Avail Your Offer
Unlock success this fall with our exclusive offer! Get 20% off on all statistics assignments for the fall semester at www.statisticsassignmenthelp.com. Don't miss out on expert guidance at a discounted rate. Enhance your grades and confidence. Hurry, this limited-time offer won't last long!
We Accept
- Understanding the Research Question and Data
- Key Steps in Understanding the Data
- Choosing the Right Statistical Method
- Regression Analysis
- Steps for Conducting Regression Analysis
- Correspondence Analysis
- Steps for Conducting Correspondence Analysis
- Experimental Data Analysis
- Steps for Conducting Experimental Data Analysis
- Conducting the Analysis
- Steps for Conducting the Analysis
- Reporting the Findings
- Key Elements of Reporting
- Conclusion
When faced with assignments involving complex statistical analysis, such as regression, correspondence analysis, or experimental data interpretation, it is important to adopt a clear and systematic approach. This blog will provide a comprehensive overview of how to tackle such assignments, using examples that may include investigating relationships between variables, exploring categorical data, and analyzing experimental outcomes. While the specific details of each assignment may vary, the strategies outlined here can be applied broadly to similar tasks, ensuring a thorough understanding and accurate analysis of the data.
Understanding the Research Question and Data
The first and most critical step in any statistical analysis is understanding the research question and the data you are working with. The research question provides the context for your analysis and helps you identify the appropriate statistical methods to use. For example, consider a scenario where an educational psychologist wants to investigate the relationship between anxiety levels and exam performance. The data might include scores from Beck’s Anxiety Inventory (BAI) and students’ exam performance scores.
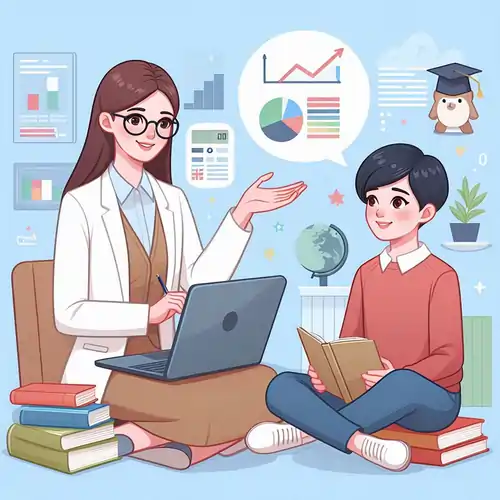
Before diving into the analysis, it is essential to thoroughly explore the dataset. Start by identifying the types of variables you are dealing with—whether they are continuous, categorical, or ordinal. For instance, the BAI scores are continuous, ranging from 0 to 63, and they categorize anxiety into three levels: low, moderate, and extreme. Exam performance scores are also continuous. Understanding the nature of your variables will guide your choice of statistical methods.
Key Steps in Understanding the Data
- Clarify the Research Hypothesis: Begin by clearly defining the hypothesis. For example, the hypothesis could be that higher anxiety levels are associated with lower exam performance. Alternatively, in an assignment involving a sport psychologist, the hypothesis might explore the relationship between weight categories and running habits.
- Inspect the Dataset: After clarifying the hypothesis, inspect the dataset for any inconsistencies, missing values, or outliers. For example, if working with SPSS files, open the data and check for completeness. Ensure that all relevant variables are included and that there are no errors in data entry.
- Understand Variable Types and Scales: Identify whether your variables are continuous, ordinal, or categorical. For example, in a correspondence analysis involving weight categories and running habits, the data might be categorical. Understanding these distinctions is crucial for selecting the correct statistical approach.
By fully understanding the research question and data, you lay a solid foundation for the subsequent analysis. This step ensures that your analysis is relevant and accurate, setting you up for success in the later stages of the assignment.
Choosing the Right Statistical Method
Once you have a firm grasp of the research question and data, the next step is to select the appropriate statistical method. The choice of method depends on the nature of the data and the specific objectives of the analysis. In statistical assignments, common methods include regression analysis, correspondence analysis, and experimental data analysis.
