Avail Your Offer Now
Celebrate the festive season with an exclusive holiday treat! Enjoy 15% off on all orders at www.statisticsassignmenthelp.com this Christmas and New Year. Unlock expert guidance to boost your academic success at a discounted price. Use the code SAHHOLIDAY15 to claim your offer and start the New Year on the right note. Don’t wait—this special offer is available for a limited time only!
We Accept
- Pre-Data Analysis
- Defining the Research Question and Operational Definitions
- Operational Definitions:
- Selecting the Research Methodology/Design
- Conducting an A Priori Power Analysis
- Data Analysis
- Formulating Hypotheses
- Testing for Assumptions of Correlation
- Running the Analysis and Recording Results
- Making a Decision
- Post-Data Analysis
- Interpreting the Effect Size (r²)
- Determining the Power of the Study
- Calculating the 95% Confidence Interval
- Explaining the Results
- Conclusion
Bivariate correlation is a fundamental statistical technique essential for examining the relationship between two variables. It plays a critical role in academic assignments where students are often required to explore and analyze these relationships within different contexts. Whether you're investigating the link between pilot experience and aviation safety or any other pair of variables, understanding how to effectively apply bivariate correlation is crucial. If you're looking for help with a bivariate correlation assignment, this blog will provide a comprehensive overview of the steps necessary to complete such tasks. By following these guidelines, you can gain valuable insights and perform rigorous analysis to uncover meaningful relationships in your data.
Pre-Data Analysis
Before diving into the data, it's essential to set the stage with a thorough pre-data analysis. This step ensures that you have a clear research question, appropriate methodology, and adequate sample size, all of which are critical for producing reliable and meaningful results.
Defining the Research Question and Operational Definitions
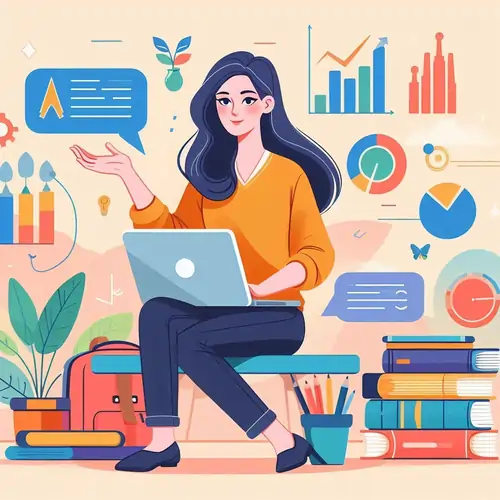
The first step in any statistical analysis is to clearly define your research question and operational definitions. For a study examining the relationship between pilot experience and aviation safety, your research question might be: "Is there a significant relationship between pilot experience and aviation safety?"
Operational Definitions:
- Pilot Experience: Total flight hours in all aircraft.
- Aviation Safety: Scores on the Aviation Safety Index (ASI), which consists of five safety-related statements. Each statement is rated on a 7-point scale, with aggregate scores ranging from 5 to 35. Higher scores indicate more safety-conscious pilots.
Clearly defining these variables ensures that you and your audience understand exactly what is being measured and analyzed.
Selecting the Research Methodology/Design
Choosing the appropriate research design is critical to the success of your analysis. For correlation studies, a non-experimental, cross-sectional design is typically used. This design is appropriate because it allows you to examine the relationship between two variables without manipulating them.
Justification for the Methodology:
- Non-experimental Design: Since the study aims to observe and analyze existing data without intervention, a non-experimental design is suitable.
- Cross-Sectional Design: This approach is effective for studying the relationship between variables at a single point in time, providing a snapshot of the correlation between pilot experience and aviation safety.
Conducting an A Priori Power Analysis
An a priori power analysis helps determine the minimum sample size needed for your study to detect a significant effect. This step is crucial for ensuring that your study has enough statistical power.
Steps to Conduct Power Analysis:
- Specify the Effect Size: Estimate the expected effect size based on previous research or pilot studies. For correlation studies, a small effect size might be around 0.1, a medium effect size around 0.3, and a large effect size around 0.5.
- Choose the Significance Level (α): Commonly set at 0.05.
- Set the Power (1-β): A typical value for power is 0.80, meaning there is an 80% chance of detecting a significant effect if it exists.
Using software like G Power, input these parameters to calculate the required sample size. Compare this with your actual sample size to assess if it is sufficient. Discuss how sample size impacts the reliability and validity of your results. Larger sample sizes generally provide more reliable results, reducing the margin of error.
Data Analysis
Data analysis is the core of any statistical assignment. This section involves multiple steps, from formulating hypotheses to testing assumptions and running the analysis itself. Each step plays a crucial role in ensuring the validity and reliability of your results. By systematically addressing each aspect of data analysis, you can draw meaningful conclusions from your data and provide a robust answer to your research question.
Formulating Hypotheses
Formulating clear and testable hypotheses is a fundamental step in data analysis.
