for analyzing the relationship between two categorical variables. It allows you to organize and summarize data in a way that makes it easier to identify patterns, relationships, and potential associations. To create an effective cross-tabulation table, include the following key components:
Components:
- Frequency Counts: Present the number of occurrences or observations within each category. This provides a clear view of how often each combination of categories occurs, which is crucial for understanding the distribution of your data.
- Percentages: Calculate and display the proportion of each category relative to the total. Percentages help to contextualize the frequency counts, making it easier to see the relative importance or distribution of each category within the dataset.
Example Table:
VariableA | Category1 | Category2 | Total |
---|---|---|---|
Category 1 | 50 | 70 | 120 |
Category 2 | 30 | 80 | 110 |
Total | 80 | 150 | 230 |
How to Interpret:
- Frequency Counts: In the table, you can see how many observations fall into each category combination. For example, 50 observations fall into Category 1 of Variable A and Category 1 of Variable B.
- Percentages: Calculate the percentage for each cell to understand the proportion of each category relative to the total. This helps in comparing the distribution of categories more effectively.
- Overall Patterns: Analyze the table to identify any patterns or trends. For instance, you might look at whether there is a higher frequency of certain combinations or whether one category tends to occur more frequently with another.
Creating a well-structured cross-tabulation table is fundamental for effectively testing your hypothesis and interpreting the relationships between categorical variables. It provides a clear, organized view of the data, enabling you to draw meaningful conclusions and support your analysis with solid evidence.
4. Generating Bar Charts
A bar chart is a powerful visual tool that helps you represent and compare the frequency or proportion of different categories in your data. It provides a clear, visual representation that can make it easier to identify trends and relationships. To create an effective bar chart, consider the following tips:
Tips:
- Use Contrasting Colors: Choose distinct colors for different categories to enhance readability and make it easier to differentiate between them. For example, if you are comparing survey responses across various groups, use a unique color for each group to ensure clarity.
- Include a Legend: A legend is essential for explaining what each color or pattern represents. This helps viewers understand the categories being compared without confusion.
- Label Axis Clearly: Ensure that both the x-axis and y-axis are labeled with descriptive titles. The x-axis typically represents the categories, while the y-axis shows the frequency or proportion. Clear labels make it easier for viewers to interpret the data accurately.
- Provide a Title: Include a concise title at the top of the bar chart that summarizes what the chart is showing. This helps to immediately inform viewers about the focus of the data being presented.
- Ensure Proper Scale: Make sure the scale on the y-axis is appropriate for the data range. Avoid overly large or small scales that might distort the interpretation of the bar heights.
Example Bar Chart:
Imagine you are comparing the support for different policies across several regions. Your bar chart might look like this:
- Title: Support for Environmental Policies by Region
- X-Axis: Regions (e.g., North, South, East, West)
- Y-Axis: Percentage of Support
- Bars: Different colors representing each policy (e.g., Blue for Policy A, Green for Policy B)
By following these tips, you can create a bar chart that effectively communicates the distribution and comparison of categories in your data. A well-designed bar chart not only enhances the visual appeal of your analysis but also improves the clarity and impact of your findings.
5. Analyzing and Interpreting Results
Once you have completed your statistical analysis, the next crucial step is to analyze and interpret your results comprehensively. This involves both descriptive analysis and making informed decisions based on statistical tests.
Descriptive Analysis:
- Theory Verification: Start by comparing your observations with the theoretical expectations you developed earlier. Determine whether the data aligns with your hypothesized relationships. This step helps validate whether your initial theory holds true or if adjustments are necessary.
- Statistical Test Results: Report the outcomes of your statistical tests clearly and accurately. Include key details such as the test statistic, degrees of freedom, and p-value. For example, if you performed a Chi-square test, present the results in the following format:
χ2(df)=value, p=value\chi^2 (\text{df}) = \text{value}, \, p = \text{value}χ2(df)=value,p=value
Here, χ2\chi^2χ2 represents the Chi-square statistic, df\text{df}df denotes the degrees of freedom, and ppp is the p-value. This information is critical for understanding the significance of your results.
Making Decisions:
- Rejecting or Failing to Reject the Null Hypothesis: Based on the p-value obtained from your statistical test, decide whether there is sufficient evidence to reject the null hypothesis. A p-value less than 0.05 typically indicates statistical significance, suggesting that the observed relationship is unlikely due to random chance. If the p-value is greater than 0.05, you fail to reject the null hypothesis, meaning there is not enough evidence to support a significant relationship between the variables.
- Interpreting Results: Discuss the implications of your findings in relation to your hypotheses and theory. Evaluate the strength and direction of the observed relationship. For instance, if your analysis reveals a strong positive correlation, explain what this implies about the variables' interaction. Additionally, consider any nuances or additional insights that might emerge from your analysis, such as unexpected trends or anomalies.
Example Interpretation:
If your p-value is less than 0.05, you would reject the null hypothesis, indicating that the relationship between the variables is statistically significant. For instance, if you find that individuals with higher education levels show significantly greater support for environmental policies compared to those with lower education levels, this supports your research hypothesis and aligns with your theory. Discuss any additional details that might inform your conclusions, such as the magnitude of the effect or potential limitations in your study.
By carefully analyzing and interpreting your results, you can draw meaningful conclusions and provide a thorough understanding of the relationships between your variables. This process ensures that your findings are well-supported and clearly communicated.
Conclusion
By systematically formulating hypotheses, developing a well-grounded theory, preparing cross-tabulations, creating informative bar charts, and thoroughly analyzing your results, you can effectively manage and excel in complex statistical assignments. This structured approach ensures that you address each component of the assignment with clarity and precision, leading to more accurate and insightful findings.
- Formulating Hypotheses: Start by crafting precise research and null hypotheses. A well-defined research hypothesis predicts the expected relationship between variables, while the null hypothesis serves as a benchmark to test for the absence of such a relationship. This initial step sets a clear direction for your analysis and establishes the framework for testing your assumptions.
- Developing a Well-Grounded Theory: Construct a robust theoretical framework based on relevant literature and logical reasoning. This theory should explain why you expect to see a particular relationship between your variables. A strong theory not only informs your hypotheses but also helps justify the analytical approach and interpretation of results.
- Preparing Cross-Tabulations: Create detailed cross-tabulation tables to summarize and visualize the relationship between categorical variables. These tables provide frequency counts and percentages, helping to reveal patterns and trends within your data. Properly structured cross-tabulations facilitate a clearer understanding of how variables interact.
- Creating Informative Bar Charts: Utilize bar charts to represent the frequency or proportion of different categories visually. Choose contrasting colors for clarity, and include a legend and axis labels to ensure that the chart is easy to interpret. Bar charts make it simpler to compare categories and identify significant differences.
- Analyzing and Interpreting Results: Conduct a thorough analysis of your data, verifying whether the observations align with your theoretical expectations. Report the results of your statistical tests, including test statistics, degrees of freedom, and p-values. Based on these results, decide whether to reject or fail to reject the null hypothesis and discuss the implications, including the strength and direction of the observed relationships.
By following these steps, you not only meet the requirements of your statistical assignments but also deepen your understanding of statistical relationships. This approach strengthens your overall analytical skills and enhances your ability to draw meaningful conclusions from complex data.