Avail Your Offer
Unlock success this fall with our exclusive offer! Get 20% off on all statistics assignments for the fall semester at www.statisticsassignmenthelp.com. Don't miss out on expert guidance at a discounted rate. Enhance your grades and confidence. Hurry, this limited-time offer won't last long!
We Accept
- Understanding the Assignment Requirements
- Analyzing the Problem Statement
- Defining the Scope of Work
- Exploratory Data Analysis (EDA)
- Initial Data Exploration
- Data Visualization
- Dealing with Missing Values and Outliers
- Handling Missing Values
- Identifying and Treating Outliers
- Statistical Analysis
- Correlation and Causation
- Regression Analysis
- Data Visualization and Interpretation
- Effective Data Visualization
- Interpreting Results
- Developing a Business Plan
- Integrating Data Insights into Strategy
- Conclusion
Data analysis assignments are a staple in statistics courses, offering students practical experience in handling, processing, and interpreting data. These assignments are critical as they provide a hands-on approach to understanding complex statistical concepts and applying them in real-world scenarios. Whether you’re interning at a ride-hailing company analyzing demand patterns or advising a government on public health issues like life expectancy, mastering the art of data analysis is crucial. In this blog, we will delve into key strategies that can help with data analysis assignments, illustrated with hypothetical scenarios that mirror those you might encounter in your coursework or professional experience. Our focus will be on developing a robust, adaptable methodology that can be applied to various datasets and questions, ensuring you can confidently tackle any data analysis task that comes your way. By the end, you will have a comprehensive guide to help you excel in your statistics assignments.
Understanding the Assignment Requirements
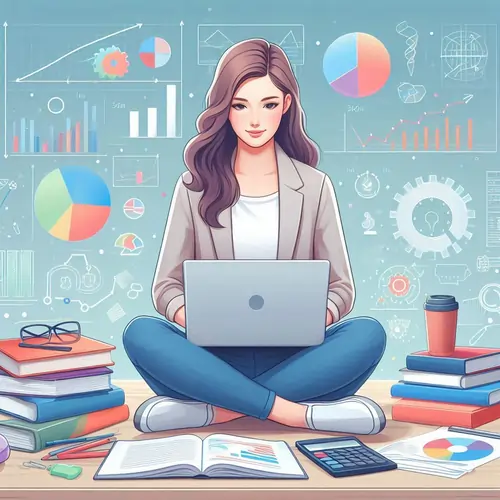
Before diving into any data analysis, it’s crucial to fully grasp the assignment requirements. This involves carefully reading and dissecting the problem statement to identify the objectives, scope, and specific questions that need to be addressed. For instance, if you're tasked with analyzing ride demand for a ride-hailing company based on weather and temporal factors, understanding the dataset variables such as pickup times, weather conditions, and geographic locations becomes paramount. By comprehensively understanding these requirements, you can effectively plan your data analysis approach, ensuring that your methodologies and interpretations align closely with the assignment's objectives. This section will guide you through the initial steps necessary to set a solid foundation for your data analysis journey.
Analyzing the Problem Statement
Before diving into any data analysis, it’s essential to thoroughly understand the problem statement. The problem statement provides the context, objectives, and specific questions you need to address. For instance, let’s consider a scenario where you’re asked to analyze ride demand for a ride-hailing company in New York City, like in the example assignment provided:
- Context:You’re a data science intern at a ride-hailing company.
- Objective:Analyze six months’ worth of ride data to understand demand.
- Data Variables: Variables include pickup date and time, borough, number of pickups, wind speed, visibility, temperature, dew point, sea level pressure, precipitation, snow depth, holiday indicator, and date components.
Defining the Scope of Work
Once you’ve grasped the context and objectives, define the scope of your analysis. This includes determining the key questions you need to answer, and the types of analyses required. For the ride-hailing company example, your scope might include:
- Descriptive Analysis: Summarize the data to understand general trends.
- Exploratory Data Analysis (EDA):Visualize data to uncover patterns and relationships.
- Statistical Analysis:Identify factors influencing ride demand.
- Business Recommendations:Develop strategies to improve ride demand based on your findings.
Exploratory Data Analysis (EDA)
Exploratory Data Analysis (EDA) is a crucial step in any data analysis project, serving as the foundation for deeper insights and conclusions. During this phase, you explore the dataset to understand its structure, identify patterns, and uncover relationships between variables. EDA involves both statistical methods and visualizations to help you get a sense of the data’s distribution, detect anomalies, and assess initial hypotheses.
Initial Data Exploration
EDA is a crucial step in any data analysis process. It involves summarizing the main characteristics of the data, often with visual methods. For our ride-hailing example, initial data exploration might involve:
Summary Statistics
Calculate basic summary statistics for key variables:
- Mean, median, mode, standard deviation, and range for numerical variables like number of pickups, temperature, and wind speed.
- Frequency counts for categorical variables like borough and holiday indicator.
Data Visualization
Visualize the data using appropriate charts:
- Histograms for distribution of numerical variables.
- Bar charts for categorical variables.
- Line charts to show trends over time.
- Scatter plots to examine relationships between variables.
Dealing with Missing Values and Outliers
Data cleaning is an integral part of EDA. Identify and handle missing values and outliers, as they can significantly affect your analysis.
Handling Missing Values
- Imputation: Replace missing values with mean, median, or mode.
- Deletion: Remove rows or columns with excessive missing values.
