Claim Your Offer
Unlock a fantastic deal at www.statisticsassignmenthelp.com with our latest offer. Get an incredible 10% off on all statistics assignment, ensuring quality help at a cheap price. Our expert team is ready to assist you, making your academic journey smoother and more affordable. Don't miss out on this opportunity to enhance your skills and save on your studies. Take advantage of our offer now and secure top-notch help for your statistics assignments.
We Accept
- The Essence of Inferential Statistics
- T-Tests for Comparing Means
- Analysis of Variance (ANOVA)
- Chi-Squared Tests for Categorical Data
- Key Considerations for Successful Application
- Conclusion
In the world of statistics, understanding inferential statistics is crucial for university students aiming to excel in data analysis, particularly when working on assignments using SPSS (Statistical Package for the Social Sciences). In this comprehensive guide, we will delve into essential concepts and techniques within inferential statistics, including t-tests, Analysis of Variance (ANOVA), and Chi-squared tests for categorical data. By the end of this blog, you will have a solid foundation to confidently complete your Inferential Statistics assignment using SPSS.
The Essence of Inferential Statistics
Before diving into the specific tests and techniques, let's grasp the fundamental concept of inferential statistics. Inferential statistics enables us to make predictions, inferences, and draw conclusions about a population based on data collected from a sample. In SPSS assignments, you will often encounter situations where you need to analyze and draw inferences about larger populations using sample data.
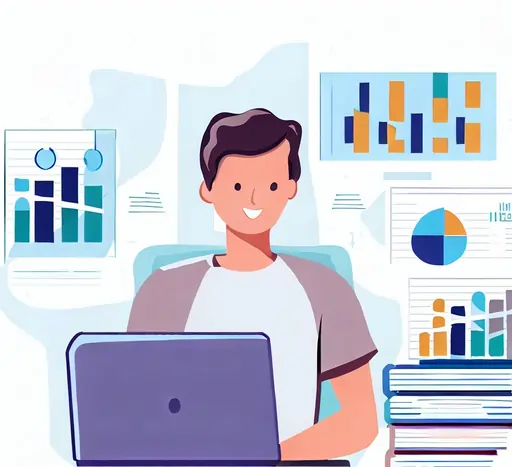
T-Tests for Comparing Means
T-tests are vital tools in inferential statistics. They help assess if there's a significant difference in means between two groups (independent samples) or related measurements (paired samples). Understanding when and how to use these tests is essential for university students tackling SPSS assignments, enabling them to make informed data-driven decisions. They come in two primary forms:
- Independent Samples T-Test
- Paired Samples T-Test
The independent samples t-test is a cornerstone of inferential statistics, especially in SPSS assignments. It serves to compare the means of two distinct and unrelated groups, revealing if there's a statistically significant difference between them. This test is indispensable in numerous research scenarios, from analyzing the impact of interventions in medical studies to investigating the effectiveness of marketing strategies in business. By mastering the independent samples t-test, students can confidently evaluate group differences, make data-driven conclusions, and enhance their skills in conducting rigorous statistical analyses using SPSS.
The paired samples t-test is a fundamental statistical technique used when dealing with related measurements within the same group. In SPSS assignments, it's invaluable for determining if there's a statistically significant difference between two sets of paired observations, often taken before and after an intervention or treatment. This test is commonly employed in fields like medicine and psychology to assess the effectiveness of interventions or to gauge changes over time. Mastery of the paired samples t-test equips university students with the skills needed to draw meaningful conclusions from paired data, making it an indispensable tool for statistical analysis.
Analysis of Variance (ANOVA)
ANOVA is a powerful statistical technique used to compare the means of more than two groups. In SPSS assignments, you may encounter situations where you need to analyze data from multiple groups to determine if there is a significant difference between them. ANOVA can be broadly categorized into three types:
- One-Way ANOVA
- Two-Way ANOVA
The One-Way Analysis of Variance (ANOVA) is a pivotal statistical method in inferential statistics. In SPSS assignments, it's essential when comparing the means of more than two groups with one independent variable. Whether you're analyzing survey data across different demographics or evaluating the impact of various teaching methods on student performance, One-Way ANOVA allows for robust group comparisons. Mastering this technique empowers university students to discern significant differences among multiple groups, enabling data-driven decision-making and a deeper understanding of complex research questions.
