Avail Your Offer Now
Celebrate the festive season with an exclusive holiday treat! Enjoy 15% off on all orders at www.statisticsassignmenthelp.com this Christmas and New Year. Unlock expert guidance to boost your academic success at a discounted price. Use the code SAHHOLIDAY15 to claim your offer and start the New Year on the right note. Don’t wait—this special offer is available for a limited time only!
We Accept
- Introduction to Correlation and Association
- Pearson's r Correlation for Linear Relationships
- How to Calculate Pearson's r in SPSS
- Spearman's Rank-Order Correlation for Non-Linear Relationships
- How to Calculate Spearman's ρ in SPSS
- Practical Applications of Correlation and Association
- Tips for Successful Correlation and Association Analysis in SPSS
- Common Mistakes in Correlation Analysis and How to Avoid Them
- Conclusion
If you're a university student grappling with your SPSS assignment and need assistance with your Correlation and Association assignment using SPSS, you're not alone. The world of statistical analysis software can be daunting, especially for beginners. However, mastering key concepts like correlation and association is essential for success in fields like psychology, sociology, and business. In this comprehensive guide, we'll delve into the two fundamental statistical techniques used in SPSS to examine relationships between variables: Pearson's r correlation for linear relationships and Spearman's rank-order correlation for non-linear relationships.
Introduction to Correlation and Association
Correlation and association are statistical methods that help us understand the relationships between variables in a dataset. They are commonly used in research and data analysis to uncover patterns and make informed decisions. SPSS, a popular software tool among researchers, provides various methods to perform these analyses efficiently.
Pearson's r Correlation for Linear Relationships
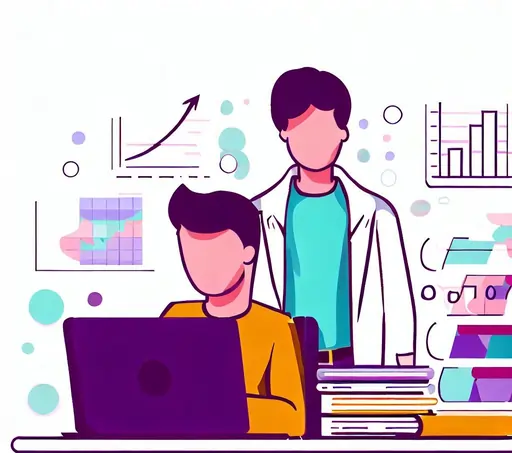
Pearson's correlation coefficient (often denoted as "r") is a statistic that quantifies the strength and direction of a linear relationship between two continuous variables. It ranges from -1 to 1:
- An r value of 1 indicates a perfect positive linear relationship, where one variable increases as the other does.
- An r value of -1 indicates a perfect negative linear relationship, where one variable decreases as the other does.
- An r value of 0 indicates no linear relationship between the variables.
How to Calculate Pearson's r in SPSS
- Open your dataset: Load your dataset in SPSS.
- Choose Analyze:Click on the "Analyze" menu and select "Correlate" and then "Bivariate."
- Select Variables:In the "Bivariate Correlations" dialog box, select the two variables you want to analyze.
- Options: You can specify options like confidence intervals and significance levels.
- Click OK: Click the "OK" button to run the analysis.
- Interpret the results:SPSS will provide the correlation coefficient (r) and its significance level (p-value). You can also view a scatterplot to visualize the relationship.
Spearman's Rank-Order Correlation for Non-Linear Relationships
While Pearson's correlation is effective for linear relationships, it may not capture non-linear associations. Spearman's rank-order correlation, denoted as "ρ" (rho), is a non-parametric measure of association that evaluates the strength and direction of a monotonic relationship between two variables. Monotonic relationships are those where variables consistently increase or decrease together but not necessarily at a constant rate.
How to Calculate Spearman's ρ in SPSS
- Open your dataset:As with Pearson's correlation, start by loading your dataset in SPSS.
- Choose Analyze:Click on the "Analyze" menu and select "Correlate" and then "Bivariate."