Identifying and Treating Outliers
- Visualization: Use box plots to identify outliers.
- Statistical Methods: Apply Z-scores or IQR methods to flag outliers.
- Treatment: Decide whether to remove or adjust outliers based on their impact on the analysis.
Statistical Analysis
Statistical analysis forms the backbone of any data-driven investigation, offering quantitative insights into relationships and patterns within the data. This phase typically involves applying mathematical models and techniques to interpret data, uncover correlations, and derive meaningful conclusions. For instance, in a scenario analyzing ride demand, statistical analysis may include regression models to understand how variables like weather conditions and time of day influence ride bookings. By employing rigorous statistical methods, you can validate hypotheses, identify significant factors impacting outcomes, and ultimately provide data-backed recommendations. This section will explore various statistical techniques that can be employed to extract actionable insights from your datasets, ensuring robust and reliable analysis outcomes.
Correlation and Causation
Understanding the relationship between variables is key to making data-driven decisions. Use correlation analysis to identify relationships between numerical variables.
Correlation Matrix
- Heatmaps:Visualize the correlation matrix using a heatmap to identify strong relationships.
- Pearson/Spearman Correlation:Calculate correlation coefficients to quantify the strength and direction of relationships.
Identifying Causal Relationships
Correlation does not imply causation. Use statistical techniques like regression analysis to explore potential causal relationships.
Regression Analysis
Regression analysis helps in understanding how the dependent variable changes with the independent variables.
Linear Regression
For the ride-hailing example, you might use linear regression to predict the number of pickups based on weather conditions and time variables.
- Model Fitting:Fit a linear regression model using variables like temperature, wind speed, and precipitation.
- Interpretation:Interpret the coefficients to understand the impact of each variable on ride demand.
Multiple Regression
Incorporate multiple independent variables to account for various factors affecting the dependent variable.
- Model Building:Include variables like borough, holiday indicator, and time of day in the regression model.
- Multicollinearity:Check for multicollinearity using Variance Inflation Factor (VIF) and address it if necessary.
Data Visualization and Interpretation
Data visualization is a critical component of data analysis that helps you to present your findings in a clear and compelling manner. Effective visualizations can reveal patterns, trends, and relationships in the data that might not be immediately obvious from raw numbers alone. This process involves selecting appropriate types of charts and graphs, such as histograms, bar charts, and scatter plots, to represent your data visually.
Effective Data Visualization
Creating effective visualizations is crucial for communicating your findings. Choose the right type of chart based on the data and the message you want to convey.
Time Series Analysis
- Line Charts: Use line charts to show trends in ride demand over time.
- Seasonal Decomposition: Decompose time series data to identify seasonal patterns and trends.
Comparative Analysis
- Bar Charts:Compare ride demand across different boroughs or time periods using bar charts.
- Box Plots:Use box plots to compare the distribution of ride demand across holidays and non-holidays.
Interpreting Results
Interpret your results in the context of the business problem. Provide clear and actionable insights.
Summarizing Findings
- Key Insights: Summarize the key insights from your analysis, such as peak demand times, impact of weather conditions, and differences across boroughs.
- Data-Driven Recommendations: Develop recommendations based on your findings. For instance, suggest increasing the number of drivers during peak demand times or in specific boroughs.
Communicating to Non-Technical Audience
- Simplify Complex Concepts: Use simple language to explain complex statistical concepts.
- Focus on Business Implications: Emphasize how your findings can help improve business operations and decision-making.
Developing a Business Plan
Once you’ve completed your data analysis, the next crucial step is to develop a business plan based on your findings. This phase involves translating your data insights into actionable strategies that can address the core business problem. Whether your analysis revealed trends in ride demand or factors affecting life expectancy, the business plan should outline clear, data-driven recommendations for improving outcomes.
To develop a robust business plan, start by identifying the key insights from your analysis, such as peak demand times or significant predictors of life expectancy. Use these insights to propose targeted strategies, such as optimizing resource allocation, enhancing services, or implementing new policies. Additionally, establish a framework for monitoring the effectiveness of your recommendations through measurable key performance indicators (KPIs) and create a feedback loop for continuous improvement. This section will provide you with the tools and techniques to craft a strategic plan that leverages your data analysis for tangible business results.
Integrating Data Insights into Strategy
The ultimate goal of data analysis is to inform business strategy. Use your data insights to develop a comprehensive business plan.
Strategic Recommendations
Based on the ride-hailing example, your business plan might include:
- Operational Improvements:Recommend optimizing driver allocation based on demand patterns.
- Marketing Strategies: Suggest targeted promotions during low-demand periods or in specific boroughs.
- Service Enhancements: Propose new services or features based on customer preferences and demand trends.
Monitoring and Evaluation
Establish a framework for monitoring and evaluating the effectiveness of your recommendations.
- Key Performance Indicators (KPIs): Define KPIs to track the impact of your strategies, such as ride completion rate, customer satisfaction, and revenue growth.
- Feedback Loop: Implement a feedback loop to continuously refine your strategies based on new data and insights.
Conclusion
Data analysis assignments are an excellent way to apply your statistical knowledge to real-world problems. By following a structured approach, from understanding the problem statement to developing actionable business recommendations, you can effectively tackle any data analysis assignment. Remember to communicate your findings clearly, especially to non-technical stakeholders, and always base your recommendations on solid data insights. Whether you’re working on ride demand analysis or life expectancy studies, these principles will guide you towards producing high-quality, impactful reports.