Regression Analysis
Regression analysis is commonly used when the objective is to predict one variable based on another. For instance, in the example of an educational psychologist studying anxiety and exam performance, you might use regression analysis to determine whether a linear, quadratic, or cubic relationship best predicts exam performance based on BAI scores.
Steps for Conducting Regression Analysis
- Visualize the Relationship: Begin by creating a scatter plot to visualize the relationship between the predictor (independent) variable and the outcome (dependent) variable. This visualization helps you assess whether the relationship appears linear, quadratic, or cubic.
- Fit Different Models: Using statistical software like SPSS, fit different regression models to the data. Start with a linear model, then explore quadratic and cubic models. Each model will produce different regression coefficients and R-squared values.
- Compare Models: Compare the models based on their R-squared values and the significance of the regression coefficients. The R-squared value indicates how well the model explains the variability in the data, while the p-values associated with the coefficients show whether the relationships are statistically significant.
- Check Model Assumptions: Ensure that the chosen regression model meets the necessary assumptions, such as linearity, homoscedasticity, and normality of residuals. If these assumptions are violated, the model may not be appropriate, and the results could be misleading.
- Interpret the Results: Finally, interpret the regression coefficients to understand the strength and direction of the relationship between variables. For example, a negative coefficient for BAI scores in a linear regression model would indicate that higher anxiety is associated with lower exam performance.
Regression analysis is a powerful tool for understanding relationships between variables. By carefully selecting and fitting the appropriate model, you can gain valuable insights into the data and draw meaningful conclusions.
Correspondence Analysis
Correspondence analysis is used to explore relationships between categorical variables. It is particularly useful when dealing with data that can be arranged in a contingency table, such as exploring the relationship between weight categories and running/drinking habits.
Steps for Conducting Correspondence Analysis
- Create a Contingency Table: Begin by organizing the data into a contingency table that shows the frequency of each combination of categorical variables. For example, in a study of running habits and weight categories, the table would display the number of people in each combination of running habits and weight categories.
- Perform the Correspondence Analysis: Use statistical software like SPSS to perform the correspondence analysis. This method will produce a plot that visualizes the relationships between the categories in a multidimensional space.
- Interpret the Plot: In the correspondence plot, categories that are close together are more similar, indicating a potential relationship. For instance, if "Slightly overweight" individuals are close to "Running more than 4 times a week," it suggests that these individuals are more likely to run frequently.
- Assess the Quality of Representation: Check the quality of representation for each category in the plot. Categories that are well represented in the plot provide reliable information, while those with poor representation might require further investigation.
- Draw Conclusions: Based on the proximity of categories in the plot, draw conclusions about the relationships between the variables. For example, you might conclude that certain weight categories are more likely to engage in frequent running or drinking habits.
Correspondence analysis is a powerful technique for uncovering patterns in categorical data. By visualizing the relationships between categories, you can gain insights into the underlying structure of the data and make informed conclusions.
Experimental Data Analysis
When completing your data analysis assignment, such as in studies examining the effects of different treatments or conditions, it is important to use the appropriate statistical tests to compare group means. For example, in an experiment investigating the effects of maternal separation on weight gain in rats, you might use ANOVA or t-tests to compare the weight gain between different groups.
Steps for Conducting Experimental Data Analysis
- Enter the Data: Begin by entering the data into statistical software like SPSS. In the example of maternal separation and weight gain, the data might include the total weight gained by rats in different groups (e.g., separated vs. not separated) and their cage locations.
- Choose the Appropriate Test: Select the statistical test that is most suitable for comparing the group means. If you have more than two groups, ANOVA is typically used. For two groups, a t-test might be appropriate.
- Run the Analysis: Run the selected test in SPSS to compare the means of the groups. The output will include test statistics (e.g., F-value for ANOVA) and p-values that indicate whether the differences between groups are statistically significant.
- Interpret the Results: Examine the test statistics and p-values to determine if there are significant differences between the groups. For example, if the p-value is below the significance level (e.g., 0.05), you can conclude that there is a significant difference in weight gain between separated and not separated rats.