- Null Hypothesis (H0): There is no significant relationship between pilot experience and aviation safety.
- Alternative Hypothesis (H1): There is a significant relationship between pilot experience and aviation safety.
Determining the Test Criteria
The next step is to determine the test criteria. This includes selecting the appropriate statistical test and setting the significance level.
- Statistical Test: For correlation analysis, Pearson’s correlation coefficient is commonly used.
- Significance Level (α): Typically set at 0.05.
Testing for Assumptions of Correlation
Before conducting the correlation analysis, it is important to test for the assumptions underlying the Pearson correlation:
- Linearity: The relationship between the variables should be linear. This can be checked using scatter plots.
- Homoscedasticity: The variability of one variable should be constant across the range of the other variable. Residual plots can help assess this assumption.
- Normality: The data should be approximately normally distributed. This can be evaluated using normal probability plots or tests like the Shapiro-Wilk test.
- Outliers: Outliers can significantly impact correlation results. Use techniques like Jackknife distances to identify and assess outliers.
Running the Analysis and Recording Results
Once the assumptions are verified, proceed with the correlation analysis using your statistical software. Here’s a step-by-step guide:
- Import Data: Load the data set into your statistical software.
- Calculate Correlation Coefficient: Use the software to compute Pearson’s correlation coefficient.
- Record Results: Document the correlation coefficient (r), p-value, and any additional statistics provided by the software.
Making a Decision
Based on the p-value obtained from the correlation analysis, make a decision regarding the null hypothesis:
- If p-value ≤ 0.05: Reject the null hypothesis. There is evidence to suggest a significant relationship between the variables.
- If p-value > 0.05: Fail to reject the null hypothesis. There is no sufficient evidence to suggest a significant relationship.
Concluding Statement: Summarize your findings in a clear and concise manner. For example, "The analysis revealed a significant positive relationship between pilot experience and aviation safety (r = 0.45, p < 0.05), suggesting that pilots with more flight hours tend to exhibit safer behaviors."
Post-Data Analysis
Post-data analysis involves interpreting and validating your findings to ensure they are meaningful and robust. This section provides insights into how to interpret effect size, assess the power of your study, calculate confidence intervals, and offer plausible explanations for your results. By thoroughly examining these aspects, you can present a comprehensive analysis that highlights the significance and reliability of your findings. This not only strengthens your conclusions but also provides valuable context and depth to your statistical analysis.
Interpreting the Effect Size (r²)
Effect size provides insight into the strength of the relationship between the variables. In correlation analysis, the effect size is represented by r², the coefficient of determination.
- Calculation: r² = (correlation coefficient)².
- Interpretation: r² indicates the proportion of variance in the dependent variable (aviation safety) explained by the independent variable (pilot experience). For example, if r = 0.45, then r² = 0.2025, meaning approximately 20.25% of the variance in aviation safety can be explained by pilot experience.
Determining the Power of the Study
Power analysis post-data collection helps confirm whether your study had adequate power to detect a significant effect.
- Power Calculation: Use statistical software to calculate the post-hoc power based on your sample size, effect size, and significance level.
- Interpretation: Aim for a power of 0.80 or higher. If your study’s power is below this threshold, consider discussing the implications, such as increased risk of Type II errors (failing to detect a true effect).
Calculating the 95% Confidence Interval
Confidence intervals provide a range within which the true correlation coefficient is likely to fall.
- Calculation: Most statistical software will provide the 95% confidence interval for the correlation coefficient.
- Interpretation: A narrow confidence interval indicates a more precise estimate of the correlation coefficient. For instance, a 95% confidence interval of 0.30 to 0.60 for a correlation coefficient of 0.45 suggests a reasonably precise estimate.
Explaining the Results
Offering plausible explanations for your findings enhances the depth of your analysis. Here are some potential factors to consider:
- Data Quality: Discuss the reliability and validity of your data. High-quality data enhances the credibility of your results.
- Sample Characteristics: Consider the demographics and other characteristics of your sample. For example, if your sample predominantly consists of highly experienced pilots, this might influence the results.
- Confounding Variables: Identify any potential confounding variables that could affect the relationship between your variables. For example, factors like pilot training programs or aircraft type might influence both pilot experience and aviation safety.
Conclusion
In conclusion, effectively tackling assignments that involve bivariate correlation requires a methodical and systematic approach. By thoroughly defining your research question and operational definitions, selecting the most appropriate research design, and conducting a comprehensive power analysis, you lay a strong foundation for your analysis. Rigorous data analysis, including testing assumptions, formulating hypotheses, and interpreting results accurately, is crucial for drawing meaningful and reliable conclusions. Remember to place your findings within the broader context of your study and carefully consider plausible explanations for your results. This structured approach not only enhances the quality of your analysis but also equips you with the skills needed to solve your statistics assignment with confidence. By following these steps diligently, you will be well-prepared to address similar statistical challenges and excel in your academic endeavors.