Two-way ANOVA is a versatile statistical method frequently encountered in SPSS assignments. It's used when there are two independent variables, allowing students to assess how these factors interact and influence a dependent variable. This technique is invaluable in experimental design, as it enables the exploration of complex relationships between variables. University students mastering two-way ANOVA not only enhance their analytical capabilities but also gain a deeper understanding of the multifaceted nature of statistical analysis, preparing them for more advanced research and real-world applications in various fields, from biology to social sciences.
In cases where the same subjects are used for all treatment conditions, such as in longitudinal studies, repeated measures ANOVA is employed to analyze within-subject changes over time.
Chi-Squared Tests for Categorical Data
Chi-squared tests are indispensable for analyzing categorical data relationships. They help determine if observed and expected frequencies significantly differ, or if two categorical variables are independent. Mastering these tests is crucial for university students dealing with SPSS assignments, empowering them to uncover meaningful insights from categorical data and make informed decisions in various fields like social sciences, healthcare, and market research.
- Chi-Squared Goodness of Fit Test
- Chi-Squared Test of Independence
The Chi-Squared Goodness of Fit Test is a pivotal tool in inferential statistics, particularly for assessing whether observed data fits an expected distribution. In SPSS assignments, this test helps students determine if the frequencies they observe align with theoretical expectations, making it crucial in validating hypotheses and ensuring data accuracy. A solid grasp of this test enables university students to confidently navigate research scenarios where fitting data to an expected model is key, enhancing their analytical prowess in diverse academic disciplines and practical applications.
The Chi-Squared Test of Independence is an essential statistical tool for assessing relationships between two categorical variables. In SPSS assignments, this test is invaluable when investigating whether two variables are dependent or independent of each other. It's widely used in diverse research domains, including sociology, epidemiology, and marketing, where understanding the association between categorical factors is crucial. Proficiency in the Chi-Squared Test of Independence equips university students with the skills to unravel complex patterns within categorical data, enabling them to draw meaningful conclusions and contribute to data-driven decision-making.
Key Considerations for Successful Application
Now that we've explored essential inferential statistical techniques, it's crucial to delve into the key considerations that can enhance your success when applying these methods in SPSS assignments.
- Data Preparation:Data preparation is the foundational step in statistical analysis using SPSS. Thoroughly clean, validate, and format your dataset to ensure its integrity. Address missing values, outliers, and inconsistencies that can skew results. Properly coding variables and ensuring they match your research objectives is crucial. Accurate data preparation not only promotes the reliability of your analysis but also streamlines the entire process. By dedicating time to this phase, university students can set a strong foundation for successful inferential statistics in their SPSS assignments, leading to more accurate and meaningful conclusions.
- Assumptions:Understanding and validating the assumptions underlying your chosen statistical test is vital for reliable results. In SPSS assignments, it's essential to assess factors like data normality, homogeneity of variances, and independence of observations. If these assumptions are not met, consider data transformations or alternative tests to ensure the validity of your analysis. Careful scrutiny of assumptions not only strengthens the credibility of your findings but also demonstrates a comprehensive understanding of statistical methodology, a valuable skill for university students pursuing data-driven research and decision-making.
- Sample Size: The significance of sample size cannot be overstated in statistical analysis. In SPSS assignments, choosing an appropriate sample size is critical. Small samples may lead to unreliable results, while excessively large ones can be impractical. Conducting a power analysis can help determine the sample size needed to detect meaningful effects. Always ensure that your sample size aligns with the research question and the statistical test's requirements, striking a balance between precision and feasibility to yield reliable outcomes in your SPSS assignments.