- Select Variables:Pick the two variables you want to analyze.
- Options:Under "Correlation Coefficients," select "Spearman" instead of "Pearson." You can also specify additional options if needed.
- Click OK: Hit the "OK" button to run the analysis.
- Interpret the results:SPSS will provide Spearman's ρ and its significance level (p-value). Additionally, you can view a scatterplot or a table of ranks to understand the relationship better.
Practical Applications of Correlation and Association
Understanding these two correlation methods and how to apply them in SPSS can be incredibly beneficial for university students working on assignments and research projects. Let's explore some practical applications:
- Social Sciences
- Business and Economics
- Healthcare and Medicine
In fields like psychology and sociology, researchers often use Pearson's correlation to examine the relationship between variables such as age and memory performance or stress levels and academic achievement. Spearman's rank-order correlation can help in cases where the relationship isn't strictly linear, such as evaluating the connection between hours spent studying and exam scores.
Business and economics students frequently use correlation analysis to study factors like consumer spending and income levels. When dealing with data that doesn't adhere to a linear pattern, Spearman's ρ can provide valuable insights, helping to identify trends that might not be apparent with Pearson's r.
Medical and healthcare students can apply correlation techniques to assess the association between variables like body mass index (BMI) and blood pressure. In cases where the relationship might be irregular, Spearman's rank-order correlation can be more informative.
Tips for Successful Correlation and Association Analysis in SPSS
Now that you have a grasp of these statistical techniques and how to apply them using SPSS, here are some tips to ensure your analysis is accurate and reliable:
- Check for Data Assumptions:Ensuring your data meets the necessary assumptions is a critical first step in correlation analysis. For Pearson's correlation, verify that both variables are continuous and normally distributed. If these assumptions aren't met, consider alternative techniques or transformations. Spearman's ρ is more robust, making it suitable for non-normally distributed data. Assess outliers, as they can significantly impact results. Address outliers by either removing them or using appropriate transformations. Remember that failing to account for data assumptions can lead to unreliable findings, making this step essential for accurate and meaningful analysis in SPSS assignments and research projects.
- Consider Outliers: Outliers are data points that deviate significantly from the rest of the dataset and can exert a disproportionate impact on correlation results. Ignoring outliers can lead to skewed and inaccurate findings. To handle outliers effectively, visualize your data with scatterplots to identify them, and then decide whether to remove them or apply data transformations. Remember that the choice depends on the context of your research and the reason for the outliers. Handling outliers thoughtfully ensures that your correlation analysis is robust, reliable, and reflective of the true relationships between variables, which is crucial when solving SPSS assignments accurately.
- Interpret the Coefficient:Interpreting the coefficient is a critical aspect of correlation analysis. This numeric value, ranging from -1 to 1, reveals the strength and direction of the relationship between variables. A coefficient close to 1 signifies a strong positive linear relationship, indicating that as one variable increases, the other does as well. Conversely, a coefficient near -1 suggests a strong negative linear relationship, meaning one variable increases as the other decreases. However, a coefficient near 0 implies no linear relationship. Keep in mind that correlation does not imply causation, so it's essential to consider the context and underlying factors when interpreting the coefficient to draw meaningful conclusions from your data.
- Report Significance Levels:Reporting significance levels is crucial in statistical analysis. It provides essential information about the reliability of your results. When presenting your findings, stating the p-value associated with your correlation coefficient is essential. A low p-value (typically < 0.05) indicates that the observed correlation is unlikely to be due to chance, suggesting a meaningful relationship between the variables. Conversely, a high p-value suggests that the observed correlation could be a random occurrence. By including significance levels, you demonstrate the robustness of your analysis and help readers or reviewers assess the validity of your conclusions. In academia and research, transparency about statistical significance is a fundamental practice.
