Claim Your Offer
Unlock an exclusive deal at www.statisticsassignmenthelp.com with our Spring Semester Offer! Get 10% off on all statistics assignments and enjoy expert assistance at an affordable price. Our skilled team is here to provide top-quality solutions, ensuring you excel in your statistics assignments without breaking the bank. Use Offer Code: SPRINGSAH10 at checkout and grab this limited-time discount. Don’t miss the chance to save while securing the best help for your statistics assignments. Order now and make this semester a success!
We Accept
Statistics, integral across diverse fields like science and economics, serves as a vital tool for interpreting data and extracting valuable insights. Within the realm of statistics, a pivotal concept is probability distributions, essential for comprehending the likelihood of diverse outcomes in random experiments. This blog delves into the intricacies of the binomial distribution, a foundational probability distribution model. Our exploration centers on the calculation of its mean and variance, critical metrics that illuminate the expected values and data spread. This knowledge empowers students to confidently navigate assignments involving binomial distributions, providing them with the analytical tools to decipher real-world scenarios and draw informed conclusions grounded in probability theory. Understanding these statistical foundations enhances problem-solving skills and equips students to make informed decisions across various disciplines.
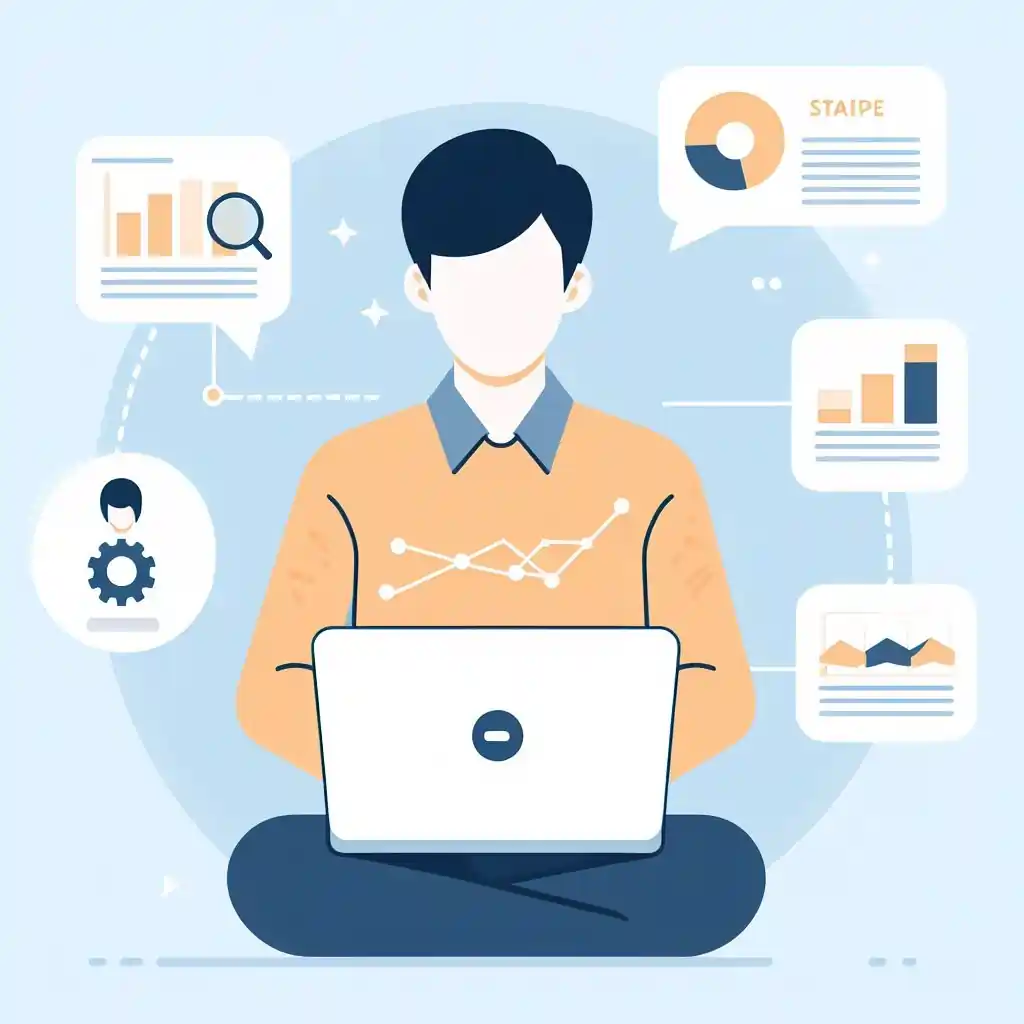
What is a Binomial Distribution?
The binomial distribution is a foundational concept in probability theory, crucially applicable in numerous fields, including biology, finance, and quality control. It provides a robust framework for understanding and predicting outcomes in situations where discrete events have just two potential results: success or failure. This distribution models the probability of a given number of successes in a fixed number of independent trials, with a constant probability of success. Its versatility makes it invaluable for decision-making, risk assessment, and hypothesis testing, making it a cornerstone of statistical analysis in both academic and practical settings.
Fixed Number of Trials (n):
Central to the binomial distribution is the notion of a fixed number of trials, often represented as 'n'. These trials can be thought of as a sequence of independent, identical experiments, each with the same probability of success 'p'. For instance, when conducting quality control tests on a production line, 'n' might represent the number of items inspected, and each trial aims to determine whether an item meets the quality standard ('success') or not ('failure').