- Hypothesis Formulation:A well-crafted hypothesis is the backbone of any statistical analysis in SPSS assignments. Start by clearly stating your null hypothesis (H0) and alternative hypothesis (H1). The null hypothesis typically represents the absence of an effect, while the alternative posits the presence of an effect. Ensuring these hypotheses are specific and testable sets the stage for a precise analysis. A carefully formulated hypothesis guides your entire study, directing your choice of statistical tests and helping you draw meaningful conclusions based on empirical evidence, making it a critical component of sound statistical practice.
- Effect Size:In the realm of inferential statistics, effect size is often overshadowed by p-values, but it holds immense importance. It quantifies the magnitude of the observed difference, providing context to statistical significance. By focusing on effect size in SPSS assignments, you can discern whether the results have real-world relevance. Common effect size measures include Cohen's d for t-tests, eta-squared for ANOVA, and Cramer's V for chi-squared tests. Embracing effect size analysis enhances the depth of your statistical interpretations, making your findings more meaningful and applicable in practical scenarios.
- Post-Hoc Tests:In the realm of inferential statistics, post-hoc tests play a critical role in refining your analysis. These tests are particularly valuable when you're dealing with multiple groups, such as in Analysis of Variance (ANOVA). Post-hoc tests, like Tukey's HSD, Bonferroni, or Scheffé, help pinpoint which specific groups exhibit significant differences after an ANOVA suggests a significant overall effect. By using post-hoc tests judiciously in your SPSS assignments, you can offer a more nuanced and accurate interpretation of your data, enhancing the depth and reliability of your findings.
- Interpretation: Interpreting statistical results is the cornerstone of making meaningful conclusions in your SPSS assignments. Beyond just stating whether a result is statistically significant, delve into the practical implications. Effective interpretation not only showcases your analytical skills but also ensures your work contributes to a deeper understanding of the subject matter, making your assignments more valuable to your academic community.
- Visualizations:Visual representations are invaluable in conveying the essence of your statistical findings. In SPSS assignments, consider creating histograms, scatterplots, or box plots to showcase the distribution of data, relationships between variables, and the impact of different treatments or conditions. Visualizations not only make your results more accessible but also help identify patterns or outliers that might be missed in raw data. They enhance the comprehensibility and impact of your assignments, making it easier for both you and your audience to interpret and appreciate the significance of your statistical analyses.
- Documentation: Accurate and organized documentation is the backbone of any successful statistical analysis in SPSS assignments. A well-maintained record of your data transformations, variable definitions, and analysis steps ensures transparency and reproducibility. It also aids in troubleshooting and collaboration with peers or instructors. Proper documentation not only showcases your diligence but also safeguards against errors and enhances the credibility of your findings. Embrace the habit of meticulous record-keeping to streamline your workflow and facilitate a clearer understanding of your statistical journey.
- Seek Guidance:When navigating the complexities of inferential statistics and SPSS, seeking guidance is a wise strategy. Don't hesitate to consult your professors, teaching assistants, or academic peers when faced with challenging assignments. Online forums, tutorials, and textbooks can also provide valuable insights. Collaboration and learning from others' experiences can shed light on nuances, offer alternative perspectives, and ultimately strengthen your statistical prowess, ensuring you approach SPSS assignments with confidence and competence.
By paying attention to these considerations, you can elevate the quality of your SPSS assignments, making your statistical analyses more robust, insightful, and impactful. Remember that practice and continual learning are key to becoming proficient in inferential statistics and effectively using SPSS as a valuable tool in your academic journey.
Conclusion
Inferential statistics, with its t-tests, ANOVA, and chi-squared tests, plays a pivotal role in the field of data analysis and is indispensable for university students working on SPSS assignments. By mastering these techniques, you will not only be better equipped to analyze data effectively but also gain the confidence needed to excel in your academic pursuits. As you venture further into the world of statistics and SPSS, remember that practice and real-world application are the keys to solidifying your understanding. Don't hesitate to seek additional resources, consult with professors, and explore practical examples to enhance your skills. The journey to becoming a proficient SPSS user starts with a firm grasp of inferential statistics, and this guide is your first step towards that goal. So go ahead, do your SPSS assignment, and unlock the power of data analysis.