- Visualize Your Data:Visualizing your data is a crucial step in the correlation analysis process. By creating scatterplots or other relevant graphs, you can gain a clearer understanding of how your variables interact. Visualizations provide insights into the shape, direction, and strength of the relationship, making it easier to identify trends or anomalies. This step is particularly valuable when deciding whether to use Pearson's r or Spearman's ρ. In cases where the relationship isn't strictly linear, visual cues from your graphs may indicate that a non-parametric approach like Spearman's rank-order correlation is more appropriate. Visualization enhances the overall quality and depth of your analysis, aiding in more accurate interpretations and better-informed conclusions.
- Document Your Analysis:Documenting your analysis is not just a good practice; it's an essential step in ensuring the integrity and reproducibility of your work. By meticulously recording your procedures, you create a clear trail of your analytical process, making it easier to spot errors, retrace your steps, and validate your findings. Additionally, well-documented analyses are crucial when presenting your results to peers, instructors, or stakeholders, as it allows others to follow your work and understand your methodology. It's a habit that not only enhances the quality of your assignments but also fosters a professional approach to data analysis, a skill highly valued in academia and beyond.
Common Mistakes in Correlation Analysis and How to Avoid Them
When aiming to solve your SPSS assignment involving correlation analysis, avoiding common mistakes is crucial. Misinterpreting causation, neglecting outliers, and not checking data assumptions can lead to inaccurate results. Stay vigilant, choose the appropriate correlation method, and consider lurking variables. By doing so, you'll navigate your SPSS assignments with precision and confidence, ensuring accurate and meaningful outcomes.
- Confusing Correlation with Causation:One of the most significant errors is assuming that a correlation implies causation. Remember that correlation only indicates a relationship between variables; it doesn't prove that one causes the other. To avoid this mistake, critically evaluate the theoretical basis for causation and consider alternative explanations.
- Small Sample Sizes:Correlation analysis requires a sufficient sample size to produce reliable results. Small samples can lead to unstable or spurious correlations. Ensure your dataset is adequately sized for the analysis you intend to perform.
- Ignoring Outliers:Outliers can distort correlation coefficients, especially Pearson's r. Identify and address outliers appropriately by either removing them or using robust correlation methods.
- Non-Linear Relationships: If your data exhibits a non-linear relationship, relying solely on Pearson's correlation may lead to inaccurate conclusions. Always consider the nature of the data and employ Spearman's rank-order correlation if necessary.
- Failure to Check Assumptions: Pearson's correlation assumes that the variables are continuous and normally distributed. Spearman's ρ is more robust but still requires ordinal or continuous data. Always assess whether your data meets these assumptions before applying correlation analysis.
- Misinterpreting Weak Correlations:Weak correlations are often dismissed as insignificant, but they can still be meaningful in certain contexts. Always consider the practical significance of the correlation in relation to your research question.
- Neglecting Lurking Variables: Lurking or confounding variables can distort correlation results. Be diligent in identifying potential lurking variables that might influence the relationship you're studying.
- Overlooking Time Lags: In longitudinal data, the timing of measurements can be crucial. Failing to account for time lags can lead to erroneous conclusions about causation.
- Publication Bias:When reviewing existing research, be aware of publication bias. Studies showing significant correlations are more likely to be published, potentially skewing your perception of the true relationship.
- Not Updating Your Knowledge:The field of statistics is continually evolving. Stay updated on the latest techniques and best practices in correlation analysis to ensure your work remains accurate and relevant.
By being mindful of these common mistakes and taking steps to avoid them, you can enhance the quality and reliability of your correlation analysis, ultimately helping you excel in your SPSS assignments and research endeavors.
Conclusion
Correlation and association analyses are indispensable tools for university students, especially when tackling assignments in fields that rely heavily on data analysis. With Pearson's r correlation for linear relationships and Spearman's rank-order correlation for non-linear relationships, you have a robust toolkit to explore the connections between variables in your datasets using SPSS. Remember, practice makes perfect. The more you apply these techniques and interpret their results, the more proficient you'll become in using SPSS to solve your assignments and conduct meaningful research. So, embrace the power of correlation and association, and elevate your data analysis skills to new heights.