Two Possible Outcomes:
In any binomial experiment, every individual trial possesses two exclusive outcomes – success (S) and failure (F). This binary nature simplifies the modeling of complex systems, making it a go-to choice for scenarios where the result is either 'yes' or 'no,' 'heads' or 'tails,' or 'accept' or 'reject.' The simplicity of this dichotomous outcome structure enables clarity in probability analysis.
Independence:
Another critical feature of the binomial distribution is the independence of trials. Each experiment or trial does not influence the outcome of others. For instance, in a medical study evaluating the efficacy of a drug, the response of one patient does not impact the response of another. This independence assumption simplifies the modeling process and makes the binomial distribution a suitable choice for numerous real-world applications.
Constant Probability of Success (p):
Perhaps the most defining characteristic of the binomial distribution is the constancy of the probability of success, denoted as 'p.' Regardless of the number of trials conducted, 'p' remains the same throughout. This characteristic is particularly relevant in scenarios where the conditions under which the trials are performed remain consistent. For instance, when flipping a fair coin, the probability of getting heads ('success') remains at 0.5 for each flip, irrespective of previous outcomes.
Now, let's explore how to compute the mean and variance of a binomial distribution to aid students in solving assignments.
Computing the Mean of a Binomial Distribution
To compute the mean of a binomial distribution, also known as the expected value, the formula employed is essential for understanding the central tendency of this discrete probability distribution. This formula, which combines the number of trials, the probability of success in each trial, and the random variable, provides a valuable statistical insight. By utilizing this mean calculation, statisticians and data analysts can gauge the average outcome of repeated binomial experiments, aiding in making informed decisions and predictions in fields ranging from finance to healthcare. The mean, often referred to as the expected value, of a binomial distribution can be calculated using the following formula:
Mean (μ) = n * p
Where:
n is the number of trials.
p is the probability of success in each trial.
Steps to Calculate the Mean
Identify the number of trials, 'n', in the given problem.
Determine the probability of success, 'p', for each trial.
Multiply 'n' by 'p' to find the mean.
Let's consider an example to illustrate this. Suppose you are conducting 20 independent coin flips, and the probability of getting heads (success) in each flip is 0.5. To find the mean:
Mean (μ) = 20 * 0.5 = 10
So, the mean number of heads you would expect in 20 coin flips is 10.
Computing the Variance of a Binomial Distribution
The variance of a binomial distribution serves as a pivotal metric in assessing the extent of data dispersion within this discrete probability model. This statistical measure, derived from the number of trials, the probability of success, and the random variable, provides valuable insights into the distribution's variability. By employing this variance formula, analysts gain a nuanced understanding of the distribution's inherent variability, aiding in risk assessment and decision-making processes across diverse domains, from quality control in manufacturing to predicting outcomes in experimental studies. For a binomial distribution, the variance can be calculated using the following formula:
Variance (σ^2) = n * p * (1 - p)
Where:
n is the number of trials.
p is the probability of success in each trial.
(1 - p) is the probability of failure in each trial.
Steps to Calculate the Variance
Identify the number of trials, 'n', in the given problem.
Determine the probability of success, 'p', for each trial.
Calculate the probability of failure, which is '1 - p'.
Multiply 'n' by 'p' and '(1 - p)' to find the variance.
Let's use the same example to compute the variance. In 20 coin flips with a probability of 0.5 for heads:
Variance (σ^2) = 20 * 0.5 * (1 - 0.5) = 5
So, the variance in the number of heads you would expect in 20 coin flips is 5.
Solving Binomial Distribution Problems
Now that we've learned how to compute the mean and variance of a binomial distribution, let's apply this knowledge to solve some problems. Here are a couple of example scenarios:
Example 1: Coin Flips
Suppose you want to find the mean and variance of the number of heads in 50 coin flips, where the probability of getting heads in each flip is 0.6.
Solution:
Number of trials, 'n' = 50.
Probability of success, 'p' = 0.6.
Mean (μ) = 50 * 0.6 = 30
Variance (σ^2) = 50 * 0.6 * (1 - 0.6) = 12
So, in 50 coin flips with a 0.6 probability of heads, the mean number of heads is 30, and the variance is 12.
Example 2: Exam Success
Imagine you have an exam with 30 multiple-choice questions, each with four options. If you guess the answers to all the questions, what is the mean and variance of the number of correct answers?
Solution:
Number of trials, 'n' = 30 (number of questions).
Probability of success, 'p' = 1/4 (probability of guessing the correct answer for each question).
Mean (μ) = 30 * (1/4) = 7.5
Variance (σ^2) = 30 * (1/4) * (1 - 1/4) = 5.625
So, when guessing answers to 30 multiple-choice questions with four options each, the mean number of correct answers is 7.5, and the variance is approximately 5.625.
Conclusion
Understanding the binomial distribution is essential in probability and statistics. It allows us to model the number of successes in a fixed number of independent trials with two possible outcomes. Computing the mean and variance of a binomial distribution helps us gain insights into the expected values and the spread of data. By following the steps and formulas provided in this blog, students can confidently tackle assignments involving binomial distributions, making them better equipped to analyze real-world scenarios and draw meaningful conclusions based on probability theory.