Claim Your Offer
Unlock an exclusive deal at www.statisticsassignmenthelp.com with our Spring Semester Offer! Get 10% off on all statistics assignments and enjoy expert assistance at an affordable price. Our skilled team is here to provide top-quality solutions, ensuring you excel in your statistics assignments without breaking the bank. Use Offer Code: SPRINGSAH10 at checkout and grab this limited-time discount. Don’t miss the chance to save while securing the best help for your statistics assignments. Order now and make this semester a success!
We Accept
- Understanding Probability Distributions and Their Properties
- Constructing the Likelihood Function
- Identifying Sufficient Statistics
- Finding the Maximum Likelihood Estimator (MLE)
- Evaluating the Minimum Variance Bound
- Constructing Confidence Intervals
- Applying the Process to Different Distributions
- Common Pitfalls and How to Avoid Them
- Conclusion
Statistical analysis often involves working with probability distributions and finding ways to estimate unknown parameters based on sample data. One of the most commonly used techniques for this is Maximum Likelihood Estimation (MLE). This method provides a framework for estimating the parameters of a probability distribution by maximizing the likelihood function, which measures how likely it is to observe the given sample data for various parameter values. In this blog, we’ll explore how students can approach assignments involving MLE and probability distributions, offering strategies to effectively solve their probability distributions assignment and similar types of problems.
Understanding Probability Distributions and Their Properties
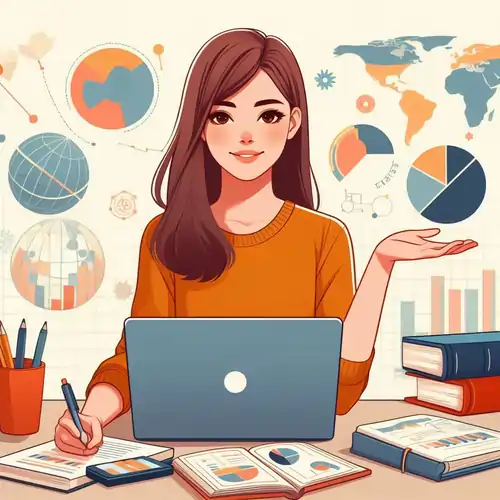
Before diving into MLE, it's crucial to have a firm grasp of the probability distribution you're dealing with. Probability distributions are mathematical functions that describe the likelihood of different outcomes in an experiment or a process. Each distribution has its own unique properties, including its mean, variance, and the specific form of its probability function, which could be either a probability mass function (pmf) for discrete distributions or a probability density function (pdf) for continuous distributions.
When tackling an assignment, the first step is to carefully read the problem to identify the distribution type and its parameters. For example, a problem might define a distribution using a parameter θ, which could represent anything from the probability of success in a Bernoulli distribution to the rate parameter in an exponential distribution. Understanding these parameters and their roles in the distribution is essential for further calculations and inferences.
Constructing the Likelihood Function
Once you’ve identified the distribution, the next step in an MLE-based assignment is to construct the likelihood function. The likelihood function represents the probability of the observed data as a function of the unknown parameter(s). It is essentially the product of the individual probabilities (or probability densities) for each observed data point in your sample.
Constructing the likelihood function correctly is key, as it lays the foundation for all subsequent steps. For instance, if you are working with a geometric distribution, your likelihood function will involve the product of the probabilities associated with each observation in your sample, with each probability depending on the parameter θ\thetaθ that you are trying to estimate.
This step might seem straightforward, but it requires careful attention to detail. Mistakes in the likelihood function will lead to incorrect estimations later on. Therefore, take your time to ensure that each step in the construction of the likelihood function is accurate.
Identifying Sufficient Statistics
In statistics, a sufficient statistic is a function of the data that encapsulates all the information needed to estimate a parameter. This concept is particularly useful in MLE, as identifying a sufficient statistic can simplify the process of parameter estimation.
To demonstrate that a statistic is sufficient, you can use the factorization theorem. This theorem states that a statistic is sufficient for a parameter if the likelihood function can be factored into a product, where one factor depends on the data only through the statistic and the other factor depends only on the parameter. This essentially means that once you have the sufficient statistic, no other function of the data provides any additional information about the parameter.
Finding the Maximum Likelihood Estimator (MLE)
The central task in an MLE problem is to find the Maximum Likelihood Estimator (MLE) for the parameter. The MLE is the value of the parameter that maximizes the likelihood function, meaning it makes the observed data most probable.