- Consider Additional Factors: In some experimental designs, other factors (such as cage location) might influence the results. Be sure to account for these factors in your analysis and interpret their effects on the outcome.
Experimental data analysis allows researchers to determine the effects of different treatments or conditions on outcomes. By carefully selecting the appropriate statistical tests and interpreting the results, you can draw meaningful conclusions from experimental data.
Conducting the Analysis
With the research question, data, and statistical method in place, it is time to conduct the analysis. This stage involves inputting the data into statistical software, running the analysis, and interpreting the results. Whether you are conducting regression analysis, correspondence analysis, or experimental data analysis, the process generally follows a similar pattern.
Steps for Conducting the Analysis
- Input the Data: Enter the data into your chosen statistical software (e.g., SPSS). Ensure that the data is correctly formatted, with each variable clearly labeled and categorized.
- Run the Analysis: Depending on the type of analysis, select the appropriate statistical test or model and run the analysis. For example, in regression analysis, you would input the predictor and outcome variables and choose the type of regression model to fit.
- Examine the Output: After running the analysis, carefully examine the output provided by the software. This output will include important statistics, such as regression coefficients, R-squared values, and p-values, which you will need to interpret.
- Check Assumptions: For many statistical methods, it is important to check that the assumptions of the test or model are met. For example, in regression analysis, you should check for linearity, homoscedasticity, and normality of residuals.
- Visualize the Results: Create visualizations (e.g., scatter plots, correspondence plots) to help interpret and present the results. Visual aids are especially important in correspondence analysis, where the plot reveals relationships between categories.
- Interpret the Results: Use the output and visualizations to interpret the results of the analysis. Consider the implications of the findings in the context of the research question and hypothesis.
Conducting the analysis is the most technical part of the assignment. By carefully following the steps outlined above, you can ensure that your analysis is accurate and that the results are meaningful.
Reporting the Findings
The final step in any statistics assignment is to report the findings. This involves summarizing the results of your analysis, discussing their implications, and drawing conclusions. Whether you are writing a report, preparing a presentation, or submitting an assignment, it is important to communicate your findings clearly and effectively.
Key Elements of Reporting
- Summary of the Analysis: Begin by summarizing the research question, data, and statistical methods used in the analysis. For example, if you conducted a regression analysis, briefly describe the predictor and outcome variables and the type of model fitted.
- Presentation of Results: Present the results of the analysis, including key statistics and visualizations. For example, in regression analysis, report the regression coefficients, R-squared values, and p-values. In correspondence analysis, include the correspondence plot and interpret its key features.
- Interpretation of Findings: Discuss the implications of the findings in the context of the research question. For example, if you found a significant relationship between anxiety and exam performance, discuss what this means for the research hypothesis and the broader context of the study.
- Discussion of Limitations: Acknowledge any limitations of the analysis, such as violations of model assumptions or limitations in the dataset. For example, if the residuals in a regression analysis were not normally distributed, discuss how this might affect the validity of the results.
- Conclusion: Conclude by summarizing the key findings and their implications. Consider the broader impact of the results and suggest areas for further research or analysis.
Reporting the findings is an essential part of any statistical assignment. By clearly communicating the results and their implications, you can demonstrate a thorough understanding of the analysis and contribute valuable insights to the field of study.
Conclusion
Analyzing regression, correspondence, and experimental data requires a systematic approach that begins with understanding the research question and data, choosing the right statistical method, conducting the analysis, and finally, reporting the findings. By following the strategies outlined in this blog, you can approach these complex statistical assignments with confidence, ensuring accurate and meaningful results. Whether you are working with continuous variables, categorical data, or experimental outcomes, the principles and techniques discussed here will help you navigate the challenges of statistical analysis and achieve success in your assignments.
The key to success in statistical assignments lies in careful planning, thoughtful analysis, and clear communication. By taking the time to fully understand the research question and data, selecting the appropriate statistical methods, and accurately interpreting and reporting the results, you can produce high-quality work that meets the demands of even the most challenging assignments. As you continue to develop your statistical skills, remember that each assignment is an opportunity to deepen your understanding and refine your analytical techniques, paving the way for future success in the field of statistics.