To find the MLE, you generally follow these steps:
- Differentiate the likelihood function (or the log-likelihood function, which is often simpler to work with) with respect to the parameter θ.
- Set the derivative equal to zero to find the critical points. These points are potential candidates for the MLE.
- Solve for θ to obtain the value that maximizes the likelihood function.
Finding the MLE can be mathematically challenging, especially in cases where the likelihood function is complex. However, the process often simplifies when you work with the log-likelihood instead of the likelihood itself, as logarithms convert products into sums, making differentiation more straightforward.
Evaluating the Minimum Variance Bound
After finding the MLE, the next step is often to evaluate how good this estimator is. One way to do this is by calculating the Cramér-Rao lower bound, which provides a theoretical minimum variance for any unbiased estimator of a parameter.
To calculate the Cramér-Rao bound:
- Compute the Fisher Information: This involves finding the expected value of the second derivative of the log-likelihood function with respect to the parameter θ. The Fisher Information measures the amount of information that an observable random variable carries about the parameter.
- Use the Cramér-Rao inequality: The variance of any unbiased estimator is at least as large as the inverse of the Fisher Information. If your MLE achieves this bound, it’s considered an efficient estimator.
Understanding the concept of the minimum variance bound is important because it helps you assess the efficiency of your estimator. An efficient estimator not only provides an unbiased estimate of the parameter but also does so with the lowest possible variance, making it the best possible estimator among all unbiased estimators.
Constructing Confidence Intervals
In addition to point estimates, statistical analysis often requires you to provide interval estimates, known as confidence intervals. A confidence interval gives a range of values within which the true parameter is likely to fall, with a certain level of confidence (e.g., 95%).
To construct a confidence interval for the parameter θ using the MLE:
- Determine the standard error of the MLE, which is related to the inverse of the Fisher Information.
- Use the asymptotic normality of the MLE in large samples to construct the interval. For large samples, the distribution of the MLE can be approximated by a normal distribution centered at the true parameter value with a variance equal to the inverse of the Fisher Information.
- Calculate the interval: For a 95% confidence level, the interval is typically constructed as is the critical value from the standard normal distribution corresponding to your desired confidence level.
Confidence intervals are particularly useful because they provide a range of plausible values for the parameter rather than a single point estimate. This interval accounts for the uncertainty inherent in using a sample to estimate a population parameter.
Applying the Process to Different Distributions
The beauty of the MLE framework is its broad applicability. Whether you are dealing with a discrete distribution like the geometric or Poisson distribution, or a continuous one like the exponential or normal distribution, the steps remain largely the same. The specific details—such as the form of the likelihood function or the Fisher Information—will change depending on the distribution, but the overarching principles do not.
Common Pitfalls and How to Avoid Them
Assignments involving MLE and probability distributions are often fraught with potential pitfalls. Being aware of these can help you avoid common mistakes:
- Incorrectly Constructing the Likelihood Function: A small mistake in the likelihood function can lead to incorrect MLEs. Double-check your work to ensure that the function correctly reflects the distribution and the observed data.
- Misidentifying Sufficient Statistics: Not all statistics are sufficient, and incorrectly identifying one can lead to inefficient or biased estimators. Make sure you use the factorization theorem correctly.
- Ignoring Boundary Conditions: When solving for the MLE, boundary conditions are sometimes overlooked, especially if the parameter is restricted to a certain range. Always consider the domain of the parameter when solving the likelihood equation.
- Overcomplicating Confidence Interval Calculations: Confidence intervals can often be simplified by using standard tables or software tools. Don’t get bogged down in overly complex calculations when a simpler solution is available.
Conclusion
Assignments involving Maximum Likelihood Estimation (MLE) and probability distributions require a methodical approach and a deep understanding of statistical concepts. By following the steps outlined in this blog—understanding the distribution, constructing the likelihood function, identifying sufficient statistics, finding the MLE, evaluating the minimum variance bound, and constructing confidence intervals—students can tackle these assignments with confidence. For those seeking assistance with statistics assignment, these strategies are particularly beneficial.
While the specific details may vary depending on the distribution, the fundamental principles remain the same. With practice, the process of deriving and applying MLEs becomes more intuitive, allowing students to handle even the most complex assignments with ease. Whether you're dealing with a geometric distribution, an exponential distribution, or any other type of probability distribution, the strategies discussed here will serve as a valuable guide in your statistical toolkit.